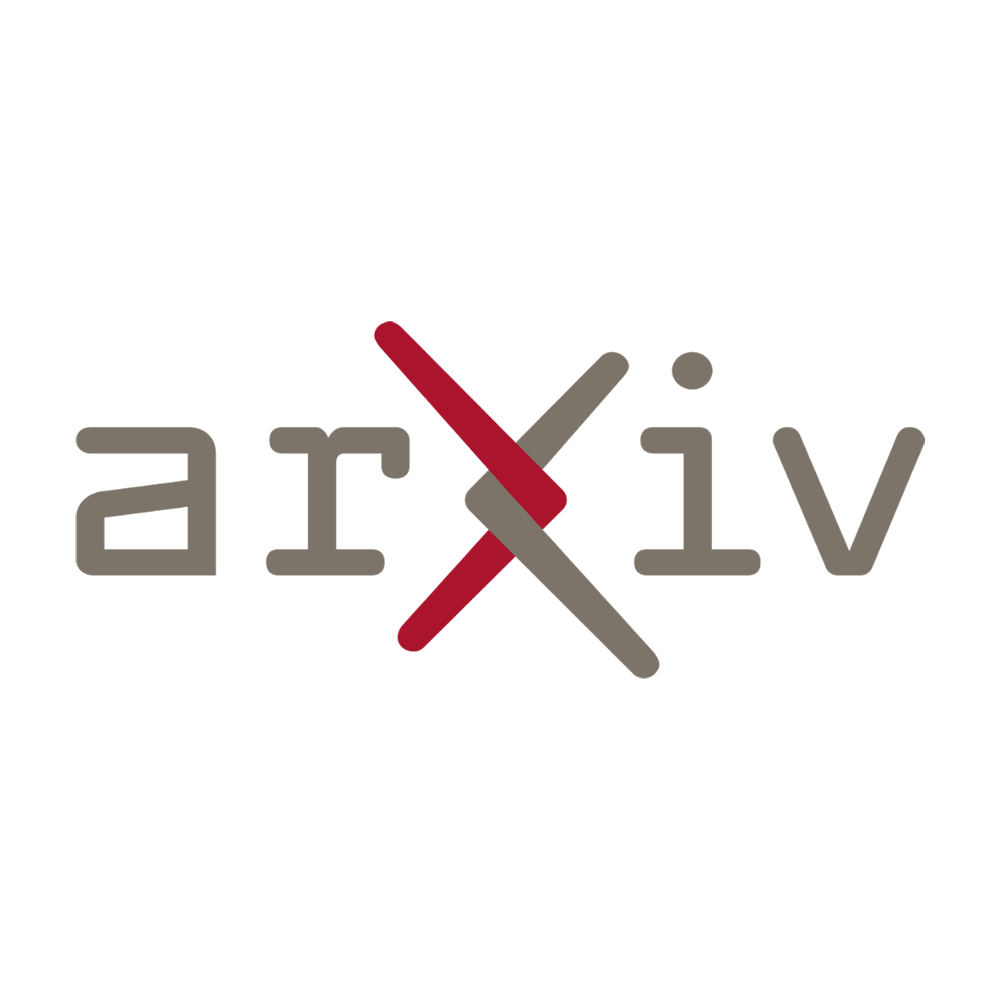
and prone to errors. To address these challenges, researchers have turned to synthetic data generation, a technique that uses computer algorithms to create realistic and diverse datasets for training AI models. In this article, we explore the benefits and limitations of synthetic data generation in AI training, and how it is revolutionizing the data annotation industry. We delve into the advancements in algorithms and technologies that enable the creation of high-quality synthetic data, and discuss its potential applications across various domains. Furthermore, we examine the ethical considerations surrounding the use of synthetic data and its impact on the future of AI development. Join us as we delve into the world of synthetic data generation and its role in shaping the future of AI training.
and prone to errors. As the need for high-quality labeled data increases, so does the need for efficient and accurate data annotation methods.
One innovative solution to this problem is the use of AI itself to assist in data annotation. By utilizing AI algorithms, we can automate parts of the annotation process and reduce the workload on human annotators. This not only speeds up the process but also improves the overall accuracy of annotations.
One such AI-powered annotation method is active learning. Active learning involves training a machine learning model to actively select the most informative samples for annotation. By doing so, the model can learn from a smaller subset of data while still achieving high accuracy. This approach significantly reduces the time and effort required for annotation, as the model learns to identify patterns and make predictions with minimal human intervention.
Another innovative approach is the use of semi-supervised learning. Traditional annotation methods rely on fully labeled datasets where each data point is labeled by human annotators. However, in many cases, obtaining such fully labeled datasets can be expensive and time-consuming. Semi-supervised learning addresses this issue by utilizing both labeled and unlabeled data. The model is initially trained on a small set of labeled data, and then it utilizes the unlabeled data to improve its performance over time. This approach reduces the dependency on fully annotated datasets and allows for faster and more cost-effective annotation.
Furthermore, the use of synthetic data generation techniques can also play a crucial role in data annotation. Synthetic data refers to artificially generated data that mimics the characteristics and patterns of real-world data. By generating synthetic data, we can create large-scale labeled datasets quickly and easily. However, it is essential to ensure that the synthetic data accurately represents the real-world scenarios to avoid bias or inaccurate labeling.
Additionally, collaborative annotation platforms have emerged as a solution to handle large-scale annotation tasks. These platforms bring together a community of annotators who can work collectively on labeling projects. By dividing the work among multiple annotators, these platforms enable faster annotation and provide a mechanism to resolve disagreements and ensure high-quality annotations.
In conclusion, the demand for AI training data has led to the growth of the data annotation industry. However, to meet this increasing demand, traditional annotation methods need to be enhanced and innovated. The use of AI in data annotation, through active learning and semi-supervised learning, can significantly improve efficiency and accuracy. Additionally, synthetic data generation techniques and collaborative annotation platforms offer further innovative solutions to address the challenges associated with large-scale annotation tasks. By embracing these new approaches, we can ensure the availability of high-quality labeled datasets for training AI models and continue advancing the field of artificial intelligence.
and prone to errors. As a result, there has been a significant shift towards using AI-powered solutions to automate the data annotation process. This not only speeds up the process but also ensures higher accuracy and consistency in the labeled data.
One of the key challenges in AI training data annotation is the need for large quantities of high-quality labeled data. This is crucial for training machine learning models effectively. However, manually annotating vast amounts of data can be a daunting task, requiring a substantial workforce and time investment.
The emergence of AI-powered annotation tools and techniques has revolutionized the industry. These tools leverage various techniques such as computer vision, natural language processing, and machine learning algorithms to automate the annotation process. By reducing human involvement, these tools can significantly accelerate the data annotation process while maintaining a high level of accuracy.
Furthermore, AI-powered annotation tools can learn from human annotations and gradually improve their performance over time. This iterative process allows the tools to reach a level of accuracy that can rival or even surpass human annotators. This is particularly beneficial in domains where the availability of human annotators is limited or where there is a need for large-scale annotation tasks.
However, it is important to note that AI-powered annotation tools are not a one-size-fits-all solution. While they excel in certain domains like image and speech recognition, there are still challenges in more complex tasks that require human expertise and contextual understanding. For instance, annotating medical images or legal documents may require domain-specific knowledge that AI algorithms may struggle to comprehend accurately.
Looking ahead, the future of AI training data annotation lies in a hybrid approach that combines the strengths of both human annotators and AI-powered tools. Human annotators can provide the necessary domain expertise, contextual understanding, and handle complex annotation tasks, while AI tools can assist in speeding up the process, ensuring consistency, and reducing human errors.
Furthermore, as AI algorithms continue to advance, we can expect to see more sophisticated annotation tools that can handle complex tasks with higher accuracy. These tools may incorporate advanced techniques such as active learning, where the algorithm intelligently selects the most informative data points for annotation, optimizing the annotation process even further.
In conclusion, the demand for AI training data annotation is driving the transformation of the industry. AI-powered annotation tools have the potential to revolutionize the process by automating it, reducing time and labor requirements, and improving accuracy. However, human annotators will continue to play a crucial role in complex annotation tasks, and a hybrid approach is likely to be the way forward. The future holds exciting possibilities for the evolution of AI training data annotation, with advancements in both AI algorithms and human-AI collaboration.
Read the original article