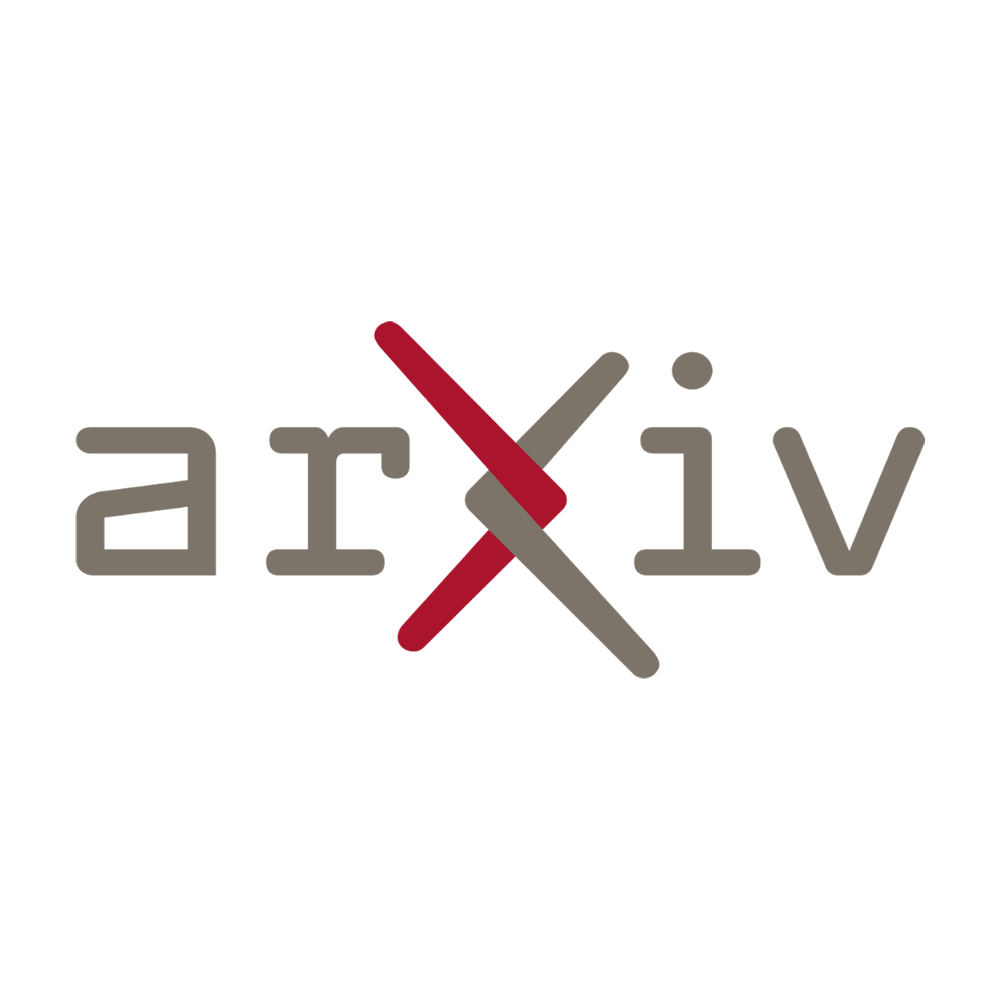
Learning (DL). NWP relies on complex mathematical models and historical data, while DL uses artificial intelligence to analyze vast amounts of data. This article explores the strengths and limitations of both paradigms and discusses the potential for combining them to improve weather forecasting accuracy. By understanding the unique advantages of NWP and DL, as well as their respective weaknesses, researchers aim to develop a hybrid approach that maximizes the benefits of both methods. Ultimately, the goal is to enhance our ability to predict weather patterns and provide more reliable information for a range of industries and activities that rely on accurate forecasts.
The field of weather forecasting plays a pivotal role in our daily lives, influencing everything from our travel plans to agricultural practices. Accurate weather predictions are crucial for societies to efficiently plan and adapt to varying atmospheric conditions. While traditional numerical weather prediction (NWP) methods have long served as the foundation for forecasting, recent advancements in deep learning have introduced a new paradigm that has the potential to revolutionize this field.
Numerical Weather Prediction (NWP)
NWP is a tried-and-true technique that relies on mathematical models and computational algorithms to simulate the atmosphere’s behavior. By assimilating vast amounts of observational data, it produces forecasts based on complex equations that dictate atmospheric processes. NWP has greatly improved meteorological predictions over time and remains widely used by meteorologists worldwide.
However, NWP does have its limitations. The modeling assumptions and simplifications inherent in the technique can lead to errors, especially in regions with complex terrain or sparse observational data. Additionally, NWP requires substantial computing power and expertise to operate effectively, making it less accessible to smaller organizations or developing countries.
Deep Learning in Weather Forecasting
Deep learning is a subset of machine learning that utilizes artificial neural networks, mimicking the human brain’s structure and functioning. This approach has gained traction across various domains due to its ability to process vast amounts of data, recognize patterns, and make accurate predictions.
The application of deep learning in weather forecasting involves training neural networks on historical weather data and observations to learn complex relationships. These trained models can then generate forecasts based on current or future weather conditions. Unlike NWP, deep learning models rely less on assumptions and can capture intricate atmospheric dynamics that traditional methods may overlook.
The Potential of Deep Learning for Weather Forecasting
Deep learning has the potential to address some of the limitations of traditional NWP methods. Its ability to harness large datasets and extract meaningful patterns can help improve forecasts in regions where NWP struggles. By incorporating various data sources such as satellite imagery, social media feeds, and sensor networks, deep learning models can enhance their predictive capabilities.
Additionally, deep learning can potentially democratize weather forecasting. Its scalability and relatively lower computational requirements make it accessible to smaller organizations or even individual enthusiasts. This democratization can lead to a more diverse range of forecast models and improved localized predictions tailored to specific needs.
Challenges and Considerations
While deep learning holds immense promise, it also faces several challenges. The need for extensive, high-quality data for training models poses a significant hurdle. Obtaining and curating such datasets may be complex and time-consuming, especially in regions with limited resources. Moreover, deep learning models can be computationally expensive during the training phase, demanding substantial computing power and resources.
Additionally, interpretability remains a concern with deep learning models. Unlike NWP, which provides clear insights into the underlying physical processes, deep learning methods often work as “black boxes,” making it difficult to understand why a particular forecast was made. Developing methods for interpreting and explaining the decisions made by these models is an ongoing area of research.
The Way Forward: Hybrid Approaches
The future of weather forecasting lies in hybrid approaches that combine the strengths of both traditional NWP and deep learning. By merging the physical understanding of NWP models with the pattern recognition abilities of deep learning algorithms, we can create more accurate and interpretable forecasts.
This hybrid approach can, for example, use NWP models to provide initial conditions for deep learning models or leverage deep learning architectures to improve specific aspects of NWP predictions. Collaborative efforts between meteorologists and data scientists are crucial to ensure the successful integration of these approaches.
Conclusion
Weather forecasting is continuously evolving, driven by advancements in both traditional methods and emerging technologies. While deep learning holds immense potential, it is essential to strike a balance between harnessing its advantages and addressing its limitations. By embracing hybrid approaches and fostering interdisciplinary collaborations, we can unlock the full potential of weather forecasting and effectively mitigate the impact of weather-related events on our lives.
Learning (DL). NWP has been the traditional method used by meteorologists for decades, relying on mathematical equations to simulate and predict atmospheric behavior. On the other hand, DL is a more recent approach that utilizes artificial neural networks to learn patterns and make predictions based on large amounts of data.
Both paradigms have their strengths and limitations. NWP excels in capturing the physics and dynamics of the atmosphere, allowing for accurate predictions of large-scale weather patterns. However, it struggles with resolving small-scale phenomena such as convective storms or local temperature variations. DL, on the other hand, is adept at capturing complex nonlinear relationships in the data, enabling it to make more accurate predictions for local and short-term weather conditions.
As technology continues to advance, we can expect to see a blending of these two paradigms in the future. One possible direction is the integration of DL into NWP models, allowing for a more comprehensive and accurate representation of atmospheric processes at all scales. This hybrid approach would combine the strengths of both paradigms, leading to improved forecasting capabilities.
Another area of development lies in the utilization of big data and machine learning algorithms to enhance weather forecasting models. With the increasing availability of weather data from various sources such as satellites, radars, and weather stations, there is a wealth of information that can be harnessed to improve predictions. By training DL models on vast amounts of historical weather data, we can uncover hidden patterns and relationships that were previously difficult to detect.
Furthermore, advancements in computing power and data processing capabilities will play a crucial role in the evolution of weather forecasting. High-performance computing systems will enable meteorologists to run more complex models with higher resolutions, resulting in finer details and improved accuracy in forecasts. Additionally, real-time data assimilation techniques will become more sophisticated, allowing forecast models to continuously update and adjust based on new observations.
In the future, we can also expect weather forecasting to become more personalized and localized. With the proliferation of smartphones and wearable devices, individuals will have access to real-time weather information tailored to their specific location and preferences. This hyper-localized forecasting will greatly benefit various sectors such as agriculture, transportation, and outdoor activities, enabling better planning and decision-making.
Overall, the future of weather forecasting holds great promise. The combination of NWP and DL, along with advancements in data analysis and computing power, will lead to more accurate and timely predictions. As our understanding of atmospheric processes deepens and technology continues to advance, we can expect weather forecasts to become an indispensable tool for individuals and industries alike.
Read the original article