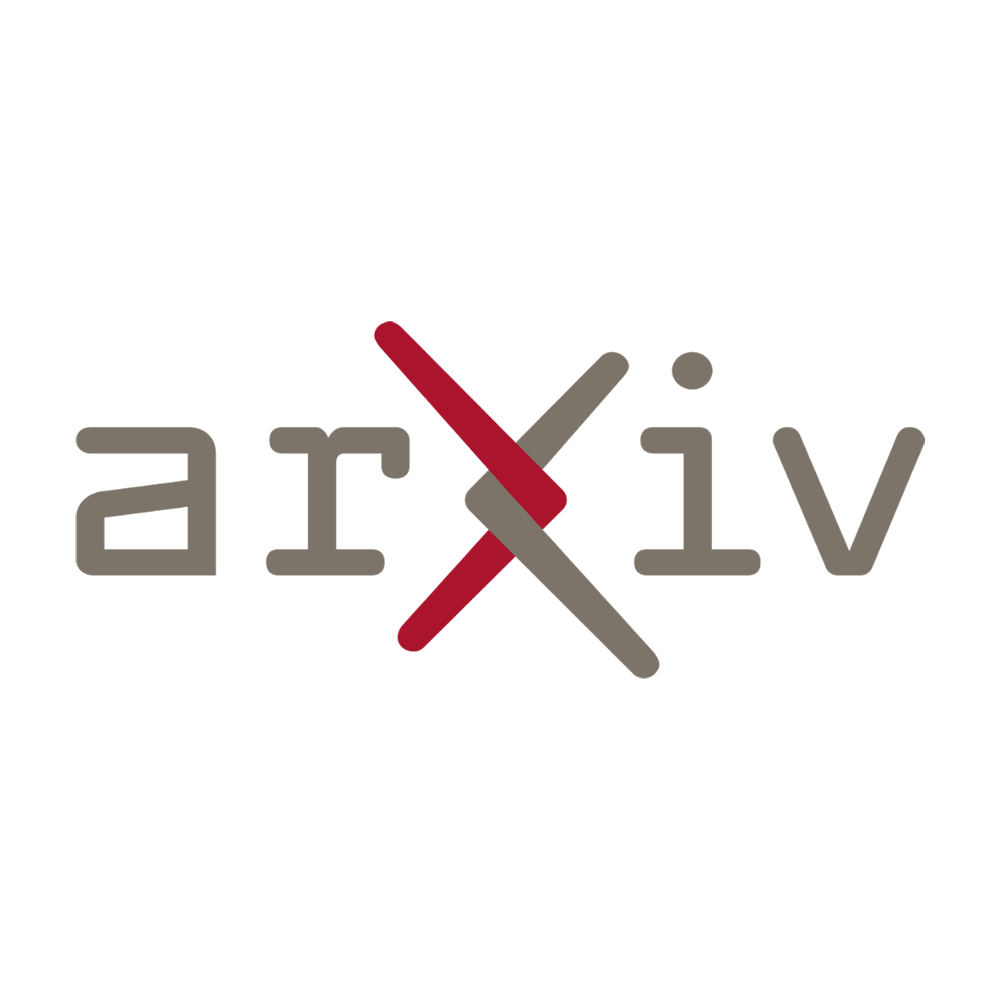
In this article, we delve into the groundbreaking research conducted by the MLCommons Science Working Group on the cloud masking benchmark. As part of the MLCommons consortium, renowned for its dedication to advancing scientific knowledge, this study sheds light on the intricate world of cloud masking and its crucial role in various fields. By exploring the methodology, findings, and implications of this research, readers will gain a comprehensive understanding of the significance and potential applications of cloud masking in scientific endeavors.
Innovation in Cloud Masking: Unleashing the Potential of MLCommons
Introduction
In the world of scientific advancements, MLCommons stands out as a consortium that continuously pushes the boundaries of machine learning. MLCommons Science Working Group has been diligently working on the cloud masking benchmark to enhance accurate cloud detection in satellite imagery. This article aims to explore the underlying themes and concepts of the provided material while proposing innovative solutions and ideas to further expand the capabilities of cloud masking.
The Importance of Cloud Masking
Cloud detection plays a vital role in various fields, including weather forecasting, agriculture, disaster management, and environmental monitoring. Accurate cloud masking allows scientists, researchers, and policymakers to make informed decisions based on reliable data. It helps in identifying cloud-free areas for detailed analysis and facilitates efficient resource allocation for different applications.
The Challenge at Hand
The existing cloud masking techniques have made significant progress in recent years. However, challenges remain, such as:
- Computational Efficiency: Cloud masking algorithms need to perform efficiently on large-scale imagery datasets to enable real-time or near-real-time applications.
- Robustness: The algorithms should be robust enough to handle complex atmospheric conditions, varying illumination, and diverse satellite sensors.
- Optimizing Accuracy: Enhancing the accuracy of cloud detection by reducing false positives and false negatives remains a critical area for improvement.
Innovative Solutions
To overcome the challenges discussed above, MLCommons can consider exploring the following innovative solutions:
- Data Augmentation Techniques: By enhancing the training dataset through data augmentation techniques, such as rotation, scaling, and image synthesis, MLCommons can improve the robustness of cloud masking algorithms. This can help the models learn to handle diverse atmospheric conditions and complex lighting scenarios.
- Parallelization and Distributed Computing: To address the computational efficiency challenge, MLCommons can leverage parallelization techniques and distributed computing frameworks. This would accelerate the cloud masking process, enabling real-time or near-real-time applications.
- Transfer Learning: MLCommons can explore the potential of transfer learning to optimize accuracy. By pre-training models on a large dataset from different satellite sensors and fine-tuning them on specific satellite imagery datasets, the algorithms can adapt better to different sensor characteristics and achieve higher accuracy.
Conclusion
MLCommons Science Working Group’s focus on improving cloud masking techniques is commendable. By addressing the challenges faced in cloud detection and leveraging innovative solutions, MLCommons can enable more accurate and efficient analyses across various domains. Embracing data augmentation, parallelization techniques, and transfer learning will unlock the true potential of cloud masking and support decision-making processes that rely on reliable satellite imagery data.
“The future of cloud masking lies in constantly pushing the boundaries of machine learning and harnessing its power to unlock the full potential of satellite imagery.” – MLCommons
MLCommons is an influential consortium that plays a crucial role in the development and maintenance of scientific benchmarks in machine learning. In this particular paper, the focus is on the cloud masking benchmark, which is a significant task in remote sensing and satellite imagery analysis.
Cloud masking refers to the process of identifying and removing cloud cover from satellite images to obtain clear and usable data. This task is essential for various applications, including weather forecasting, land cover classification, and environmental monitoring. Accurate cloud masking algorithms are vital for ensuring the reliability and quality of these applications.
The MLCommons Science Working Group has taken up the challenge of developing a benchmark to evaluate the performance of cloud masking algorithms. This is an important step in advancing the field, as it allows researchers and developers to compare different algorithms objectively and identify areas for improvement.
The development of a benchmark involves creating a standardized dataset, defining evaluation metrics, and establishing a common set of rules for testing. MLCommons has a track record of successfully developing benchmarks for various machine learning tasks, and their involvement in the cloud masking benchmark instills confidence in its credibility and potential impact.
Moving forward, it is expected that the MLCommons Science Working Group will continue to refine and expand the cloud masking benchmark. This could involve incorporating more diverse datasets, including images with varying spatial resolutions, atmospheric conditions, and sensor characteristics. Additionally, the evaluation metrics may be further refined to capture nuances in algorithm performance, such as distinguishing between different types of cloud cover.
The benchmark could also serve as a catalyst for innovation in cloud masking algorithms. By providing a standardized platform for evaluation, researchers and developers can directly compare their methods against state-of-the-art approaches. This healthy competition is likely to drive advancements in algorithmic techniques and lead to more accurate and efficient cloud masking solutions.
Furthermore, MLCommons has a strong community of experts and industry partners who collaborate on benchmark development. This collaborative effort ensures that the benchmark remains relevant and up-to-date with the latest advancements in the field. It also fosters knowledge sharing and encourages the adoption of best practices across the community.
In conclusion, the MLCommons Science Working Group’s efforts in developing a cloud masking benchmark are commendable and have the potential to significantly impact the field of remote sensing and satellite imagery analysis. The benchmark will not only provide a standardized platform for evaluating algorithm performance but also foster innovation and collaboration among researchers and developers. As the benchmark evolves, it is expected to contribute to the development of more accurate and efficient cloud masking algorithms, ultimately benefiting a wide range of applications that rely on satellite imagery data.
Read the original article