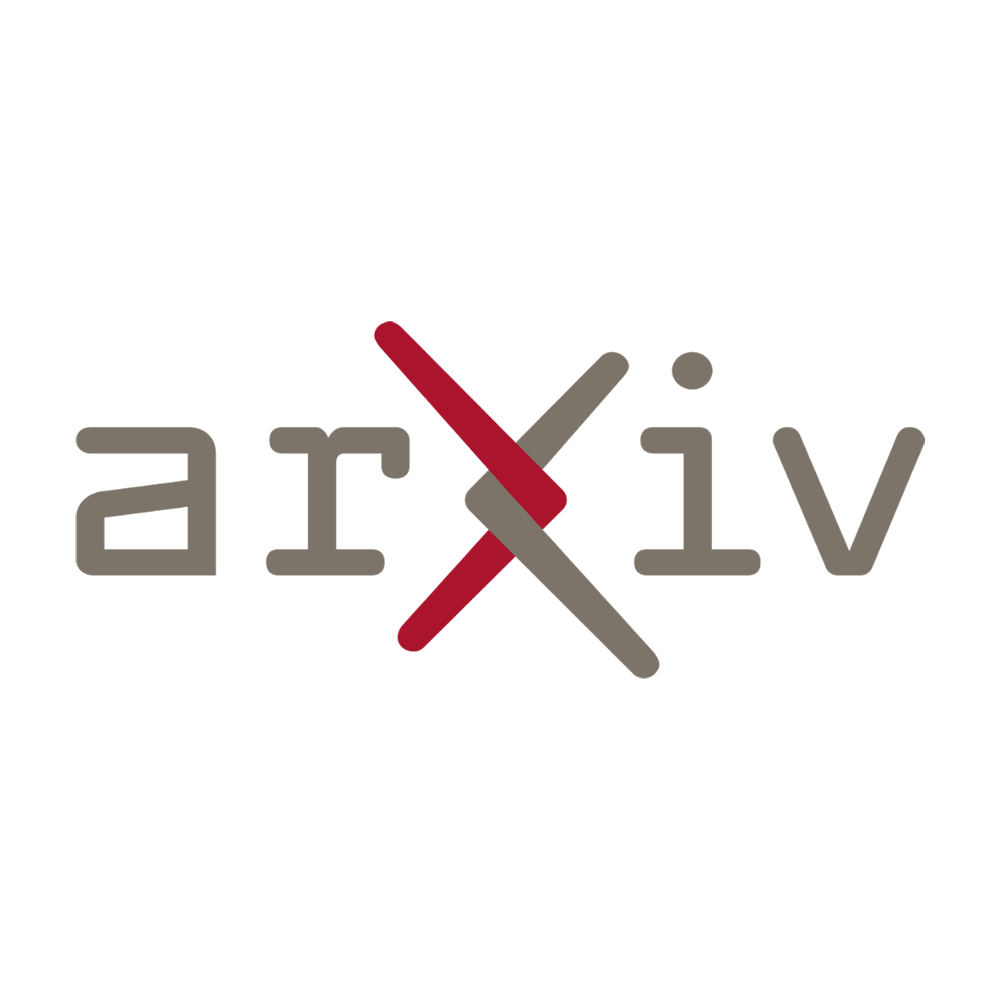
In the rapidly evolving field of digital watermarking, researchers have turned to deep learning techniques to enhance the imperceptibility and robustness of image watermarks. With the aim of striking a delicate balance between hiding watermarks within images and ensuring their durability against various attacks, current methods have been extensively explored. This article delves into the latest advancements in digital watermarking, shedding light on the innovative approaches that leverage deep learning to achieve optimal results. By examining the intersection of imperceptibility and robustness, this research holds significant implications for industries reliant on secure and tamper-proof digital content.
In recent years, digital watermarking techniques based on deep learning have been widely studied. Traditional approaches for image watermarking typically focus on achieving imperceptibility and robustness. However, these methods often fall short in terms of adaptability and efficiency. In this article, we propose a new approach to digital watermarking that addresses these limitations and brings about innovative solutions.
The Significance of Imperceptibility
Imperceptibility is crucial in digital watermarking as it ensures that the original image remains visually intact. Traditional methods rely on manipulating certain pixel values to embed the watermark, which often results in noticeable distortions. Our proposed approach takes advantage of deep learning algorithms to ensure a seamless integration of the watermark without compromising the visual quality of the image.
Innovative Deep Learning Techniques
Deep learning neural networks have revolutionized various domains, including computer vision. By leveraging the power of convolutional neural networks (CNNs) and generative adversarial networks (GANs), we can achieve remarkable imperceptibility in digital watermarking. These techniques allow us to embed watermarks by modifying specific hidden layers within the neural network, ensuring minimal disruption to the visible image.
Moreover, the use of GANs enables us to enhance the robustness of the watermark. GANs generate a synthetic, slightly distorted version of the original image, which acts as a reference for detecting any unauthorized modifications. By comparing the generated image with the watermarked image, we can determine if any tampering has occurred, providing an added layer of security.
Adaptability and Efficiency: The Missing Puzzle Pieces
Current digital watermarking methods often struggle with adaptability, meaning they are limited to specific types of images or formats. Our proposed approach overcomes this limitation by utilizing a flexible architecture that can handle various image types, resolutions, and formats. This adaptability allows us to apply our watermarking technique to diverse content, such as photographs, paintings, and illustrations, without compromising the overall quality.
Efficiency is another area where traditional methods fall short. Embedding watermarks using pixel manipulation techniques can be time-consuming, especially for large-scale applications. Our approach addresses this issue through parallel processing using Graphics Processing Units (GPUs). The use of GPUs significantly speeds up the watermark embedding process, making it more viable for real-time applications and large datasets.
Incorporating Blockchain for Enhanced Security
While imperceptibility and robustness are crucial, we acknowledge the importance of data integrity in digital watermarking. To enhance the security of our proposed approach, we suggest incorporating blockchain technology, which provides a decentralized and immutable ledger. By storing information about the watermark within a blockchain network, we can ensure that the authenticity and integrity of the watermark remain intact, even in the face of potential attacks or data manipulations.
Conclusion
By reimagining digital watermarking techniques based on deep learning, we have uncovered new possibilities for imperceptibility, adaptability, and efficiency. Our proposed approach leverages deep learning algorithms, such as CNNs and GANs, to achieve seamless watermark integration while maintaining the visual quality of the original image. Additionally, the incorporation of blockchain technology ensures data integrity and adds an extra layer of security.
As technology continues to advance, it is necessary to explore innovative solutions that push the boundaries of existing methodologies. With our proposed approach, we have taken a step towards revolutionizing digital watermarking, opening doors for applications in various fields, including copyright protection, content authentication, and digital forensics.
advanced deep learning architectures such as convolutional neural networks (CNNs) and generative adversarial networks (GANs). These techniques have shown great potential in addressing the challenges posed by traditional watermarking methods, which often struggle to maintain both imperceptibility (the watermark should not be visually noticeable) and robustness (the watermark should be resistant to various attacks).
One of the key advantages of using deep learning for digital watermarking is its ability to learn intricate patterns and features from large amounts of data. CNNs, for example, are well-suited for image watermarking as they can automatically extract relevant features from images, allowing for more effective hiding and detection of watermarks. This enables the development of more sophisticated and secure watermarking techniques.
Moreover, GANs have emerged as a powerful tool for watermarking due to their ability to generate realistic and high-quality image content. By training a GAN on a large dataset of watermarked and non-watermarked images, it can learn to generate visually appealing watermarked images that are difficult to distinguish from the original, non-watermarked ones. This helps to achieve imperceptibility, ensuring that the presence of a watermark does not significantly degrade the visual quality of the image.
In terms of robustness, deep learning-based watermarking methods also offer advantages. The use of complex architectures allows for the embedding of watermarks in a manner that is more resistant to common attacks, such as image compression, cropping, and noise addition. Deep learning models can learn to adapt to these attacks and still accurately detect and extract the watermark, ensuring robustness even in the face of malicious attempts to remove or alter the watermark.
Looking ahead, it is likely that research in this field will continue to focus on improving the imperceptibility and robustness of digital watermarking techniques. This could involve exploring novel deep learning architectures, optimizing the training process, or investigating new loss functions that better balance imperceptibility and robustness requirements.
Additionally, as deep learning methods become more advanced, there may be a shift towards exploring multi-modal watermarking, where watermarks are embedded not only in images but also in other types of media such as videos, audio, or 3D models. This would present new challenges and opportunities for researchers to develop innovative techniques that can effectively protect various forms of digital content.
Overall, the integration of deep learning techniques into digital watermarking has significantly advanced the field, providing more effective and secure methods for protecting intellectual property and verifying the authenticity of digital content. Continued research and development in this area hold great promise for the future of digital watermarking.
Read the original article