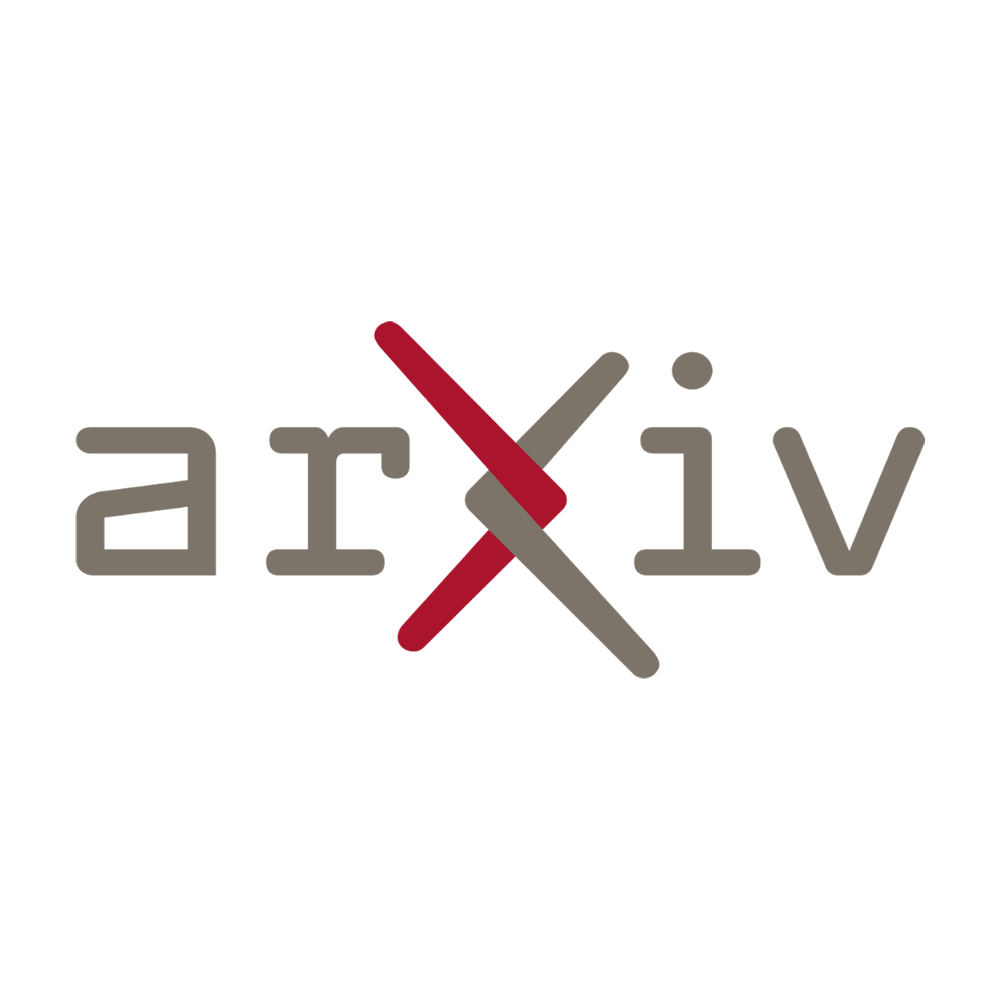
introduces a groundbreaking research study that focuses on the detection and prediction of survival and grade in glioblastoma, an extremely aggressive brain tumor. With a life expectancy of just 3 to 6 months without treatment, the accurate assessment of this tumor’s progression is of paramount importance. The article delves into the findings of this study, shedding light on the potential advancements in diagnosing and managing glioblastoma, ultimately offering hope for improved patient outcomes.
Glioblastoma: Exploring Innovative Solutions for Detection and Survival Prediction
Glioblastoma, a highly malignant brain tumor, is a devastating diagnosis. Without treatment, patients face a life expectancy of only 3 to 6 months. Detecting and predicting the survival and grade of glioblastoma accurately is crucial for improving treatment outcomes and extending patient lives. In this article, we will explore innovative solutions and ideas that could revolutionize the way we approach glioblastoma detection and survival prediction.
1. Artificial Intelligence for Accurate Diagnoses
Glioblastoma diagnosis heavily relies on interpreting MRI scans. However, this process can be time-consuming and subject to human error. By harnessing the power of artificial intelligence (AI), we can develop algorithms that analyze these scans with remarkable accuracy and efficiency.
AI algorithms can learn from vast datasets of MRI images to identify patterns and detect even the subtlest signs of glioblastoma. This technology can assist radiologists by providing instant feedback and reducing the chances of misdiagnosis.
2. Genomic Profiling and Personalized Medicine
Glioblastoma is a complex tumor with significant molecular heterogeneity. Traditional treatment approaches based on standard protocols often fall short in addressing these differences. Adopting genomic profiling can help identify the specific genetic alterations driving each patient’s glioblastoma.
With this knowledge, personalized medicine approaches can be tailored to target the unique genetic characteristics of each patient’s tumor. By combining genomic information with drug screening techniques, we can identify potential targeted therapies that may improve treatment outcomes and extend survival rates.
3. Promoting Collaborative Research
Due to the complexity and rarity of glioblastoma, conducting large-scale trials and studies can be challenging. A solution to this obstacle is promoting collaborative research efforts between different institutions, researchers, and clinicians.
Establishing a global network of glioblastoma researchers can facilitate data sharing, multidisciplinary collaborations, and faster progress. By pooling resources, data, and expertise, we can accelerate the development of novel detection techniques, treatment strategies, and predictive models.
4. Early Detection through Liquid Biopsies
Early detection is crucial for improving glioblastoma outcomes. Currently, diagnosis often occurs when symptoms become apparent or through invasive procedures, such as surgical biopsies. However, emerging technologies like liquid biopsies offer a less invasive and potentially earlier detection method.
Liquid biopsies involve analyzing the genetic material released by tumors into bodily fluids like blood or cerebrospinal fluid. By detecting specific tumor markers in these samples, we may have a non-invasive tool for early glioblastoma detection, allowing for timely interventions and increased survival rates.
5. Integrating Imaging and Biomarkers for Prognosis
To accurately predict survival and grade in glioblastoma, we need to consider multiple factors beyond imaging alone. By integrating imaging data with biomarkers associated with tumor aggressiveness and patient prognosis, we can develop comprehensive prediction models.
Beyond traditional radiological features, biomarkers can provide valuable insights into a patient’s overall health, immune response, and genetic predisposition to treatment response or resistance. By combining these variables, we can enhance the accuracy of survival predictions and tailor treatment plans accordingly.
In conclusion, glioblastoma presents significant challenges in detection and survival prediction. However, by embracing innovative solutions such as artificial intelligence, personalized medicine, collaborative research efforts, liquid biopsies, and integrated imaging-biomarker approaches, we have the opportunity to make substantial progress. Through these advancements, we can improve patient outcomes, extend survival rates, and ultimately work towards finding a cure for this devastating disease.
This study on detecting and predicting the survival and grade of glioblastoma is of significant importance in the field of brain tumor research. Glioblastoma is known for its aggressive nature and poor prognosis, with a life expectancy of only 3 to 6 months without treatment. Therefore, accurate detection and prediction of its survival and grade are critical for guiding treatment decisions and improving patient outcomes.
One of the key challenges in managing glioblastoma is its heterogeneity, meaning that the tumor can vary in terms of its genetic characteristics and response to treatment within an individual patient. This heterogeneity makes it difficult to accurately predict the tumor’s behavior and determine the most appropriate treatment strategy. Therefore, any advancements in detecting and predicting glioblastoma survival and grade would be highly valuable for clinicians and researchers.
The study likely employed various methods to analyze and interpret a large dataset of patient information, including clinical data, radiological imaging, and molecular profiling. Machine learning algorithms, such as deep learning or random forest models, may have been utilized to identify patterns and associations between different variables that could aid in predicting survival and determining the grade of glioblastoma.
It is crucial to note that accurate prediction of glioblastoma survival and grade can have a significant impact on treatment decisions. For instance, if a patient is predicted to have a more aggressive tumor with a shorter survival time, clinicians may opt for more intensive treatments, such as surgery followed by radiation and chemotherapy. On the other hand, if a patient is predicted to have a less aggressive tumor with a longer survival time, a more conservative treatment approach may be considered to minimize potential side effects.
While this study is undoubtedly a step forward in improving the accuracy of glioblastoma detection and prediction, there are still several challenges that need to be addressed. Firstly, the study’s findings should be validated in larger patient cohorts to ensure their generalizability. Secondly, incorporating longitudinal data and monitoring changes in tumor characteristics over time could enhance the accuracy of predictions. Additionally, the integration of novel biomarkers or imaging techniques, such as advanced MRI or PET scans, may provide further insights into glioblastoma behavior and improve prediction models.
Looking ahead, future research should focus on refining and validating these prediction models to ensure their reliability in real-world clinical settings. Moreover, efforts should be made to integrate these models into routine clinical practice, allowing clinicians to make more informed decisions regarding treatment strategies for glioblastoma patients. Ultimately, the goal is to improve patient outcomes by tailoring treatments based on accurate predictions of survival and grade, thereby maximizing the chances of success while minimizing unnecessary interventions.
Read the original article