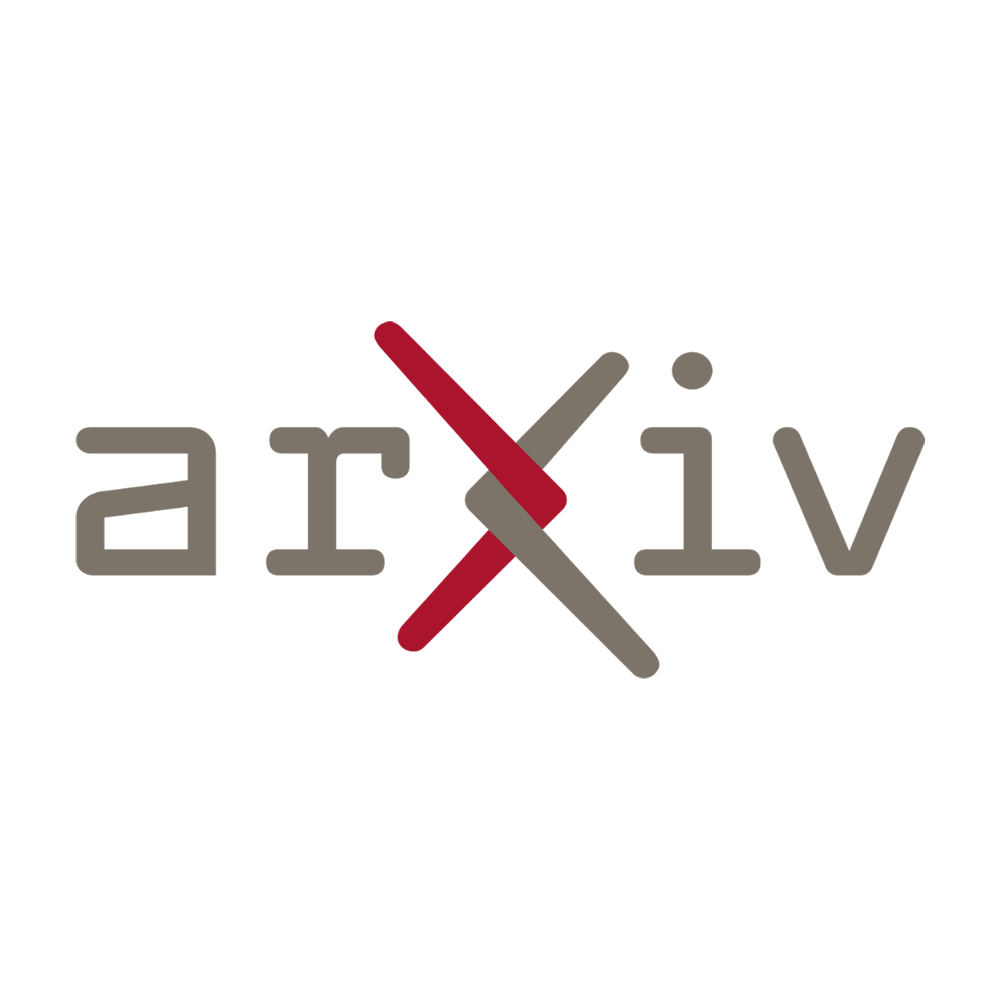
the field of deep learning continues to evolve, object detection has emerged as a crucial technology, finding extensive applications in autonomous driving. With the ability to analyze visual data, object detection plays a pivotal role in enhancing the safety and efficiency of self-driving vehicles. This article explores the significant advancements in deep learning that have fueled the rise of object detection in autonomous driving, highlighting its importance and potential impact on the future of transportation. From improving pedestrian detection to enabling real-time decision-making, object detection is revolutionizing the way we navigate the roads, ultimately paving the way for a safer and more autonomous future.
Spurred by consistent advances and innovation in deep learning, object detection applications have become prevalent, particularly in autonomous driving that leverages various visual data. As vehicles are becoming increasingly autonomous, the need for accurate and robust object detection algorithms has become crucial for ensuring safety and efficiency on roads.
The Challenge of Object Detection
Object detection involves identifying and localizing multiple objects within an image or video stream. Traditional methods relied on handcrafted features and heuristics, making them less reliable and often limited in their ability to handle complex scenarios. However, with the rise of deep learning, object detection has witnessed significant improvements.
One of the key challenges in object detection is achieving real-time performance without compromising accuracy. Deep learning algorithms that excel in accuracy tend to be computationally expensive. This poses a challenge in resource-constrained environments such as embedded systems and autonomous vehicles, where real-time processing is critical.
Innovative Solutions for Real-Time Object Detection
To address the challenge of real-time object detection, researchers and engineers are exploring several innovative solutions:
- Efficient Neural Architectures: Recent advancements have focused on designing efficient neural network architectures that strike a balance between accuracy and computation performance. Models like YOLO (You Only Look Once) and SSD (Single Shot Multibox Detector) have gained popularity for their ability to achieve near real-time performance while maintaining high accuracy.
- Hardware Acceleration: Another approach to achieving real-time object detection is through hardware acceleration. Specialized hardware, such as Graphics Processing Units (GPUs) or dedicated deep learning accelerators (e.g., NVIDIA’s Tensor Cores), can significantly speed up the inference process. These accelerators allow for parallel computations and optimized matrix operations, resulting in faster object detection.
- Knowledge Distillation: Knowledge distillation involves training a lightweight model to mimic the predictions of a larger, more accurate model. By transferring the knowledge from the accurate model to the lightweight model, real-time performance can be achieved without sacrificing accuracy. This technique has gained attention due to its ability to balance the trade-off between speed and accuracy.
The Future of Object Detection
As the field of deep learning continues to evolve, the future of object detection holds promising possibilities. Some areas of exploration include:
- Incremental Learning: Traditional object detection models are trained on large datasets from scratch. However, incremental learning aims to update the model using smaller, incremental datasets. This approach allows for continuous improvement and adaptability to new environments without discarding prior knowledge.
- Domain Adaptation: Object detection algorithms typically perform best when trained and tested on data from the same distribution. However, in real-world scenarios, there may be a significant domain shift between training and testing data. Domain adaptation techniques aim to bridge this gap by adapting the model to perform well in different domains, enhancing its robustness and generalization capabilities.
- Meta-Learning: Meta-learning focuses on developing algorithms that can learn from few-shot learning scenarios. By training models on a variety of tasks and datasets, they can quickly adapt to new tasks with limited labeled data. Meta-learning holds the potential to make object detection more efficient and effective in scenarios where labeled data is scarce.
Object detection algorithms have come a long way with the advent of deep learning. However, the pursuit of real-time performance without sacrificing accuracy remains a challenge. Through innovative techniques such as efficient neural architectures, hardware acceleration, and knowledge distillation, researchers and engineers are paving the way towards real-time object detection. The future of the field holds exciting possibilities with incremental learning, domain adaptation, and meta-learning opening new avenues for robust and efficient object detection.
an expert commentator, I would like to add my analysis and insights into the current state of object detection applications and their implications for autonomous driving.
Deep learning has revolutionized the field of computer vision, enabling significant advancements in object detection algorithms. This has paved the way for the development of various applications, with autonomous driving being one of the most prominent areas of implementation.
Autonomous vehicles heavily rely on visual data to perceive and interpret their surroundings. Object detection plays a crucial role in this process by accurately identifying and localizing objects such as pedestrians, other vehicles, traffic signs, and obstacles. Deep learning techniques, particularly convolutional neural networks (CNNs), have proven to be highly effective in achieving this task with remarkable accuracy and speed.
The integration of object detection applications in autonomous driving has brought about significant improvements in safety, efficiency, and overall performance. By accurately identifying objects in real-time, autonomous vehicles can make informed decisions and take appropriate actions to navigate through complex traffic scenarios. This not only enhances passenger safety but also contributes to the reduction of accidents caused by human error.
However, there are still challenges that need to be addressed to further enhance object detection in autonomous driving. One such challenge is the ability to detect and classify objects accurately under various environmental conditions, such as adverse weather (heavy rain, fog) or low light conditions. Robustness in object detection algorithms is crucial to ensure reliable performance in all scenarios.
Another aspect that requires attention is the real-time processing of visual data. Autonomous vehicles operate in dynamic environments where decisions need to be made quickly. Therefore, object detection algorithms should be capable of processing large amounts of data in real-time, ensuring minimal latency between perception and action.
Furthermore, as autonomous driving technology evolves, there is a growing need for more sophisticated object detection techniques that can handle complex traffic situations. This includes detecting subtle driver behaviors, understanding road markings and lane changes, and predicting the intentions of other vehicles. Advancements in deep learning, combined with the integration of other sensor modalities like LiDAR and radar, will play a crucial role in addressing these challenges.
Looking ahead, the future of object detection in autonomous driving seems promising. Continued advances in deep learning will likely lead to even more accurate and efficient algorithms. Additionally, the integration of edge computing and distributed processing will enable faster and more efficient object detection systems. Furthermore, the development of hybrid approaches that combine the strengths of different object detection methods, such as CNNs and graph-based algorithms, could lead to significant improvements in performance and robustness.
In conclusion, deep learning-powered object detection applications have revolutionized autonomous driving, improving safety and efficiency. However, there are still challenges to be overcome, such as robustness in adverse conditions and real-time processing. The future holds great promise with further advancements in deep learning techniques and the integration of multiple sensor modalities, paving the way for even more sophisticated object detection systems in autonomous vehicles.
Read the original article