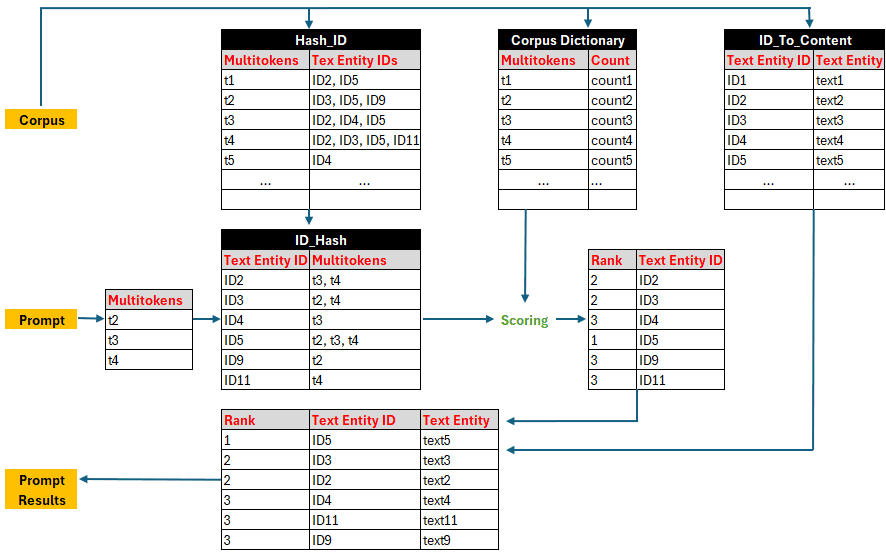
Introduction
The increasing prevalence and integration of Artificial Intelligence (AI) in various fields has brought about numerous advancements, methodologies, and challenges. Some key points of significance include RAG (Retrieval Augmented Generation), LLM (Large Language Models), Generative AI, Knowledge Graph, Chunking, Statistical AI, and AI Optimization. Each has a distinct impact and potential on AI’s future trajectory.
Understanding Key Concepts
RAG, LLM, and Generative AI
RAG combines retrieval-based models with sequence-to-sequence Transformers in a novel way, enhancing the effectiveness of AI. LLMs, on the other hand, have the capacity to understand and produce human language at an unprecedented scale, while Generative AI models can learn to generate data resembling a given dataset, improving innovation and efficiency.
Knowledge Graph, Chunking, Statistical AI
Knowledge Graphs are a way to structure and interlink all knowledge and data, providing artificial systems with a distillation of human understanding. Chunking enhances data processing by splitting inputs into more manageable pieces, thereby improving AI computation speed. Meanwhile, Statistical AI utilizes algorithms that learn from vast data sets, making AI systems more intelligent and reliable.
AI Optimization
This is the practice of improving AI metrics by fine-tuning model’s parameters or architecture, often using large datasets and compute power. Optimization is a critical component in achieving high-performing AI systems.
Long-term Implications
AI’s progress toward a more intelligent and reliable future is closely tied to advancements in these key areas. Considering their integrated nature, their joint improvement is likely to expedite AI’s evolution significantly.
Possible Future Developments
The future of AI points towards a substantial improvement in efficiency, innovative capabilities, reliability, and the ability to handle enormous sets of data. This will cultivate a more interconnected, data-rich world where AI is seamlessly integrated into daily life.
- RAG can greatly enhance AI’s learning capabilities, potentially leading to the development of more reliable, intelligent, and efficient machine learning systems.
- LLM promises a future where AI understands and produces human-like text, paving the way for more sophisticated AI-human interaction.
- Generative AI can ensure a more inventive and creative AI, giving birth to innovative solutions for both existing and unforeseen challenges.
- Knowledge Graphs are poised to create an interconnected web of knowledge, analogous to human understanding.
- Chunking will quicken data processing, enriching machine learning algorithms and making AI systems more robust.
- Statistical AI will offer more reliable and accurate predictions, fostering trust in AI systems.
- AI Optimization, the catalyst for turning theoretical AI into practical solutions, will continue to advance, ensuring consistently high-performing AIs.
Actionable Advice
- Embrace the changes – AI is poised for enormous growth and the key is to adapt and evolve with it.
- Invest in learning and understanding these key concepts – they lay the foundation for the AI of the future.
- Stay current with AI advancements – this is essential in the rapidly growing AI field.
- Continue to foster trust in AI – With advancements in AI reliability, maintaining public trust should be a priority.