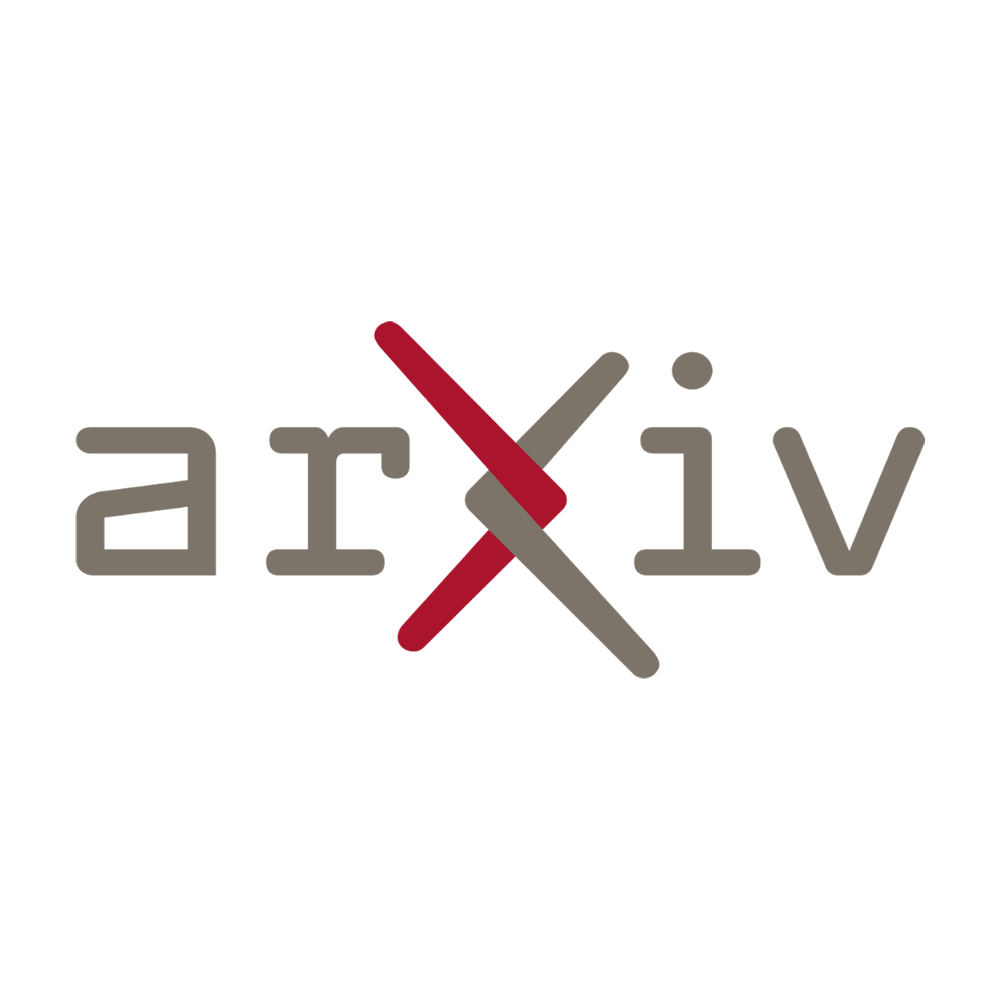
Action recognition from video data is a crucial field with extensive applications, but traditional single-view action recognition has its limitations. Relying solely on a single viewpoint, it fails to capture the full complexity and variability of actions. However, a new approach is emerging that overcomes these limitations, enabling a more comprehensive understanding of actions. By incorporating multiple viewpoints and leveraging the power of advanced algorithms, this innovative method promises to revolutionize action recognition and open up a world of possibilities for various industries and research domains.
Action recognition from video data has become an essential component for various applications in today’s technology-driven world. It enables us to analyze, understand, and predict human actions, providing valuable insights for fields such as surveillance, robotics, healthcare, and even entertainment.
Traditionally, single-view action recognition has been the dominant approach, relying on a single viewpoint to detect and classify actions. However, this approach has its limitations. It fails to capture the full context of an action, as it is restricted by the viewpoint from which the video was recorded. As a result, it may struggle with accuracy and robustness when dealing with complex and ambiguous actions.
To overcome these limitations, recent advancements have shifted the focus towards multi-view action recognition. This approach aims to utilize multiple viewpoints of a video sequence, capturing different perspectives and angles of an action. By combining these viewpoints, a more comprehensive understanding of the action can be achieved, leading to improved accuracy and generalization.
One innovative solution that has gained traction in multi-view action recognition is the use of deep learning techniques. Deep learning models, such as convolutional neural networks (CNNs) and recurrent neural networks (RNNs), have demonstrated remarkable success in various computer vision tasks, including single-view action recognition. By extending these models to incorporate multi-view data, researchers have achieved significant improvements in performance.
Another promising direction in multi-view action recognition is the integration of temporal information. Actions are inherently dynamic, and their temporal evolution plays a crucial role in understanding their semantics. By modeling the temporal dynamics of actions across multiple viewpoints, we can further enhance the discriminative power of our recognition systems. This can be achieved through recurrent architectures, temporal convolutional networks, or attention mechanisms that focus on relevant temporal segments.
Furthermore, the combination of multi-view action recognition with other modalities, such as depth or skeleton data, holds great potential. Depth information provides additional cues about the 3D structure of actions, while skeleton data captures the joint movements of a person. By fusing these modalities with multi-view data, we can create more robust and comprehensive models, capable of capturing finer details and nuances of actions.
In conclusion, multi-view action recognition offers a promising alternative to single-view approaches, addressing their limitations and expanding the possibilities of action analysis. By leveraging multiple viewpoints, incorporating deep learning techniques, modeling temporal dynamics, and integrating other modalities, we can improve the accuracy, robustness, and generalization of action recognition systems. These advancements have the potential to revolutionize various domains, from surveillance and robotics to healthcare and entertainment, opening up new frontiers in understanding human actions and behaviors.
multi-view action recognition utilizes multiple viewpoints to capture a more comprehensive understanding of actions. This approach has gained significant attention in recent years, as it offers improved accuracy and robustness compared to single-view methods.
One of the key advantages of multi-view action recognition is its ability to capture the spatial and temporal dynamics of actions from different angles. By fusing information from multiple viewpoints, it becomes possible to overcome occlusions and ambiguities that often arise in single-view scenarios. This is particularly useful in complex and cluttered environments, where actions may be partially obstructed or obscured.
Another important aspect of multi-view action recognition is its potential for enhancing the generalizability of action recognition models. By training on diverse viewpoints, the models can learn to recognize actions from different perspectives, making them more adaptable to real-world scenarios. This is especially crucial in applications such as surveillance, robotics, and human-computer interaction, where actions can be observed from various angles.
However, multi-view action recognition also comes with its own set of challenges. One major challenge is the synchronization of multiple viewpoints. Ensuring that the different camera views are temporally aligned is crucial for accurate action recognition. Additionally, the fusion of information from multiple views requires careful consideration to avoid information redundancy or loss.
To address these challenges, researchers have been exploring various techniques, such as view transformation, view-invariant feature extraction, and view fusion methods. These approaches aim to effectively combine information from different viewpoints and create a unified representation that captures the essence of the action.
Looking ahead, the future of multi-view action recognition holds great potential. With advancements in camera technologies and the increasing availability of multi-camera setups, the quality and quantity of multi-view video data are expected to improve. This will enable the development of more sophisticated models that can leverage multiple viewpoints to achieve even higher accuracy and robustness.
Moreover, the integration of deep learning techniques, such as convolutional neural networks (CNNs) and recurrent neural networks (RNNs), has shown promising results in multi-view action recognition. These models can effectively learn spatiotemporal patterns from multiple viewpoints, further enhancing the discriminative power and generalizability of the recognition system.
Furthermore, the combination of multi-view action recognition with other modalities, such as depth information from depth sensors or audio signals, could lead to even more comprehensive and accurate action understanding. This multimodal fusion has the potential to unlock new applications, such as human behavior analysis, interactive gaming, and immersive virtual reality experiences.
In conclusion, multi-view action recognition has emerged as a powerful approach to overcome the limitations of single-view methods. Its ability to capture spatial and temporal dynamics from multiple viewpoints offers improved accuracy and robustness. While challenges remain, ongoing research and advancements in technology hold great promise for the future of multi-view action recognition, paving the way for more sophisticated and versatile action understanding systems.
Read the original article