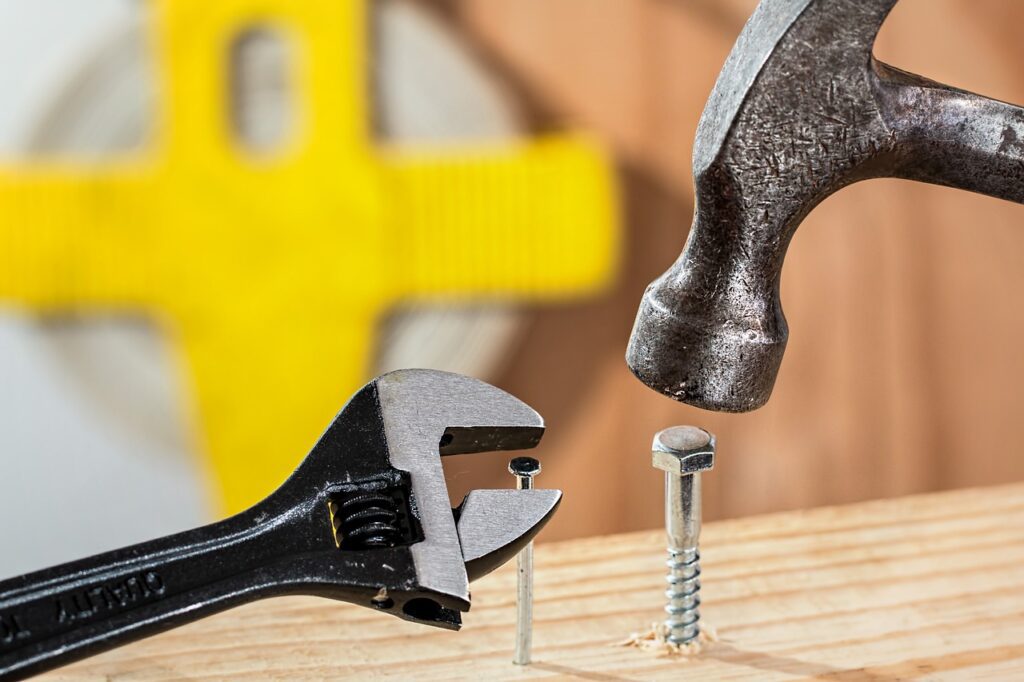
Analysis of Lowest Common Denominator Data Science
It’s important to delve deeper into the concept of Lowest Common Denominator (LCD) Data Science and evaluate the long-term implications and potential future developments in this area. LCD Data Science refers to a lack of critical thinking, not questioning the prevailing wisdom nor trying to counter it. This approach appears to be dominant in the current data science landscape and has unfortunately triggered a wide array of damaging side effects.
Long-Term Implications
An impact of LCD Data Science is its potential to negatively affect more thoughtful approaches to Artificial Intelligence (AI). In the long term, this could stifle innovation and progress within the field. AI depends greatly on creative and critical thinking – to negate these aspects through LCD Data Science raises concerns for its future progress.
Additionally, LCD Data Science runs the risk of reinforcing existing biases and assumptions, which can lead to misguided models and predictions. In turn, this could result in real-world negative consequences, such as decisions based on skewed data, perpetuating systematic biases.
Possible Future Developments
Considering the current direction, it is plausible that unless trends change, LCD Data Science might continue to prevail. This would lead to a suppression of critical thinking, hampering the process of discovery, innovation, and progress within data science. However, the opposite might also occur, with a backlash against LCD Data Science leading to a greater emphasis on thoughtful, critical approaches to AI and Data Science.
Actionable Insights
Bearing these implications and potential developments in mind, the following recommendations can be made:
- Encourage critical thinking: Institutions and businesses involved in AI and data science should prioritize critical thinking and challenge the current LCD approach.
- Invest in training and education: Investment in the education of data scientists in the importance of thoughtfulness and unconventional wisdom can help counter the impacts of LCD data science.
- Promote ethical considerations: Ethical considerations should be brought to the fore to prevent the entrenchment of biases and improve the relevance and accuracy of models and predictions.
- Advocate for openness and transparency: An open, transparent approach to AI will foster an environment of critique, collaboration, and progress.
By focusing on these actions, the damaging effects of LCD Data Science can be mitigated and more thoughtful approaches to AI can flourish. The hope is that these recommendations, if applied diligently, might help steer the field towards a future of meaningful innovation and discovery.