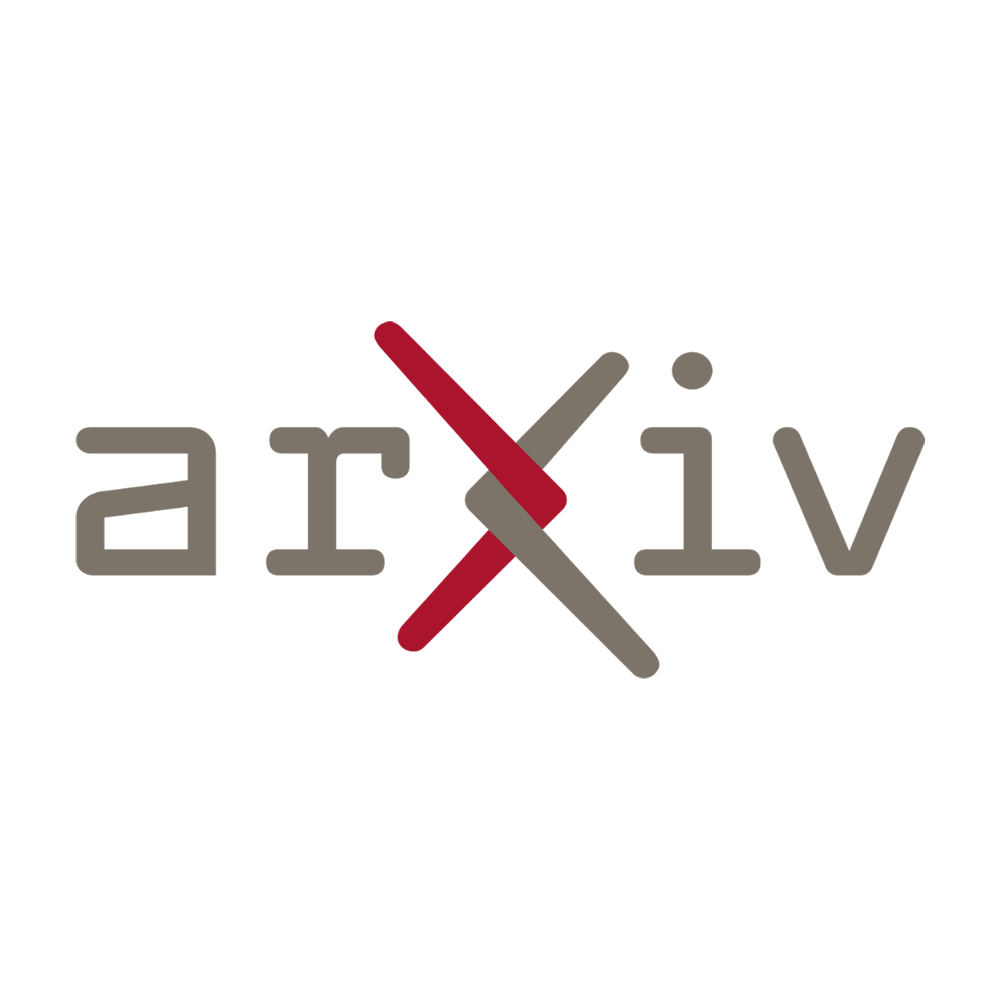
Accurate modeling of system dynamics is a crucial aspect in various scientific disciplines, such as cytodynamics and fluid mechanics, offering exciting possibilities for advancements. However, this task is often riddled with significant challenges, especially when (i)…
Exploring the Boundless Potential of Accurate System Dynamics Modeling
Accurate modeling of system dynamics holds intriguing potential in broad scientific fields including cytodynamics and fluid mechanics.
The Challenges of Accurate System Dynamics Modeling
Undoubtedly, the relevance of accurate modeling of system dynamics cannot be overstated. In various scientific disciplines, such as cytodynamics and fluid mechanics, understanding and predicting the behavior of complex systems rely heavily on the ability to create precise models. However, this task is not without its challenges.
Limited Data Availability
One of the primary hurdles in accurate system dynamics modeling is the scarcity of data. In many cases, experimental data might be limited or difficult to obtain. This creates a gap in knowledge, hindering the development of comprehensive models. Scientists must explore alternative methods to bridge this data gap and ensure their models are based on reliable information.
Complexities and Interactions
Systems found in cytodynamics and fluid mechanics are often highly complex and involve intricate interactions between various components. Capturing these complexities and interactions accurately in a model requires a deep understanding of the system’s underlying mechanisms. Researchers must invest significant time and effort into unraveling these complexities to develop robust models capable of capturing the true essence of the system.
Nonlinear Behavior
Many systems exhibit nonlinear behavior, meaning their output is not directly proportional to their input. Nonlinear dynamics are particularly challenging to model accurately, as they often result from intricate feedback loops and complex relationships between variables. Scientists must employ advanced mathematical techniques and algorithms to effectively model and simulate such nonlinear behavior.
Redefining System Dynamics Modeling: Innovative Solutions
While accurate system dynamics modeling presents challenges, researchers and scientists continue to innovate and propose novel solutions to overcome these obstacles. Here are a few innovative approaches that hold promise:
Data Integration and Machine Learning
To counter limited data availability, scientists can leverage data integration techniques to combine existing data sources from various domains. Additionally, machine learning algorithms can be used to extract meaningful patterns and insights from available data, enabling the creation of more informed models. This interdisciplinary approach enhances the accuracy and reliability of system dynamics modeling.
Agent-based modeling offers a unique way to capture complex interactions and behaviors within a system. By simulating individual agents and their interactions, researchers can gain valuable insights into emergent properties and system-level behavior. This approach can provide a deeper understanding of complex systems in cytodynamics and fluid mechanics, allowing for more accurate predictions and optimizations.
With advancements in computational power, multiscale modeling has become increasingly feasible. This approach involves modeling a system at different levels of detail simultaneously, capturing both macroscopic and microscopic phenomena. By integrating scales, researchers can bridge the gap between different spatial and temporal resolution requirements, facilitating accurate system dynamics modeling.
Embracing the Potential: Future Implications
The exploration of accurate system dynamics modeling and its innovative solutions has far-reaching implications across scientific disciplines. In cytodynamics, precise models can aid in understanding cellular behavior, leading to advancements in disease research and drug discovery. Similarly, in fluid mechanics, accurate models can enhance the efficiency of fluid flow simulations, optimizing design processes in various industries.
By embracing new modeling techniques and addressing the challenges head-on, scientists can unlock the true potential of accurate system dynamics modeling. As our understanding grows, we are poised for groundbreaking discoveries and transformative advancements across a multitude of scientific fields.
dealing with complex systems that contain a large number of interacting components or variables. One of the main challenges lies in accurately capturing the intricate relationships and interdependencies between these variables, as even small changes in one component can have a ripple effect throughout the entire system.
In the field of cytodynamics, accurate modeling of system dynamics is crucial for understanding the behavior of biological systems at various levels, from molecular interactions to cellular processes. For example, modeling the dynamics of gene regulatory networks can help unravel the complex mechanisms underlying cellular development and disease progression. By accurately representing the interactions between genes, proteins, and other molecules, researchers can simulate different scenarios and predict how changes in one component may affect the entire network.
Fluid mechanics is another scientific field that greatly benefits from accurate modeling of system dynamics. Fluids exhibit complex behavior, especially when considering turbulent flows or multiphase systems. Accurate modeling of fluid dynamics is crucial for optimizing industrial processes, designing efficient transportation systems, and understanding natural phenomena such as weather patterns and ocean currents.
One of the key advancements in accurate modeling of system dynamics is the use of computational methods, such as numerical simulations and computational fluid dynamics (CFD). These methods allow researchers to solve complex equations that govern the behavior of the system under consideration. However, these simulations often require massive computational resources and can be computationally expensive.
To address these challenges, researchers are constantly developing new techniques and algorithms to improve the accuracy and efficiency of modeling system dynamics. Machine learning approaches, for instance, have shown promise in optimizing simulations by learning from existing data and making predictions based on patterns and trends. Additionally, advancements in hardware technology, such as high-performance computing and parallel processing, enable faster and more detailed simulations.
Looking ahead, there are several exciting possibilities for the future of accurate modeling of system dynamics. With the increasing availability of big data and advancements in data analytics, researchers can incorporate real-world data into their models, making them more realistic and reliable. This integration of experimental data with modeling can lead to more accurate predictions and a deeper understanding of complex systems.
Furthermore, the development of hybrid modeling approaches that combine different modeling techniques, such as physics-based models and data-driven models, holds great potential. These hybrid models can leverage the strengths of each approach, allowing for more accurate representation of system dynamics while reducing computational costs.
In conclusion, accurate modeling of system dynamics is a challenging yet crucial task in scientific fields such as cytodynamics and fluid mechanics. Advances in computational methods, machine learning, and data analytics offer exciting opportunities for improving accuracy and efficiency. By continuously pushing the boundaries of modeling techniques and incorporating real-world data, researchers can gain deeper insights into complex systems and pave the way for future scientific advancements.
Read the original article