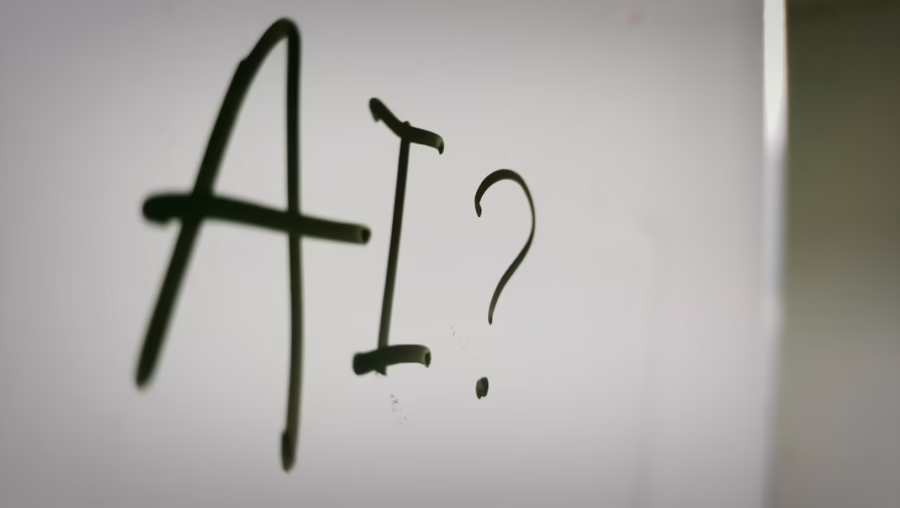
Generative AI & QA: Long-term implications and Future Possibilities
The ever-evolving and accelerated software development cycles present daunting challenges for quality assurance (QA) teams worldwide. Engineering leaders grapple with these demands by continuously seeking to maintain quality while juggling the speed and complexity of modern software superhighways. The increasing demands of the users and the fast-paced industry changes necessitate a shift from traditional QA strategies. A potential game-changer in this scenario is generative AI, which is reshaping traditional QA, offering a new dimension of possibilities.
Long-term Implications of Generative AI on QA
All indicators point towards significant implications of generative AI on existing QA strategies as we see them today. A few such key transformations to anticipate include the following:
- Reduction in QA cycle time: Generative AI has the potential to significantly decrease the time spent on QA by automatically generating test data, identifying blind spots and streamlining the entire testing process.
- Better product quality: With automating QA processes, the chance for human errors decreases substantially, leading to overall product quality enhancement.
- Escalation of efficiency: Efficient use of resources can lead to cost savings and increased productivity, as repetitive and mundane tasks can be automated, allowing the QA team to focus on more complex aspects of quality checks.
Possible Future Developments
The use of generative AI in QA has just started touching the surface, and its full potential is yet to be unlocked. Here are some potential future developments we might witness:
- Advanced Machine Learning: AI stands to become more intelligent and intuitive as advancements in machine learning set in, reshaping the way we understand software testing.
- Real-time QA: The future might see AI perform testing in real-time, ensuring consistent product quality and timely detection of any flaws.
- AI-driven Risk Assessment: AI could develop capabilities to assess and predict risks based on data patterns, outperforming traditional manual QA methods.
Actionable Advice
“Change is the only constant”
This timeless saying holds true especially in the context of software development and QA. It’s vital to not just acknowledge this change but to actively adapt to the new realities. Here are some practical steps that can be taken:
- Embrace AI: It is important to welcome the power of AI and start incorporating it into the workflow. Initial steps may look like leveraging AI tools for automating repetitive tasks.
- Invest in Training: As technology evolves, so too must the team’s skills. Training the team to understand and optimally utilize AI will become indispensable.
- Virtualization and Cloud: Ensure the use of virtualization and cloud technology to fully exploit the potential benefits of AI in QA.
By proactively incorporating these anticipatory strategies, QA teams can reshape traditional tactics to accommodate the power of generative AI and ultimately realize enhanced software reliability and effectiveness.