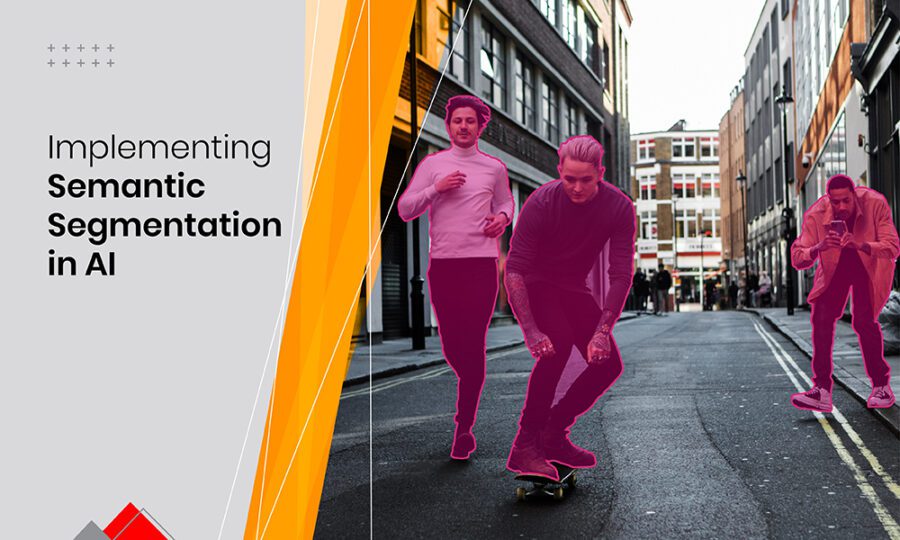
Long-term Implications and Future Developments in AI Semantic Segmentation
Semantic segmentation, an essential component of the artificial intelligence (AI) pipeline, offers promising potential for numerous applications. The following outlines an analytical perspective on the long-term implications, future developments, and practical advice concerning semantic segmentation within the AI pipeline.
Long-term Implications
As AI technology evolves, semantic segmentation will progressively become a crucial element. With its capacity to understand and interpret images at the pixel level, applications of this tool in industries such as autonomous driving, healthcare, and surveillance are paramount.
For instance, in autonomous driving, semantic segmentation can be applied to process real-time images and distinguish between different objects like pedestrians, other vehicles, and structures, vastly improving safety and operational efficiency.
In healthcare, semantic segmentation can aid in precise medical imaging analysis, facilitating better treatment plans, diagnostics, and monitoring. For surveillance, it can assist in monitoring activities, identifying anomalies, and potentially predicting threatening situations before they occur.
Future Developments
As we move towards the future, the demands for refined semantic segmentation models that outdo limitations such as inadequate generalization capabilities and overfitting are expected to increase. We can also foresee continuous development in data annotation techniques essential for training these models, and strategies for integrating these models into broader AI systems.
Actionable Advice
- Invest time in quality data annotation: An accurate, comprehensive annotation is a crucial starting point for any semantic segmentation project. Subsequently, investing time and resources in this step will directly influence the success of the project.
- Keep abreast with the latest tools and techniques: The AI field is continually evolving. Stay updated with the latest advancements concerning semantic segmentation.
- Fine-tune models continuously: Regularly evaluate the performance of your models to avoid overfitting. Keep refining your model to enhance its generalization capabilities.
- Integration is key: Ensure to develop a clear strategy on how to integrate the semantic segmentation model into your existing AI system for the smooth functioning of the entire pipeline.
In conclusion, rendering the complexity of semantic segmentation into a structured, manageable process marks a significant step forward in effectively incorporating this powerful tool into the AI pipeline. The future holds vast possibilities for advancements in each step from data annotation to model integration, unlocking an array of potential for businesses and industries worldwide.