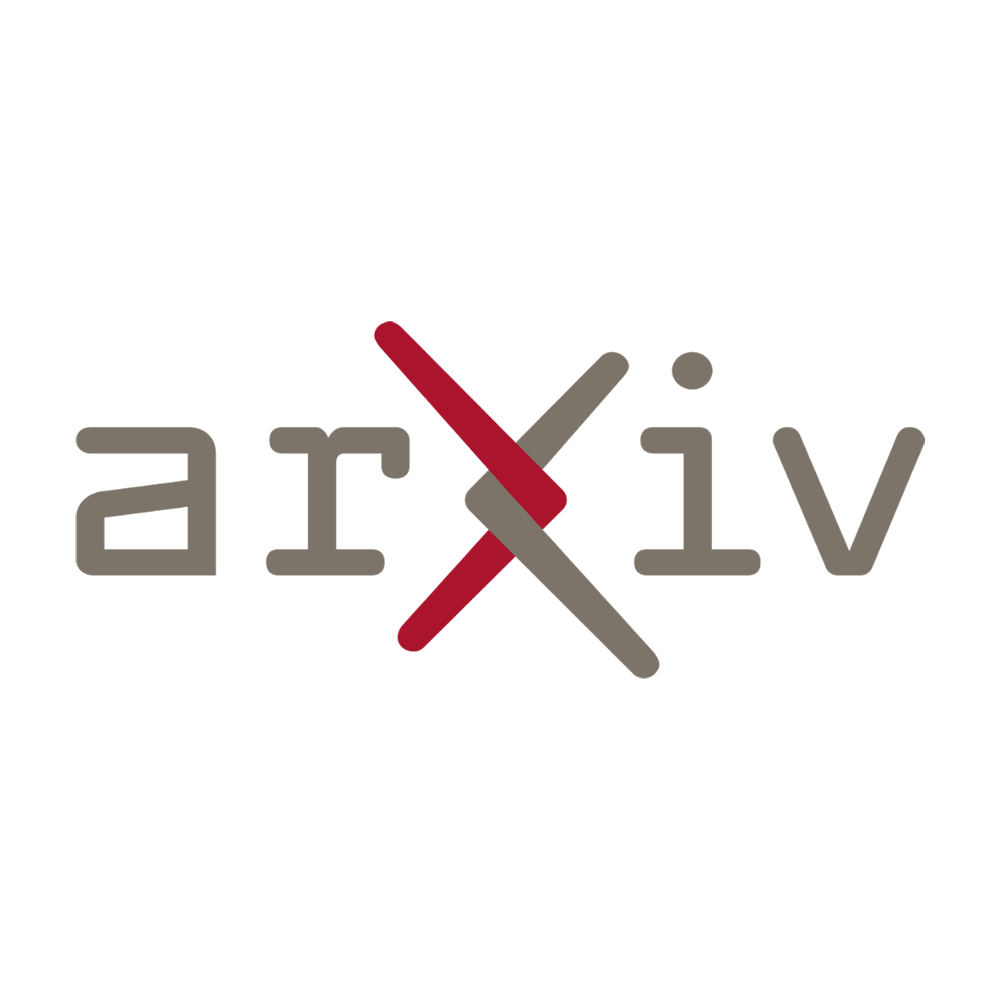
In today’s digital age, the unauthorized use of text in images has become a widespread concern. To combat this issue, a revolutionary technique called Scene Text Removal (STR) has emerged as a crucial task. STR aims to automatically remove text from images and replace it with a seamless, text-less background, ensuring the integrity and privacy of visual content. This article delves into the core themes of STR, exploring its significance in preventing unauthorized use of text in images and highlighting its ability to restore images to their natural, text-free state.
Exploring Innovative Solutions and Ideas in Scene Text Removal (STR)
In today’s digital age, the presence of text in images has become ubiquitous. From advertisements to social media posts, text is an integral part of our visual culture. However, there are instances where the presence of text may be unwanted or burdensome, such as when manipulating images or creating a text-less background for aesthetic or privacy purposes. This is where Scene Text Removal (STR) comes into play.
The Crucial Task of Scene Text Removal
Scene Text Removal (STR) is a computational task that aims to automatically detect and remove text from images, replacing it with a natural, text-less background. Whether it is removing captions from images for further analysis or eliminating text for enhancing image aesthetics, STR has become an essential tool in various fields, including computer vision, image editing, and content moderation.
Understanding the Underlying Themes and Concepts
At its core, STR involves two fundamental themes: text detection and text inpainting. Text detection focuses on identifying and localizing text within an image, while text inpainting deals with replacing the detected text regions with meaningful visual content that blends seamlessly with the surrounding background.
Proposing Innovative Solutions for Scene Text Removal
As the field of STR evolves, researchers and developers continually propose innovative solutions to enhance the accuracy and efficiency of the techniques involved. One such idea is the integration of deep learning algorithms, specifically Convolutional Neural Networks (CNNs), for text detection and inpainting tasks.
Deep Learning and Text Detection
Deep learning models, particularly CNNs, have demonstrated remarkable performance in text detection tasks. By training CNNs on large datasets containing labeled images with and without text, these models can learn to differentiate between text and non-text regions, achieving impressive accuracy in identifying text within images.
Enhancing Text Inpainting with Generative Adversarial Networks (GANs)
In the realm of text inpainting, Generative Adversarial Networks (GANs) have shown promising results. GANs consist of two components: a generator network, responsible for creating plausible inpainting proposals, and a discriminator network, which evaluates the quality of the generated proposals.
By training GANs on paired datasets, consisting of images with text and their corresponding text-less versions, the generator network can learn to generate realistic inpainting proposals that seamlessly replace the text regions. Meanwhile, the discriminator network helps improve the realism and coherence of the generated proposals by providing feedback during the training process. This approach has the potential to create highly convincing text-free backgrounds while preserving the overall image context.
Conclusion
As Scene Text Removal (STR) becomes increasingly important in our digital landscape, innovative solutions like deep learning algorithms and GANs offer promising avenues for enhancing the accuracy and efficiency of text detection and inpainting tasks. These advancements open up new possibilities for both researchers and practitioners in various fields, enabling them to unlock the full potential of text removal and accompanying image manipulation techniques. By pushing the boundaries of STR, we can harness the power of visual content while seamlessly integrating it into our ever-evolving digital world.
Scene Text Removal (STR) is indeed a critical task in the field of computer vision, as it addresses the challenge of removing text from images. With the increasing prevalence of text in images, such as street signs, billboards, and captions, the need for automated text removal techniques has become paramount.
The primary objective of STR is to automatically detect and remove text while preserving the underlying content and context of the image. This task involves several complex steps, including text detection, character recognition, and inpainting.
Text detection algorithms play a crucial role in identifying the regions of an image that contain text. These algorithms utilize various techniques, such as edge detection, connected component analysis, and machine learning-based approaches, to accurately locate and segment text regions.
Once the text regions are identified, character recognition methods are employed to extract the textual content. Optical Character Recognition (OCR) techniques have made significant advancements in recent years, enabling accurate text extraction even in challenging scenarios involving complex fonts, distorted text, or low-resolution images.
After the text is recognized, the next step is to replace it with a text-less background seamlessly. This process, known as inpainting, aims to fill the void left by the removed text with plausible content that matches the surrounding context. Inpainting techniques leverage image synthesis and texture completion methods to generate visually coherent backgrounds.
Despite the advancements in STR, there are still several challenges that need to be addressed. One major hurdle is the removal of text from complex backgrounds, such as textures, patterns, or cluttered scenes. Text that overlaps with important objects or has similar colors to the background poses additional difficulties.
To overcome these challenges, researchers are exploring deep learning-based approaches, which have shown promising results in recent years. Convolutional Neural Networks (CNNs) and Generative Adversarial Networks (GANs) have demonstrated their effectiveness in text removal tasks by learning complex visual patterns and generating realistic background textures.
Looking ahead, we can expect further improvements in STR techniques driven by advancements in deep learning architectures, larger annotated datasets, and the integration of contextual information. Additionally, the development of real-time STR algorithms will be crucial for applications such as video editing, surveillance, and augmented reality.
Furthermore, the application of STR extends beyond text removal. It can also be utilized for text manipulation, where text is modified or replaced with different content, opening up possibilities for content editing, language translation, and image enhancement.
In conclusion, Scene Text Removal is an evolving field with immense potential. As technology progresses, we can anticipate more accurate and efficient STR algorithms that will enhance our ability to automatically remove text from images while preserving the visual integrity and context of the underlying content.
Read the original article