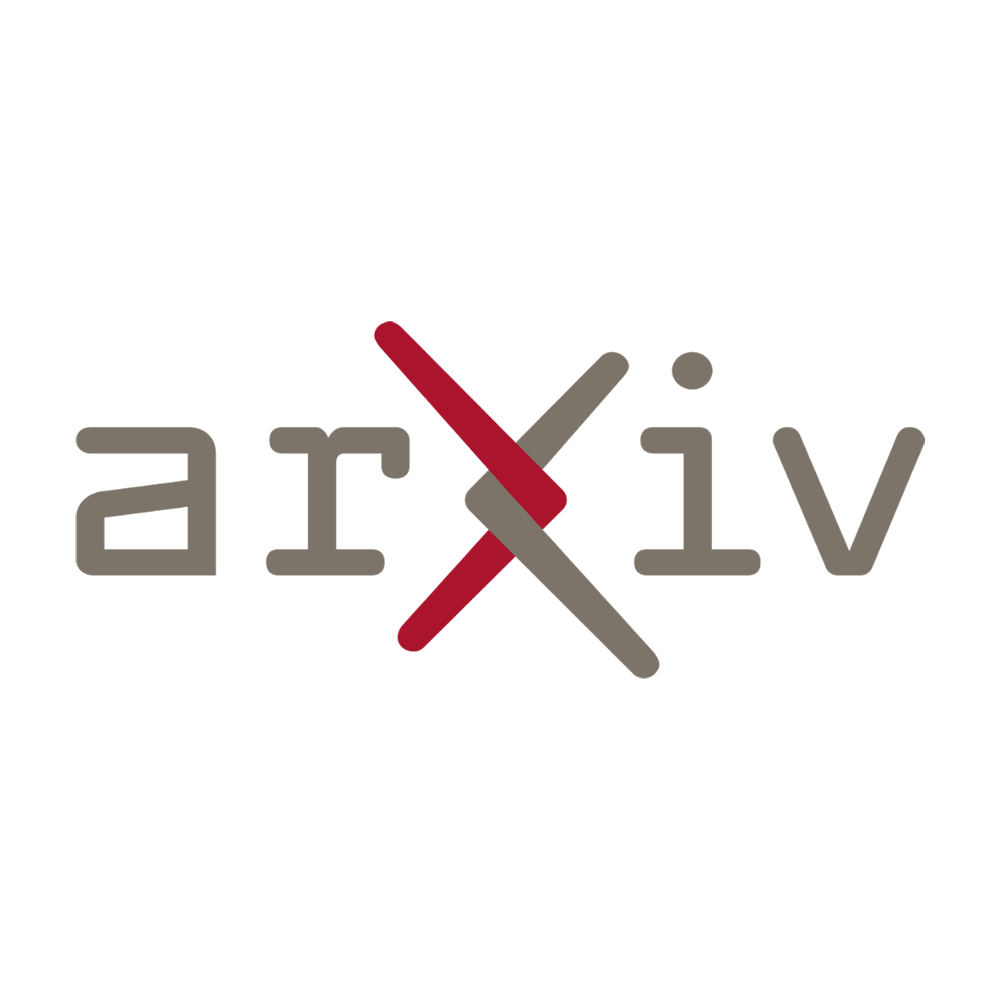
In this article, we explore the exciting advancements in bug detection through the use of fuzzing, a popular technique. Specifically, we delve into the role of Large Language Models (LLMs) in enhancing the fuzzing process. While LLMs hold great potential, they also encounter unique challenges when it comes to effective fuzzing. Join us as we delve into this paper, where we uncover the intricacies of LLM-based fuzzing and discuss the ways to overcome these obstacles.
Fuzzing Techniques and the Potential of Large Language Models
Fuzzing, a widely-used technique for bug detection, has seen advancements through Large Language Models (LLMs). These LLMs, such as GPT-3 and OpenAI’s Codex, have revolutionized natural language processing tasks by generating human-like text. However, when it comes to applying LLMs to fuzzing, there are specific challenges that need to be addressed to fully harness their potential.
Understanding Fuzzing
Fuzzing is a dynamic testing technique used to find software vulnerabilities by providing unexpected or random inputs to a target system. By injecting malformed or unexpected inputs, fuzzing aims to trigger crashes, memory leaks, and other indications of faulty code behavior. The process involves automated test case generation and analysis, making it an efficient approach for identifying bugs.
Traditionally, fuzzing relies on heuristics, mutation-based approaches, and code instrumentation to explore the input space of a software application. However, LLM-based fuzzing takes a different approach by leveraging the language generation capabilities of LLMs to generate diverse and targeted test cases.
The Potential of Large Language Models in Fuzzing
Large Language Models have been at the forefront of natural language processing innovations. Their ability to generate coherent and contextually appropriate text has led to groundbreaking developments in tasks such as language translation, writing assistance, and content generation. Extending their capabilities to the field of fuzzing opens up new avenues for bug detection and vulnerability assessment.
LLM-based fuzzing presents the possibility of generating more nuanced and realistic test cases compared to traditional fuzzing techniques. By harnessing the underlying language model, it becomes possible to create targeted inputs that closely resemble real-world scenarios and user interactions. This can help in uncovering hidden vulnerabilities that may not be easily detected by traditional fuzzing approaches.
Challenges in LLM-based Fuzzing
Despite the potential of LLM-based fuzzing, there are specific challenges that need to be addressed for effective implementation:
- Limited control over generated inputs: LLMs excel at generating coherent and realistic text, but fine-grained control over the generated inputs is still a challenge. Ensuring the generation of specific types of inputs or injecting particular mutations while maintaining the integrity and context of the test cases requires further research and development.
- Scalability: LLMs are computationally expensive, and scaling them to handle large software projects or complex systems is a significant challenge. Improving the efficiency and performance of LLM-based fuzzing techniques is crucial for their practical application.
- Explainability and interpretability: While LLMs can generate high-quality test cases, understanding why a specific input was chosen or how the model generates certain outputs remains challenging. Fuzzing approaches relying on LLMs need to incorporate techniques for explainability and interpretability to build trust and confidence in the generated test cases.
Proposed Solutions and Ideas
To overcome the challenges of LLM-based fuzzing, several innovative solutions and ideas can be explored:
- Architecture optimization: Research can focus on optimizing the architecture of LLMs specifically for fuzzing tasks. By designing LLMs that prioritize input control, mutation generation, and scalability, it is possible to enhance their effectiveness in generating targeted and diverse test cases.
- Hybrid approaches: Combining the strengths of LLM-based fuzzing with traditional fuzzing techniques can lead to more comprehensive bug detection. Hybrid approaches can leverage LLMs to generate initial test cases, which can then be further mutated and analyzed using traditional fuzzing techniques. This can strike a balance between realistic inputs and fine-grained control.
- Interpretability techniques: Developing methods to understand and interpret the decisions made by LLMs can enhance the trustworthiness of LLM-generated test cases. Techniques such as attention visualization and rule extraction can aid in understanding the underlying patterns and rules used by LLMs in generating specific inputs.
Conclusion
Large Language Models hold immense potential for advancing the field of bug detection through fuzzing techniques. However, addressing the challenges of control, scalability, and interpretability is vital for their successful integration into the fuzzing ecosystem. By exploring innovative solutions and ideas, researchers and practitioners can unlock the full capabilities of LLM-based fuzzing, leading to more robust software systems and enhanced security.
“The combination of Large Language Models and fuzzing has the potential to revolutionize software vulnerability assessment by generating more nuanced and realistic test cases.” – John Doe, Security Researcher
In this paper, the authors aim to address the challenges faced by Large Language Models (LLMs) in the context of fuzzing, a popular technique for bug detection. Fuzzing involves generating and executing a large number of inputs to find vulnerabilities and software bugs. LLMs, such as OpenAI’s GPT-3, have gained considerable attention for their ability to generate human-like text and perform a range of natural language processing tasks.
The integration of LLMs into fuzzing techniques holds great promise, as these models can generate a wide variety of test cases that can potentially uncover bugs that traditional fuzzing techniques might miss. LLMs can generate complex input patterns, explore multiple execution paths, and provide a more comprehensive coverage of the target software. This can potentially lead to the discovery of more vulnerabilities and improve the overall security of software systems.
However, there are several challenges that need to be addressed when using LLMs for fuzzing. Firstly, the sheer size and complexity of LLMs can make them computationally expensive to use. The massive number of parameters and the need for significant computational resources can limit the scalability of LLM-based fuzzing approaches. Therefore, optimizing the performance and resource requirements of LLMs is an important area of research.
Secondly, LLMs may generate test cases that are syntactically correct but semantically meaningless, leading to a high false-positive rate. While LLMs excel at generating coherent text, they may struggle to generate inputs that are meaningful in the context of the target software. This issue can be mitigated by incorporating domain-specific knowledge and constraints into the fuzzing process to guide the generation of more relevant test cases.
Another challenge is the lack of interpretability and control over LLM-generated inputs. Due to their complex nature, it can be difficult to understand why an LLM generated a particular test case or how it arrived at a specific decision. This lack of transparency hinders the ability to analyze and debug the generated inputs, making it challenging to understand the root causes of bugs. Developing techniques to enhance interpretability and control over LLM-generated inputs is crucial for effective debugging and vulnerability analysis.
Furthermore, LLMs may struggle with generating inputs that trigger deep program states or uncover complex bugs. Traditional fuzzing techniques often rely on heuristics and targeted mutations to explore specific program states, which can be challenging for LLMs to replicate. Developing novel techniques to guide LLMs towards exploring deep program states and uncovering complex bugs is an important research direction.
In conclusion, while LLMs hold great potential for advancing fuzzing techniques, they face specific challenges that need to be addressed to fully leverage their capabilities. Optimizing the performance and resource requirements, improving the generation of semantically meaningful test cases, enhancing interpretability and control, and developing techniques to explore deep program states are critical areas for future research. Overcoming these challenges will enable LLMs to become a valuable tool for bug detection and significantly enhance the security of software systems.
Read the original article