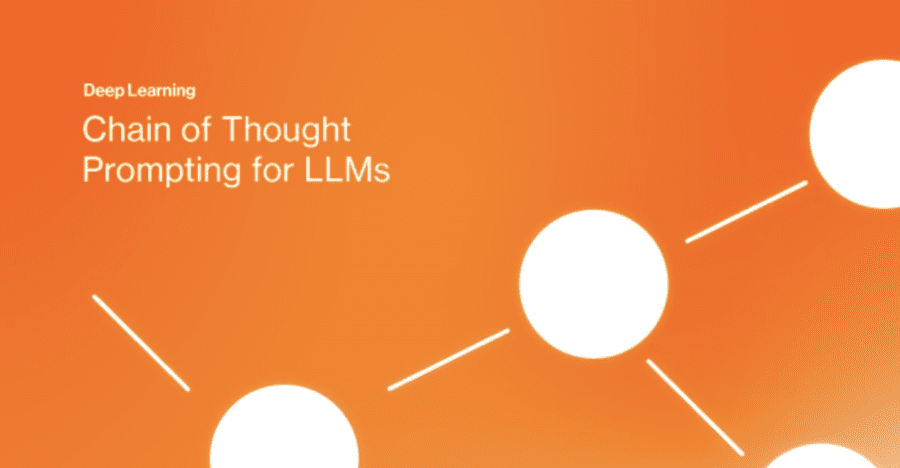
The Impact and Future of Chain-of-Thought Process in LLMs and AI Performance
With the rapid advancement of machine learning and artificial intelligence (AI), language models like Large Language Models (LLMs) are becoming increasingly important. A key aspect of enhancing AI capabilities in these models is chain-of-thought prompting, which shows the potential to improve problem-solving and overall performance in tasks related to mathematics and reasoning. This article will analyze this topic in more depth and provide recommendations for future actions.
Understanding Chain-of-Thought Prompting
Chain-of-thought prompting essentially integrates problem-solving by allowing AI systems to generate more complex responses. By creating a series of prompts or questions for the AI to answer, chain-of-thought prompting enables these systems to effectively tackle tasks that require a longer thought process, such as advanced mathematical and reasoning problems.
Chain-of-Thought Prompting: Long-Term Implications
Enhanced AI Problem-Solving Capabilities
As AI technology becomes more advanced and integrated into everyday life, the importance of sophisticated problem-solving skills in AI systems grows. Chain-of-thought prompting fundamentally enhances the problem-solving abilities of AI, as it forces these systems to consider a problem from multiple angles or steps before generating a response. This results in more accurate and complex responses that better reflect human thinking patterns.
Boosting AI Performance in Math and Reasoning Tasks
Improved problem-solving skills inevitably lead to better AI performance in math-based and reasoning tasks. Chain-of-thought prompting pushes the AI system beyond simple one-step problems to tackle multistage tasks, mirroring the way humans approach complicated problems. In the long term, this could significantly improve AI capabilities in sectors that require advanced mathematic and reasoning skills, such as scientific research, financial forecasting, and even policy formulation.
The Future of Chain-of-Thought Prompting in LLMs
Given its potential to significantly enhance AI problem-solving abilities, chain-of-thought prompting is likely to be integrated into more LLMs and AI systems in the future. Researchers may also focus more on developing more sophisticated forms of this technology, potentially creating AI systems that match or even surpass human cognitive capabilities in the long term.
Actionable Advice
- Invest in the development of improved chain-of-thought prompting algorithms: As AI continues to evolve, there is bound to be an increased demand for more sophisticated models. By investing in R&D targeting chain-of-thought prompting, organizations can stay at the forefront of this trend.
- Focus on training models to better mimic human-like problem-solving: Successful AI technology needs to react and respond like a human, particularly when it comes to high-level problem-solving. Make human-like problem-solving a key focus in model training and development.
- Promote real-world applications of superior AI problem-solving skills: By showcasing the real-world benefits of more efficient and advanced problem-solving capabilities in AI, businesses can spur further investment and innovation in this field.