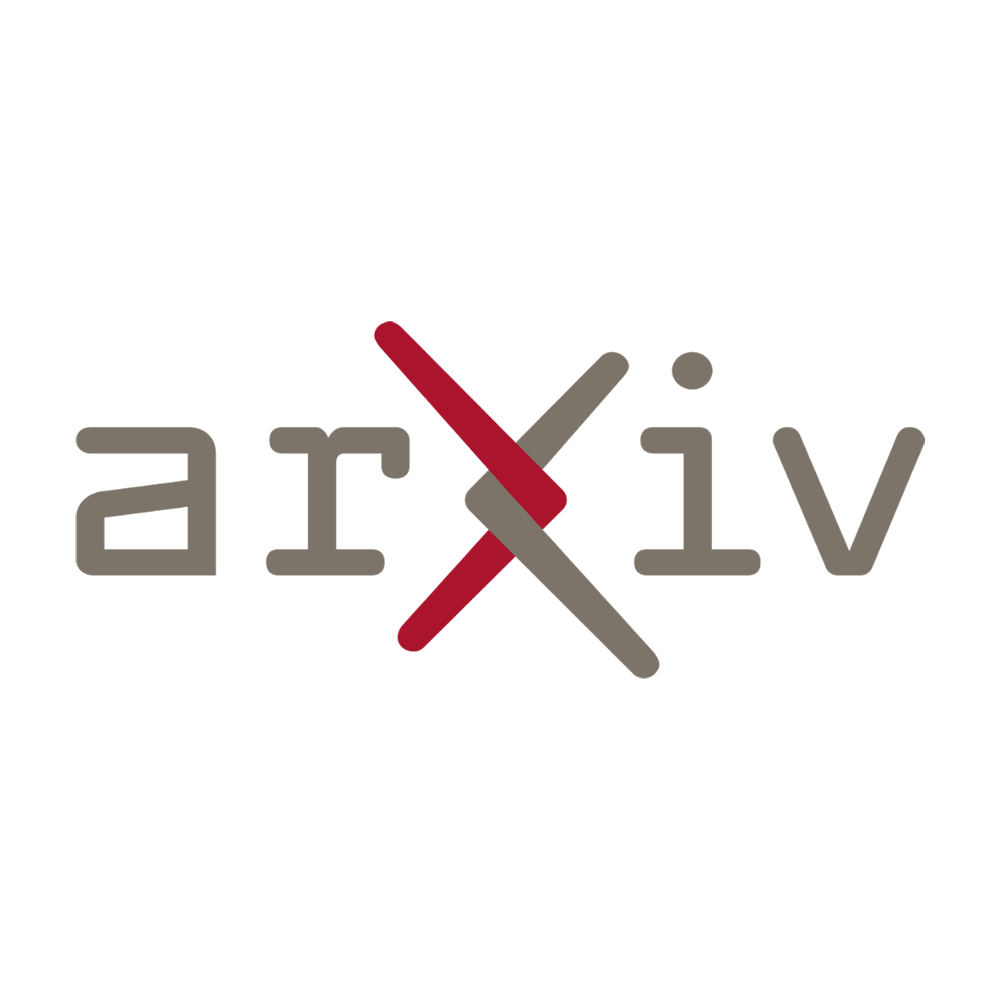
Machine unlearning (MU) has emerged as an innovative technique to selectively erase certain data from trained models, eliminating the need for extensive retraining. This article explores the advancements and challenges in the field of MU, highlighting its potential to enhance privacy, mitigate bias, and improve model performance. Despite notable progress, researchers are still grappling with the complexities of MU, including the identification of relevant data, the development of efficient unlearning algorithms, and the potential impact on model interpretability. By delving into these core themes, this article sheds light on the promising future of machine unlearning and its implications for the evolving landscape of artificial intelligence.
Machine unlearning (MU) has gained significant attention as a means to remove specific data from trained models without requiring a full retraining process. While progress has been made in developing MU techniques, there are underlying themes and concepts that deserve exploration in a new light, accompanied by innovative solutions and ideas.
The Ethical Dimension
One of the underlying themes in machine unlearning is the ethical dimension. As AI becomes more integrated into our lives, it is crucial to consider the impact of biased or erroneous data on trained models. MU presents an opportunity to rectify these issues by selectively removing problematic data points. However, the ethical responsibility falls on developers to ensure a fair and unbiased process of unlearning.
To address this, innovative solutions can be implemented that require developers to thoroughly analyze the removed data and question its potential biases. An algorithm could be designed to identify patterns of discrimination or misinformation within the data, flagging them for human review. This human oversight would ensure that the unlearning process aligns with ethical guidelines and promotes fairness.
Privacy and Data Protection
Another crucial theme in machine unlearning is privacy and data protection. As we entrust AI systems with more personal information, the ability to selectively unlearn sensitive data becomes imperative. MU provides a solution by allowing the removal of individual data points, enabling a balance between retaining model accuracy and safeguarding privacy.
Innovative ideas for data protection in MU could involve a combination of encryption techniques and differential privacy. Encrypted machine unlearning would allow for secure removal of specific data points without compromising privacy. Additionally, integrating differential privacy mechanisms during unlearning would add an extra layer of protection by ensuring that individual data points cannot be re-identified.
Dynamic and Continual Learning
Machine unlearning also raises the concept of dynamic and continual learning. Traditional machine learning models are trained on static datasets, limiting their ability to adapt and evolve as new data emerges. MU opens up possibilities for incorporating continual learning methodologies, allowing models to unlearn outdated or irrelevant data on the fly.
An innovative solution in this realm could be the development of an adaptive unlearning framework. This framework would analyze the relevance and accuracy of data over time, enabling continuous model refinement through targeted unlearning. By unlearning outdated data and focusing on recent and more relevant information, models can better adapt to changing circumstances and improve their performance in real-world applications.
Conclusion: Machine unlearning is an emerging field that presents exciting opportunities for improving the fairness, privacy, and adaptability of AI systems. By exploring the ethical dimension, prioritizing privacy and data protection, and incorporating dynamic learning methodologies, we can unlock the true potential of machine unlearning. As developers and researchers delve further into this field, it is paramount to consider these underlying themes and concepts, constantly innovating and iterating on our approaches to create responsible, robust, and continually improving AI systems.
the field of machine learning, there are still challenges to overcome in the area of machine unlearning. The ability to selectively remove specific data from trained models is crucial for addressing privacy concerns, ensuring regulatory compliance, and handling biases that may have been unintentionally learned by the model.
One of the key advancements in machine unlearning is the development of algorithms that can identify and remove specific instances or patterns from the trained model without the need for retraining. This is particularly important in situations where certain data points or attributes need to be forgotten due to legal or ethical reasons. For example, in the case of personal data that should no longer be stored or used, machine unlearning can help ensure compliance with privacy regulations such as the General Data Protection Regulation (GDPR).
Another area where machine unlearning can be beneficial is in addressing biases that may exist within trained models. Biases can arise from the data used for training, reflecting societal prejudices or unequal representation. With machine unlearning, problematic biases can be identified and selectively removed, allowing for fairer and more unbiased decision-making processes.
However, there are several challenges that need to be addressed for machine unlearning to be widely adopted. One challenge is the lack of standardized techniques and frameworks for machine unlearning. As of now, there is no widely accepted approach or set of guidelines for implementing machine unlearning in different scenarios. This makes it difficult for researchers and practitioners to compare and replicate results, hindering the progress in this field.
Another challenge is the potential loss of performance or accuracy when removing specific data from trained models. Removing certain instances or patterns may lead to a decrease in the model’s overall performance, as the removed data might have contributed to the model’s ability to generalize and make accurate predictions. Balancing the removal of unwanted data with the preservation of model performance is a complex task that requires further research and development.
Looking ahead, the future of machine unlearning holds promise. As the field matures, we can expect to see the emergence of standardized techniques and frameworks, enabling more consistent and reliable machine unlearning processes. Additionally, advancements in explainable AI and interpretability will play a crucial role in understanding the impact of data removal on model behavior and performance.
Furthermore, the integration of machine unlearning within larger machine learning pipelines and frameworks will be essential. This will require seamless integration with existing model training and deployment processes, ensuring that machine unlearning becomes an integral part of the machine learning lifecycle.
In conclusion, machine unlearning has gained attention for its potential to selectively remove specific data from trained models. While progress has been made, challenges remain, such as the lack of standardized techniques and the potential loss of performance. However, with further research and development, machine unlearning has the potential to enhance privacy, address biases, and improve the overall fairness and transparency of machine learning systems.
Read the original article