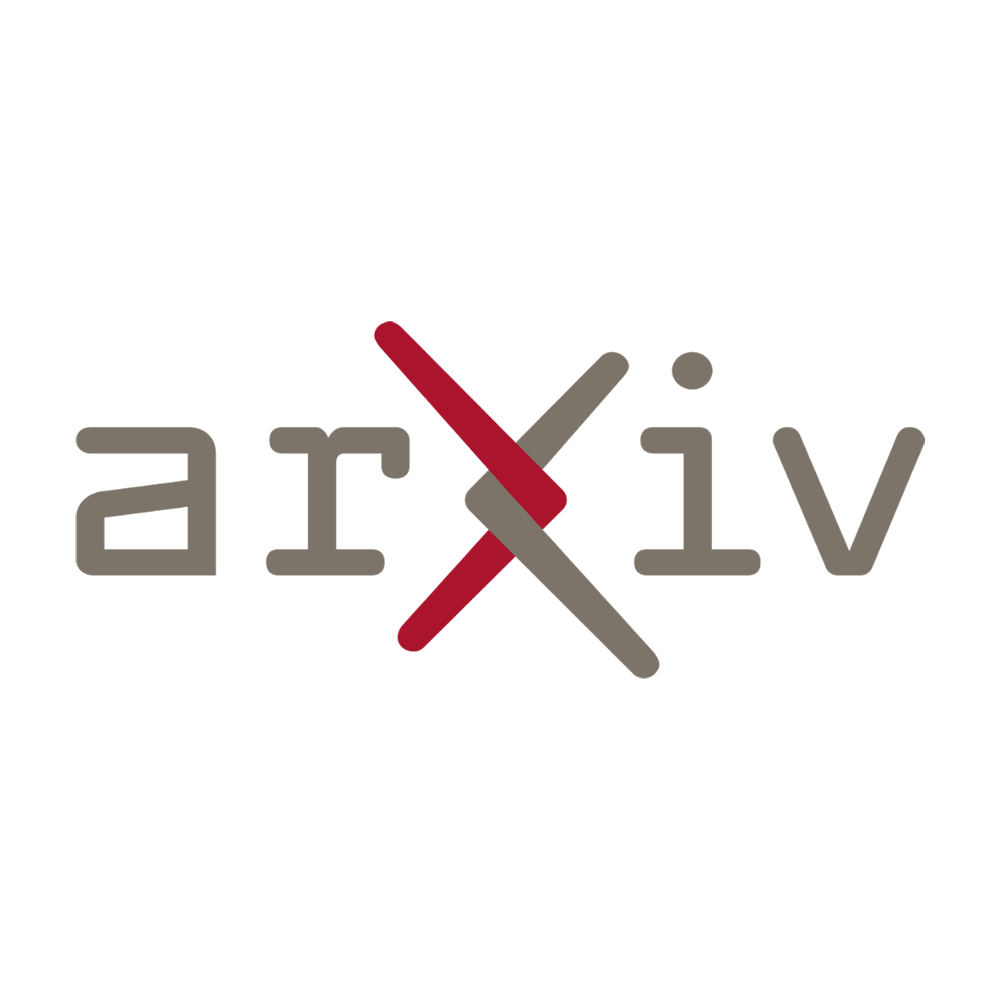
the complex interactions between various atmospheric factors. In recent years, researchers have turned to artificial intelligence (AI) and machine learning (ML) techniques to improve precipitation prediction accuracy. These advanced technologies have shown promising results in capturing the intricate relationships between meteorological variables and enhancing forecast models. This article delves into the cutting-edge applications of AI and ML in precipitation prediction, exploring their potential to revolutionize the way we anticipate and manage rainfall patterns. From enhancing agricultural planning to mitigating the impacts of extreme weather events, the integration of AI and ML in precipitation prediction offers a glimpse into a more resilient and sustainable future.
Precipitation prediction plays a crucial role in modern agriculture and industry. However, it poses significant challenges due to the diverse patterns and dynamics in time and space, as well as the increasing frequency of extreme weather events. Traditional methods of precipitation prediction have limitations in accuracy and reliability, often resulting in unexpected outcomes and significant economic losses. To address these challenges, innovative solutions are needed to improve the accuracy and reliability of precipitation prediction.
Harnessing Artificial Intelligence
One innovative solution is to harness the power of Artificial Intelligence (AI) in precipitation prediction. AI algorithms can analyze vast amounts of data, including historical weather records, satellite imagery, and atmospheric conditions, to identify patterns and correlations that human experts may overlook. By training AI models on diverse datasets, they can learn to accurately predict precipitation patterns in different regions, seasons, and even extreme weather events.
Moreover, AI models can continuously learn and adapt as they receive new data, making them more robust and reliable over time. This ability to adapt to changing conditions is especially crucial in precipitation prediction, where weather patterns can vary significantly in different years or even within a single season.
Utilizing Internet of Things (IoT) Devices
An additional innovative solution lies in utilizing Internet of Things (IoT) devices for real-time data collection. IoT devices like weather sensors, drones, and ground-level monitoring stations can provide localized and high-resolution data on temperature, humidity, wind speed, and other atmospheric parameters. When combined with AI algorithms, this real-time data can significantly improve the accuracy of precipitation prediction.
By deploying IoT devices across a wide geographical area, a comprehensive network of data collection can be established. This network ensures that data from remote and underserved regions is also included in the prediction models. The spatial diversity of data helps capture localized variations in weather patterns, leading to more accurate and reliable precipitation predictions on a regional scale.
Integration of Big Data Analytics
Big Data analytics approaches can also play a key role in improving precipitation prediction. By integrating diverse datasets from various sources, including weather records, satellite imagery, oceanic conditions, and historical climate patterns, we can gain deeper insights into the complex dynamics of precipitation.
Through data mining and machine learning algorithms, Big Data analytics can identify hidden patterns and relationships between seemingly unrelated variables. For example, it can reveal how oceanic temperature anomalies influence rainfall distribution or how atmospheric pressure systems impact the likelihood of extreme precipitation events.
Collaborative Efforts and Open Data Sharing
Lastly, collaborative efforts and open data sharing are essential for advancing precipitation prediction. In a rapidly changing climate, it is crucial to establish a global network of scientists, researchers, and meteorological agencies to share data, techniques, and insights.
By fostering an environment of cooperation and knowledge exchange, we can leverage collective expertise to develop innovative solutions and address the challenges of precipitation prediction more effectively. Open data platforms and standardized data formats can facilitate easy access and sharing of weather-related information globally, enabling interdisciplinary research and collaboration across borders.
In conclusion, the future of precipitation prediction lies in harnessing innovative technologies such as Artificial Intelligence, Internet of Things devices, and Big Data analytics. By combining these approaches with collaborative efforts and open data sharing, we can overcome the challenges posed by diverse weather patterns and extreme events. The implementation of these solutions holds the potential to revolutionize agriculture, industry, and disaster preparedness, ultimately building resilience in the face of the ever-changing climate.
the complex interactions between various atmospheric and environmental factors. Accurate precipitation prediction is essential for farmers, water resource management, and industries that rely on weather conditions, such as renewable energy generation and construction.
One of the primary challenges in precipitation prediction is the spatial variability of rainfall patterns. Rainfall can vary significantly even within a small geographical area, making it difficult to predict with high accuracy. This variability is influenced by factors such as topography, local climate, land cover, and atmospheric conditions. Therefore, understanding these local-scale processes and their interactions is crucial for improving precipitation prediction models.
Another challenge is the temporal dynamics of precipitation. Rainfall patterns can exhibit seasonality, diurnal variations, and interannual variability. For example, some regions experience monsoon seasons with heavy rainfall, while others have more evenly distributed rainfall throughout the year. Predicting these temporal patterns accurately requires comprehensive knowledge of global climate dynamics, ocean-atmosphere interactions, and other large-scale atmospheric phenomena like El Niño and La Niña.
Furthermore, the interactions between different atmospheric and environmental factors add complexity to precipitation prediction. Factors such as temperature, humidity, wind patterns, and cloud dynamics all influence rainfall. Additionally, feedback mechanisms between the land surface and the atmosphere can further complicate the prediction process. For instance, vegetation cover and soil moisture content can affect local weather conditions and subsequently impact rainfall patterns.
To overcome these challenges, advancements in technology and data collection have been instrumental. Weather radars, satellites, and ground-based weather stations provide valuable data for precipitation prediction models. The integration of these data sources with advanced numerical weather prediction models has significantly improved forecast accuracy.
However, there is still room for improvement. Machine learning techniques and artificial intelligence have shown promise in enhancing precipitation prediction by incorporating large amounts of data and identifying complex patterns. By leveraging these technologies, we can potentially develop more robust models that can capture the intricate relationships between different variables and improve forecast accuracy.
Looking ahead, the future of precipitation prediction lies in the integration of various data sources and the advancement of modeling techniques. The development of high-resolution models that can capture small-scale processes, coupled with improved understanding of atmospheric dynamics, will be crucial. Additionally, collaborations between meteorologists, climatologists, hydrologists, and data scientists will be essential to tackle the interdisciplinary nature of precipitation prediction.
Overall, while precipitation prediction poses significant challenges, advancements in technology and modeling techniques provide hope for improved accuracy. Continued research and innovation in this field will not only benefit agriculture and industry but also contribute to better understanding and management of our planet’s water resources.
Read the original article