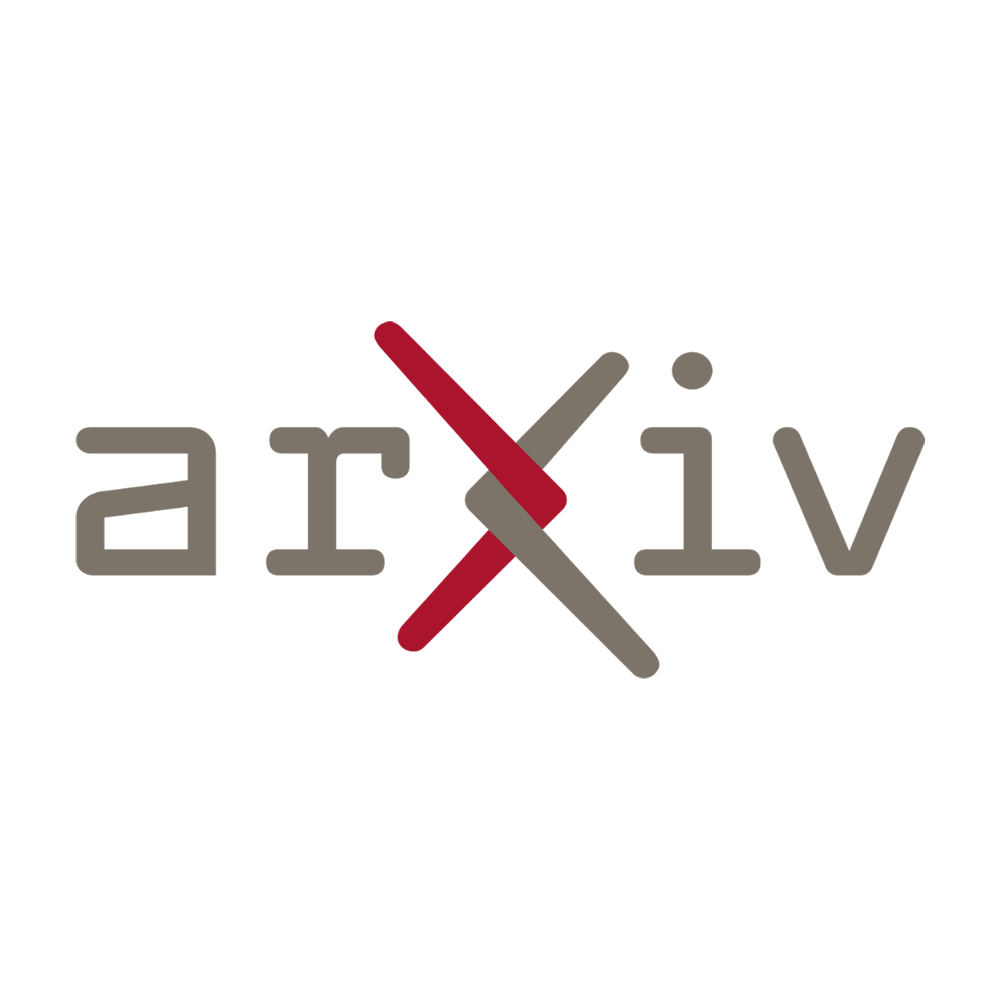
Text-to-image diffusion models (T2I) have revolutionized the field of image generation by utilizing a latent representation of a text prompt to create stunning visuals. These models have been widely successful in producing realistic and coherent images. However, the underlying process through which the encoder generates the text representation has remained a challenge. In this article, we delve into the intricacies of the encoder’s role in T2I models and explore the various techniques and advancements that have been made to enhance its performance. By understanding this crucial aspect, we can gain valuable insights into the inner workings of T2I models and further improve their ability to generate visually captivating images.
Text-to-image diffusion models (T2I) have revolutionized the field of image generation by utilizing a latent representation of a text prompt to guide the image generation process. These models have garnered immense attention due to their ability to generate realistic and coherent images based on textual descriptions. However, despite their success, there are still underlying themes and concepts that can be explored further to propose innovative solutions and ideas for enhancing the T2I models.
The Process of Latent Representation
The core of any T2I model lies in the process of encoding text prompts into a latent representation that can then be used for generating images. This encoding process determines the success and quality of the generated images. However, there is an opportunity to consider alternative encoding techniques that can potentially improve the representation of the text prompts.
An innovative solution could be to incorporate semantic analysis techniques that delve deeper into the textual content. By understanding the contextual relationship between words and phrases, the encoder can create a more robust latent representation. This could involve techniques such as syntactic parsing, word sense disambiguation, and entity recognition. By incorporating these techniques, the T2I model can capture more nuanced information from the text prompts, resulting in more accurate and diverse image generation.
Exploration of Multi-modal Representations
While T2I models utilize text prompts to generate images, there is scope for further exploration of multi-modal representations. By incorporating additional modalities such as audio, video, or even haptic feedback, T2I models can generate images that not only capture the essence of the textual prompt but also incorporate information from other sensory domains.
For instance, imagine a T2I model that generates images based on a description of a beautiful sunset and accompanying calm and soothing music. By incorporating both text and audio modalities, the resulting image can capture not only the visual components of the sunset but also evoke the emotional experience associated with the music.
Dynamic Text Prompts for Interactive Generation
Current T2I models generate images based on static text prompts, limiting the interactive potential of these models. To introduce more interactivity, an innovative solution could involve the use of dynamic text prompts. These prompts can change and evolve based on user feedback or real-time interactions.
Consider a T2I model used in a game environment where users describe objects they want to see within the game world. Instead of relying on a single static text prompt, the model can adapt and generate images iteratively based on real-time user inputs. This would create an interactive and dynamic experience, allowing users to actively participate in the image generation process.
Conclusion
Text-to-image diffusion models have revolutionized image generation, but there is still room for exploration and innovation in the field. By delving into the encoding process, incorporating multi-modal representations, and introducing dynamic text prompts, T2I models can reach new heights of image generation capabilities. These proposed solutions and ideas open up exciting possibilities for the future of T2I models and their applications in various domains.
is a crucial component in the effectiveness and quality of the generated images. The encoder’s role is to capture the semantic meaning of the input text and convert it into a latent space representation that can be easily understood by the image generator.
One of the challenges in designing an effective encoder for T2I models is ensuring that it can extract the relevant information from the text prompt while discarding irrelevant or misleading details. This is especially important in cases where the text prompt is long or contains ambiguous phrases. A well-designed encoder should be able to focus on the key aspects of the text and translate them into a meaningful representation.
Another important consideration in encoder design is the choice of architecture. Different architectures, such as recurrent neural networks (RNNs) or transformer models, can be used to encode the text prompt. Each architecture has its strengths and weaknesses, and the choice depends on factors like computational efficiency and the ability to capture long-range dependencies in the text.
In addition to the architecture, the training process of the encoder is crucial. It is essential to have a diverse and representative dataset that covers a wide range of text prompts and their corresponding images. This ensures that the encoder learns to generalize well and can handle various input scenarios effectively.
Furthermore, ongoing research is focused on improving the interpretability and controllability of the latent representation generated by the encoder. This can enable users to have more fine-grained control over the generated images by manipulating specific attributes or characteristics in the text prompt. Techniques such as disentangled representation learning and attribute conditioning are being explored to achieve this goal.
Looking ahead, the future of T2I models lies in enhancing the quality and diversity of the generated images. This can be achieved by further improving the encoder’s ability to capture nuanced information from the text prompt and by refining the image generation process. Additionally, incorporating feedback mechanisms that allow users to provide iterative guidance to the model can lead to more personalized and accurate image generation.
Overall, the development of text-to-image diffusion models has opened up exciting possibilities in various domains, including creative content generation, virtual environments, and visual storytelling. Continued advancements in encoder design, training methodologies, and interpretability will play a vital role in unlocking the full potential of these models and revolutionizing how we interact with visual content.
Read the original article