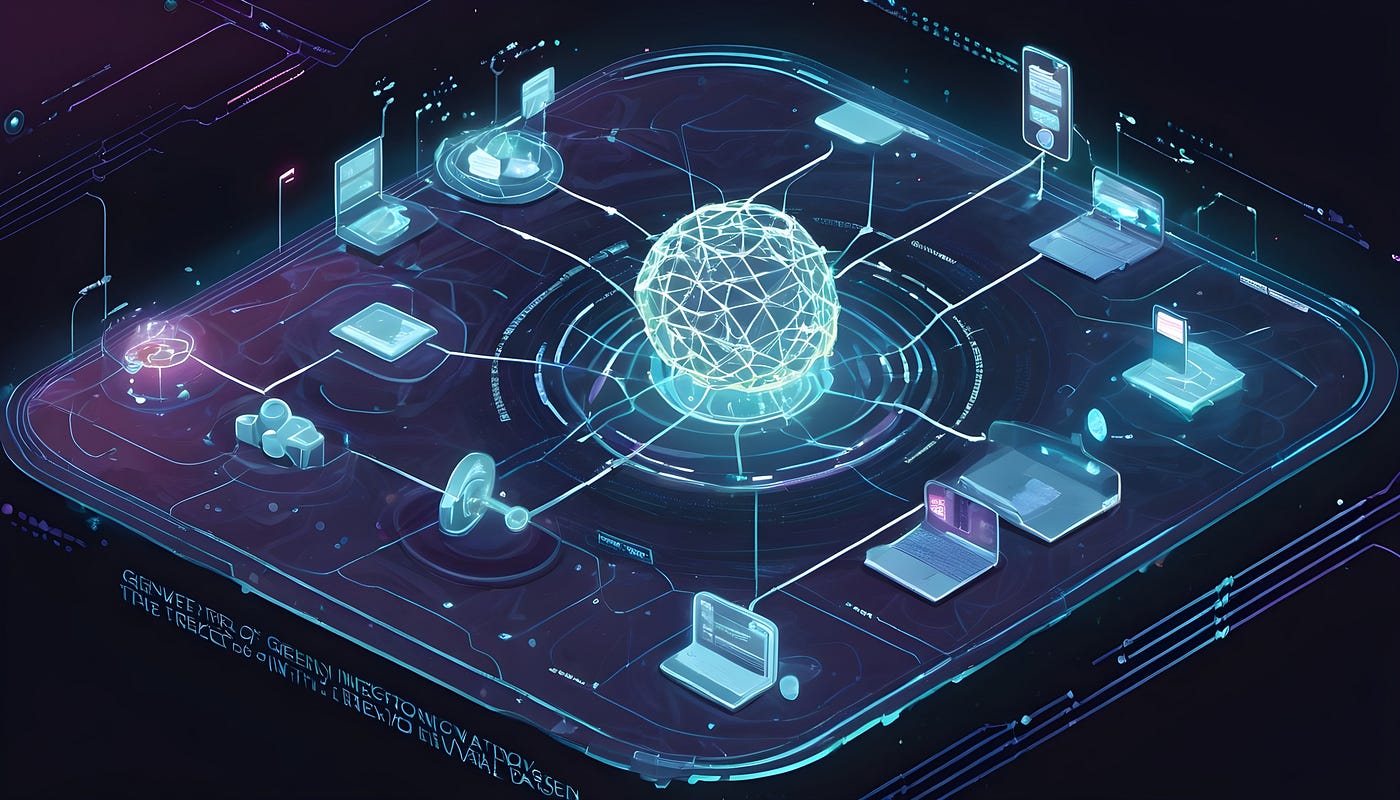
Analyzing the Impact and Future of Retrieval-Augmented Generation in Generative AI
In the dynamic world of artificial intelligence (AI), Retrieval-Augmented Generation (RAG) has emerged as a promising, novel framework that holds the potential to transform Generative AI’s capabilities. RAG leverages the power of both retrieval-based and generative models, effectively combining their strengths to generate highly precise and relevant text. The key techniques underpinning RAG’s success include, but not limited to, RetrievalQA, MultiqueryRetriever, Vector Store-backed retriever, Hybrid Search using BM25Retriever, and Contextual Compression.
Long-term Implications and Future Developments of RAG
Observing the significant strides RAG has already made in the Generative AI landscape, it’s clear that it’s likely to hold numerous long-term implications. RAG might play a major part in multiple fields like automated customer assistance, creative writing assistance, and content curation, among others. It is worth exploring these potential ramifications and possible future developments linked with this AI technique.
RAG’s Role in Revolutionizing Customer Assistance
One of the most significant implications of RAG is how it could potentially augment automated customer service systems. Given its ability to generate precise and relevant text, RAG may allow AI chatbots to respond more coherently and contextually to customer queries, thus enhancing customer experience and creating more interactive automated assistant platforms.
Impact on Content Creation and Curation
RAG’s strengths could also be leveraged in the realm of creative writing assistance and content curation. This technique, with its ability to generate high-quality, relevant content, can be used in AI-powered content generation tools, making it easier for creators to generate and curate engaging and value-added content.
Future Developments in RAG
The potential scope for future developments in RAG is vast and exciting. Enhancements in the constituent techniques of RAG like RetrievalQA, MultiqueryRetriever and others could lead to further advances. For instance, more accurate Vector Store-backed retrievers might improve the quality of search results while Improvements in Contextual Compression might enhance the overall text generation process.
Actionable Advice Based on These Insights
- Companies that rely on AI to drive their customer-facing operations should monitor developments in RAG closely, with a view to incorporate latest advancements to enhance customer service.
- Content creators and creative professionals can consider leveraging AI tools powered by RAG to streamline their content generation and curation process.
- Stakeholders invested in AI development should prioritize refining and enhancing the underlying techniques of RAG for achieving higher precision and relevant text generation.
Retrieval-Augmented Generation, as the name suggests, stands poised to redefine the landscape of Generative AI. Staying alert to its potential and working persistently to maximize its capabilities will yield game-changing results for many applications.