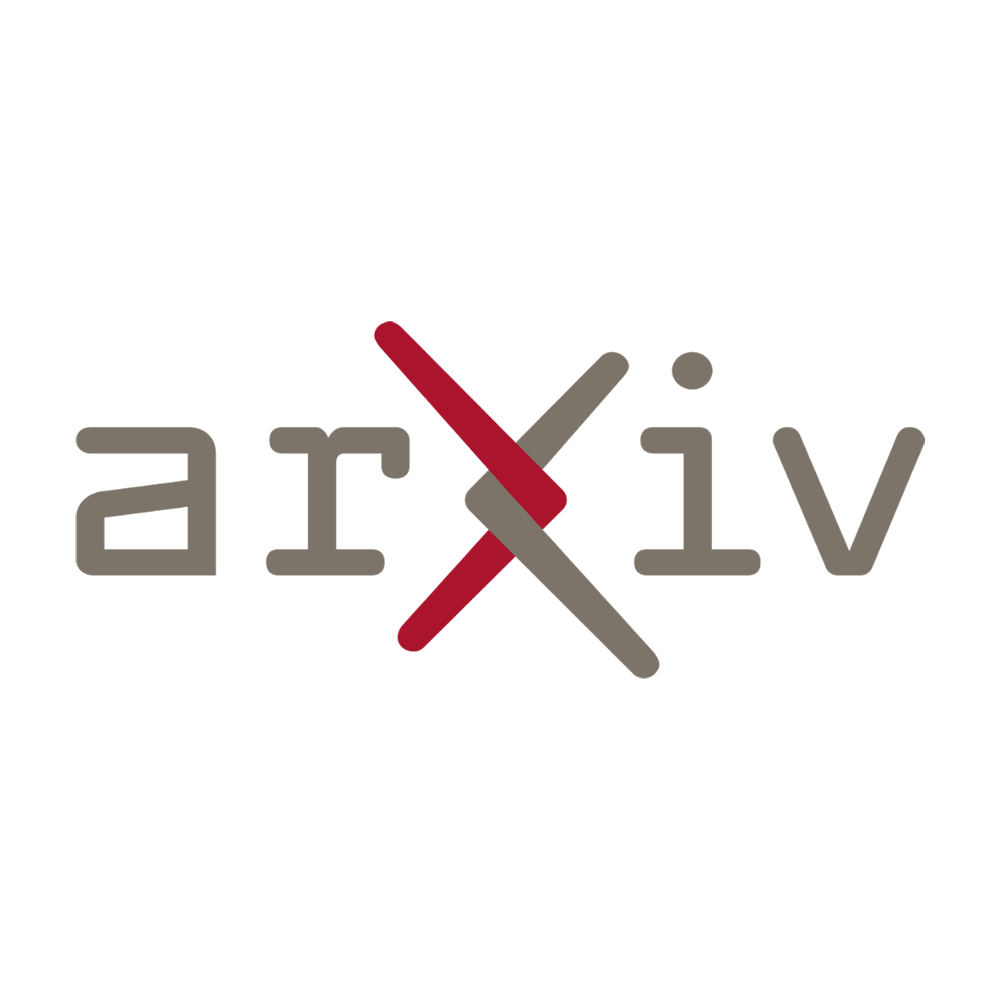
in the form of captions, which limits the models’ ability to understand visual information beyond what is explicitly described. To address this limitation, researchers propose a new method called Conceptual Captions, which leverages a vast dataset of images and their corresponding captions to enhance the visual comprehension of these models. By training on Conceptual Captions, vision-language models can learn to associate images with a broader range of concepts, leading to improved performance in tasks like image retrieval. This article explores the potential of Conceptual Captions in enhancing the visual understanding capabilities of vision-language models and the implications it holds for various applications.
As technology continues to advance, the field of computer vision has made great strides in understanding and analyzing images. Vision-Language Models (VLMs) have emerged as a powerful tool in this domain, enabling tasks such as image retrieval with remarkable accuracy. However, the pre-training process for VLMs heavily relies on large-scale image-text datasets, which pose some interesting challenges.
Understanding Pre-Training
Pre-training VLMs involves exposing them to vast amounts of image-text pairs, allowing the model to learn from the relationships between these modalities. The model is trained to predict a masked word or phrase in a given sentence, given both the surrounding text and the associated image. Through this process, the VLM learns to associate textual descriptions with visual content, ultimately aiding in tasks like image retrieval.
One fundamental limitation of current pre-training methods is the selection of images used during training. In most cases, the images are selected explicitly for their textual relevance. As a result, the pre-trained VLM may become biased towards capturing language-specific features instead of generalizable visual features.
Towards Generalizable Pre-Training
To address this limitation, we propose an innovative approach to VLM pre-training that focuses on enhancing the model’s ability to capture generalizable visual features. Instead of relying solely on textually-relevant images, we suggest incorporating a diverse range of visual data from various domains and sources during pre-training.
By exposing the VLM to images from multiple domains, we enable it to learn visual features that are not tied solely to the textual descriptions provided. This encourages the model to capture more abstract visual representations that can be adapted to different downstream tasks more effectively.
Combining Domain-Specific Knowledge
Another critical aspect of our proposed approach involves leveraging domain-specific knowledge to refine the pre-training process. While the inclusion of diverse images undoubtedly helps with generalizability, incorporating relevant domain-specific cues can further enhance the model’s performance.
For example, if the VLM is intended for medical image analysis, we can incorporate domain-specific labels, annotations, or even expert knowledge during pre-training. By doing so, the model can learn to identify specific features or patterns that are critical for diagnosing certain medical conditions. Similarly, for tasks like object recognition in satellite imagery, incorporating knowledge from the field of remote sensing can greatly improve the model’s accuracy.
Breaking Down Language Biases
One concern with VLMs is the potential biases that may be captured during pre-training. As these models learn from large-scale datasets, they may unintentionally adopt societal biases present within the text data. For instance, the association of certain words with particular genders or races is a well-documented issue in natural language processing.
To ensure fair and unbiased performance, it is essential to carefully curate the linguistic corpus used for pre-training. By actively identifying and removing biased language or imagery during the data collection stage, we can mitigate the risk of perpetuating harmful biases in VLMs.
Conclusion
Vision-Language Models have revolutionized image retrieval tasks, but there is still room for improvement. By expanding the selection of images used for pre-training and incorporating domain-specific knowledge, we can enhance the models’ ability to capture generalized visual features. Additionally, by curating the training data to remove biases, we can ensure fair and unbiased performance in downstream tasks. These innovative ideas pave the way for more robust and versatile VLMs, capable of addressing real-world challenges in computer vision.
in the form of captioned images, where each image is associated with a textual description. This approach allows the model to learn a joint representation of both visual and textual information, enabling it to understand the relationship between images and their corresponding captions.
One of the key advantages of pre-training vision-language models on large-scale datasets is the ability to capture a broad range of visual and textual information. By exposing the model to a diverse set of images and their associated captions, it can learn to recognize various objects, scenes, and concepts depicted in the images. Additionally, it learns to understand the semantics and context conveyed by the textual descriptions.
The pre-training process involves optimizing the model to predict the correct caption given an image or vice versa. This forces the model to learn a shared representation that captures the underlying meaning and connections between visual and textual modalities. As a result, the model becomes proficient in tasks such as image retrieval, where it can retrieve images relevant to a given textual query or vice versa.
However, there are still some challenges and limitations in vision-language models. One limitation is the reliance on the availability of large-scale annotated datasets for pre-training. Creating such datasets can be time-consuming, expensive, and require human effort for annotation. Moreover, biases present in the training data can be inadvertently learned by the model, leading to biased or unfair behavior.
To address these challenges, researchers are exploring techniques like data augmentation and transfer learning to improve the generalization capability of vision-language models. Data augmentation involves creating additional training examples by applying transformations such as cropping, rotating, or adding noise to the images. Transfer learning leverages pre-trained models on related tasks to initialize vision-language models, enabling them to learn faster and perform better on downstream tasks.
Looking ahead, one exciting direction for vision-language models is their application in more complex tasks, such as visual question answering or generating detailed image descriptions. These tasks require a deeper understanding of both visual and textual information, and further advancements in pre-training techniques can help in achieving better performance.
Additionally, there is a growing interest in addressing the biases present in large-scale image-text datasets. Efforts are being made to develop methods that mitigate biases and promote fairness in vision-language models. This includes techniques like debiasing algorithms, adversarial training, and careful dataset curation to ensure a more balanced representation of diverse perspectives.
In conclusion, pre-training vision-language models on large-scale image-text datasets has proven to be highly effective in improving performance in downstream tasks like image retrieval. With ongoing research and advancements, we can expect these models to continue evolving, enabling them to tackle more complex vision-language tasks and address the challenges of biases and fairness in their training data.
Read the original article