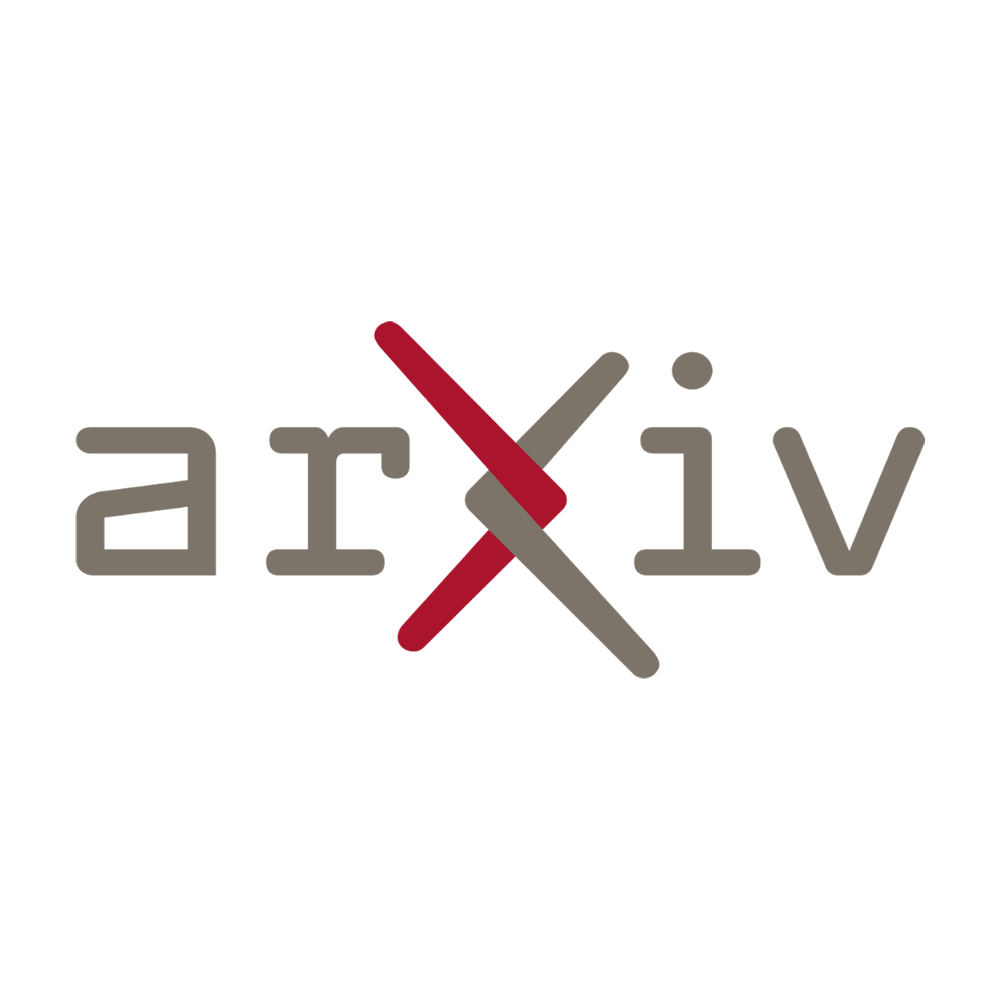
on automated metrics such as BLEU and METEOR, which primarily focus on linguistic similarity. However, a recent study by researchers from the University of California, Santa Cruz, proposes a novel evaluation metric called Caption-Metric, which aims to address these limitations. This metric takes into account not only the linguistic aspects of captions but also their visual quality, relevance, and diversity. By incorporating human judgments and leveraging state-of-the-art image captioning models, Caption-Metric provides a more comprehensive and accurate assessment of caption quality. This article explores the shortcomings of traditional evaluation metrics, the development of Caption-Metric, and its potential implications for advancing the field of caption generation.
Exploring the Unseen Dimensions: Evaluating the Full Quality and Fine-Grained Details of Captions
Despite significant advancements in caption generation, existing evaluation metrics often fail to capture the full quality or fine-grained details of captions. This is mainly due to their reliance on limited predefined criteria, which may not encompass the diverse aspects that make captions meaningful and engaging. In order to fully harness the potential of captions and enhance their quality assessment, we need to explore new dimensions and propose innovative solutions.
The Limitations of Existing Metrics
Existing evaluation metrics for captions typically focus on measuring basic aspects such as accuracy, fluency, and grammaticality. While these aspects are undoubtedly important, they only scratch the surface of what makes a caption truly valuable. Captions are not simply strings of words; they are a means of conveying information, emotions, and intentions. Therefore, it is crucial to widen the scope of evaluation metrics to capture these underlying dimensions.
Furthermore, existing metrics often fail to account for the fine-grained details that differentiate between captions of varying quality. For instance, two captions may have similar accuracy and fluency scores, but one may have a more creative and engaging choice of words, resulting in a more impactful and memorable caption. To fully appreciate and distinguish such nuances, we need to develop evaluation methods that delve deeper into the subtleties of captions.
Proposing Innovative Solutions
In order to overcome the limitations of existing metrics and advance the evaluation of captions, we propose several innovative solutions:
- Contextual Evaluation: Instead of evaluating captions in isolation, we should consider the context in which they are presented. Captions are often accompanied by visual content, such as images or videos, which can greatly influence their interpretation and impact. By incorporating the contextual elements into the evaluation process, we can gain a more comprehensive understanding of the caption’s quality.
- Semantic Analysis: Captions are not mere strings of words but convey semantic meaning. By employing natural language processing techniques, we can analyze the semantic structure of captions and assess how effectively they convey the intended message. This approach allows us to evaluate the richness and appropriateness of the language used in captions, going beyond surface-level metrics.
- Subjective Feedback: While objective metrics play a vital role, subjective feedback from human evaluators is equally crucial. Captions are subjective by nature, as they aim to evoke emotions and cater to different audiences. By incorporating human judgments, we can capture the subjective aspects of caption quality and obtain a more holistic evaluation.
- User Engagement Metrics: To understand the impact of captions on users, we can leverage user engagement metrics such as likes, comments, or sharing frequency. By analyzing how captions resonate with the audience and drive interaction, we can gain insights into their effectiveness and adjust our evaluation criteria accordingly.
Conclusion
The evaluation of captions is not a one-dimensional task. It requires a holistic approach that goes beyond accuracy and fluency measurements. By exploring new dimensions such as contextual evaluation, semantic analysis, subjective feedback, and user engagement metrics, we can develop innovative solutions to assess the full quality and fine-grained details of captions. These advancements will not only improve the evaluation metrics but also contribute to the development of more captivating and impactful captions that enhance the overall user experience.
on simple metrics such as BLEU (Bilingual Evaluation Understudy) that primarily focus on measuring the overlap between generated captions and reference captions. While BLEU and other similar metrics have been useful in evaluating the overall correctness of captions, they often fall short in capturing more nuanced aspects of caption quality, such as fluency, relevance, and coherence.
One of the major limitations of existing evaluation metrics is their inability to assess the semantic meaning and contextual understanding of captions. BLEU, for instance, primarily relies on n-gram matching to measure the similarity between generated and reference captions. However, this approach fails to consider the semantic meaning of words and the overall coherence of the generated text. As a result, captions that may have different wording but convey the same meaning are penalized, while captions with similar words but different meanings are rewarded.
To overcome these limitations, recent research has focused on developing more sophisticated evaluation metrics that can better capture the quality and nuances of captions. For example, metrics like CIDEr (Consensus-based Image Description Evaluation) and METEOR (Metric for Evaluation of Translation with Explicit ORdering) have been proposed to address the shortcomings of BLEU. These metrics take into account not only the lexical similarity but also the semantic similarity and sentence structure, providing a more comprehensive evaluation of caption quality.
Furthermore, advancements in natural language processing and machine learning have paved the way for the development of more context-aware evaluation metrics. These metrics aim to assess the quality of captions by considering the context in which they are generated. For instance, metrics like SPICE (Semantic Propositional Image Caption Evaluation) and ROUGE (Recall-Oriented Understudy for Gisting Evaluation) incorporate semantic analysis and understanding of captions to evaluate their quality within the context of the image.
Looking ahead, the future of caption evaluation lies in the development of even more sophisticated and context-aware metrics. As deep learning models continue to improve, there is a possibility of leveraging neural networks to develop evaluation metrics that can better capture the fine-grained details of captions. By incorporating contextual information, semantic understanding, and even user feedback, these metrics could provide a more comprehensive and accurate assessment of caption quality.
Moreover, the field of caption evaluation could benefit from the establishment of standardized datasets and benchmarks. Currently, there is a lack of consensus on what constitutes a high-quality caption, and different evaluation metrics may produce conflicting results. Establishing benchmark datasets with diverse images and captions, along with well-defined evaluation criteria, would enable researchers to compare and evaluate different caption generation models more effectively.
In conclusion, while existing evaluation metrics have played a crucial role in assessing the quality of generated captions, they often fail to capture the full richness and nuances of captions. The future of caption evaluation lies in the development of more sophisticated metrics that consider semantic meaning, context, and user feedback. By addressing these challenges, we can expect to see improved evaluation techniques that will drive advancements in caption generation and enhance the quality of automated image captioning systems.
Read the original article