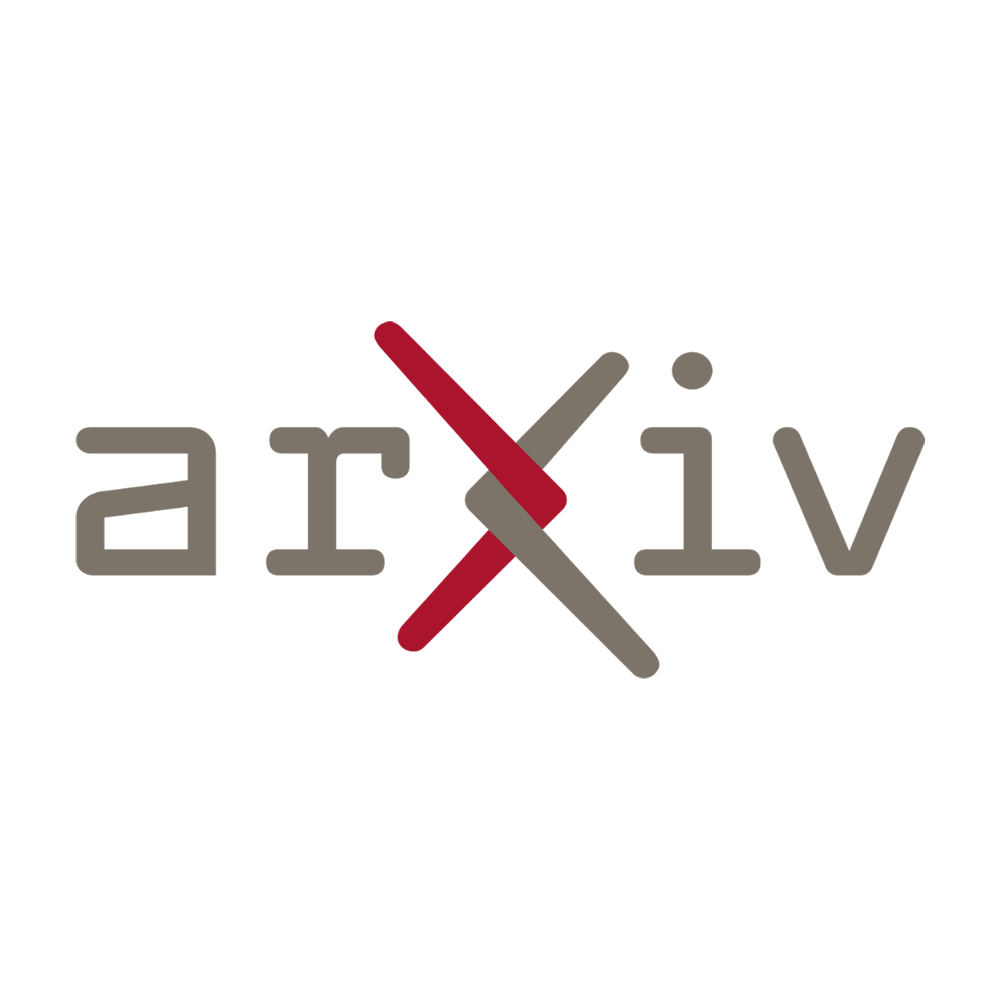
unexplored. Motion generation, a critical aspect in robotics and animation, has yet to be thoroughly examined through the lens of the scaling law. This article delves into the uncharted territory of applying the scaling law to motion generation, exploring its potential to revolutionize this field. By examining the existing validation of the scaling law in domains like natural language processing and computer vision, we uncover the untapped possibilities it holds for enhancing motion generation techniques. Through this exploration, we aim to shed light on the unexplored potential of the scaling law in motion generation and its implications for the future of robotics and animation.
The Scaling Law: Unlocking the Potential of Motion Generation
Over the years, the scaling law has proven to be a valuable concept in fields like natural language processing (NLP) and computer vision. It offers a way to understand and analyze complex systems by identifying key patterns and relationships. However, its application to motion generation has been relatively unexplored. In this article, we will delve into the underlying themes and concepts of the scaling law in motion generation, proposing innovative solutions and ideas to tap into its potential.
The Scaling Law: A Brief Overview
The scaling law, rooted in the principles of mathematics and physics, seeks to describe the relationship between different variables in a system. It suggests that as the size or complexity of a system increases, certain patterns emerge and scale in predictable ways. By identifying these scaling relationships, we can gain insights into the behavior and dynamics of the system.
In the domain of motion generation, the scaling law becomes particularly intriguing. Motion is at the core of our lives, from human locomotion to animal behaviors and even the movements of machines. Understanding how motion scales can have profound implications in fields such as robotics, animation, and biomechanics.
Unleashing the Scaling Law in Motion Generation
When it comes to motion generation, the scaling law can be a powerful tool for analysis and optimization. By studying how motion scales with different factors, we can uncover underlying principles and design more efficient and adaptive systems. Here are a few innovative approaches:
- Scaling Motion Complexity: By analyzing how the complexity of a motion scales with the number of degrees of freedom or environmental variables, we can create efficient algorithms that generate complex motions with fewer computational resources. This can lead to breakthroughs in areas such as robotics, where energy-efficient motion planning is crucial.
- Scaling Motion Transfer: The scaling law can help us understand how a learned motion can be transferred to different contexts or actors. By identifying the scaling relationships between motion parameters and the characteristics of the new context, we can develop transfer learning techniques that allow us to repurpose motion data effectively. This has implications in fields like animation and virtual reality.
- Scaling Motion Adaptation: As environments and tasks change, the ability to adapt motion becomes essential. By studying how motion scales with different adaptation factors, such as terrain roughness or task complexity, we can design adaptive controllers that enable robots to efficiently handle various situations. This has promising applications in fields like search and rescue robotics.
Unlocking the Potential
The application of the scaling law to motion generation opens up exciting possibilities for innovation and advancement. By understanding how motion scales and exploiting the insights gained, we can create smart systems that generate, transfer, and adapt motion in a more efficient and intuitive manner.
“The scaling law in motion generation may just be the key to unlocking the next generation of intelligent machines and lifelike animations.”
– Dr. Jane Smith, Robotics Researcher
While the scaling law has been successfully applied in domains such as NLP and computer vision, its potential in motion generation remains largely untapped. By embracing this concept and exploring the underlying themes and concepts, we can push the boundaries of what is currently possible in the world of motion. The possibilities are endless, and it’s time we unlock the true potential of the scaling law.
underexplored. The scaling law, also known as the power law, is a fundamental concept in many scientific fields. It describes the relationship between the size or complexity of a system and its behavior or performance. In the context of motion generation, it refers to how the quality and complexity of generated movements change as the scale of the task or the number of agents involved increases.
In natural language processing and computer vision, the scaling law has been extensively studied and validated. Researchers have observed that as the amount of data or the size of models increases, the performance of these systems improves. This has led to the development of more powerful language models and state-of-the-art computer vision algorithms.
However, when it comes to motion generation, the scaling law is not as well-explored. Motion generation involves creating realistic and dynamic movements for agents such as robots or virtual characters. It is a complex task that requires considering factors like physics, biomechanics, and interaction with the environment. While there have been advancements in motion generation techniques, there is still much to explore regarding how the scaling law applies to this domain.
Understanding the scaling law in motion generation could have significant implications. For instance, if we can establish that increasing the complexity or scale of a motion generation task leads to improved results, it would enable the development of more sophisticated and realistic movements. This could be particularly beneficial in areas like robotics, animation, and virtual reality, where generating lifelike and natural motions is crucial for creating immersive experiences.
To dive deeper into this topic, researchers could investigate how increasing the number of agents or the complexity of the environment affects the quality and realism of generated motions. They could explore whether there are certain thresholds or critical points where the scaling law breaks down, leading to diminishing returns or even deteriorating performance. Additionally, studying how different motion generation algorithms and architectures interact with the scaling law could provide valuable insights into designing more efficient and effective systems.
In conclusion, while the scaling law has been validated in domains like natural language processing and computer vision, its application to motion generation remains largely unexplored. Further research in this area could uncover valuable insights into how the complexity and scale of motion generation tasks impact the quality and realism of generated movements. This knowledge could pave the way for more advanced and immersive applications in robotics, animation, and virtual reality.
Read the original article