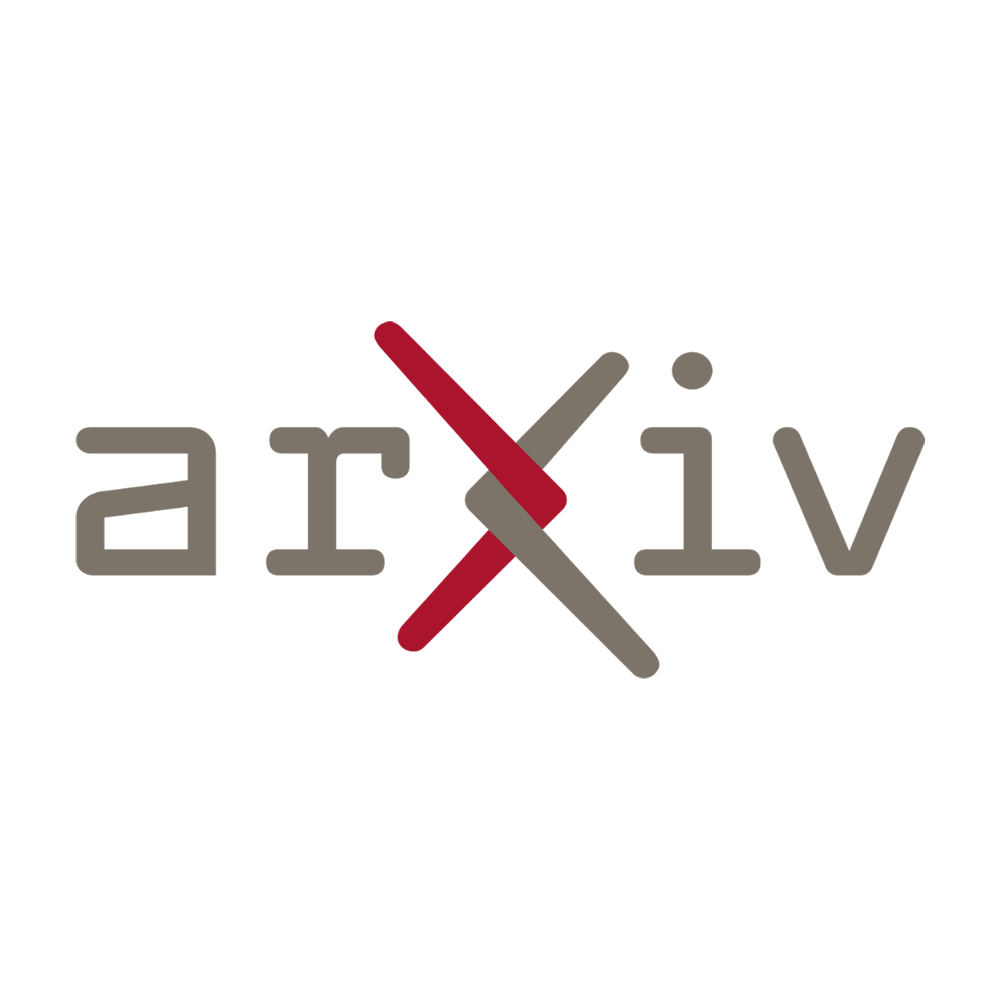
allowing for the integration of both text and image inputs. This article explores the potential of combining large language models with multimodal capabilities to enhance few-shot in-context learning. By leveraging the power of these models, researchers have achieved remarkable results in various tasks, such as image captioning and visual question answering. The article highlights the significance of these advancements and their implications for improving the understanding and generation of multimodal content. Additionally, it discusses the challenges and future directions in this field, paving the way for further exploration and innovation in multimodal few-shot learning.
Large language models have revolutionized the field of natural language processing, enabling impressive capabilities such as few-shot in-context learning (ICL). This approach allows models to quickly adapt and understand new information given a small amount of context. However, the advancements in multimodal foundation models have paved the way for even greater opportunities. These models, which incorporate both text and visual information, offer unparalleled possibilities for long context windows.
Expanding Context with Multimodal Models
In traditional language models, the context window is limited to a certain number of preceding and succeeding tokens. This constraint, while effective for many tasks, restricts the overall context available to the model. With the introduction of multimodal models, we can extend this context beyond just textual information.
By incorporating visual data alongside text, multimodal models allow for a more comprehensive understanding of the context. For example, when processing a news article about a scientific discovery, the model can analyze both the written content and any accompanying images or charts to gain a deeper understanding of the topic. This expanded context window enhances the model’s ability to generate accurate and contextual responses, opening new avenues for innovation.
Potential Applications
The possibilities presented by multimodal models extend across various domains and industries. Here are a few areas where these models can have a significant impact:
- Visual Question Answering: Multimodal models can excel in tasks that require image understanding and language processing. For instance, imagine a virtual assistant that can answer questions about an image like “What breed of dog is in this picture?” or “Where was this photo taken?” This integration of visual and textual understanding enhances the overall user experience.
- Social Media Analysis: With multimodal models, analyzing social media posts becomes more insightful. By considering both the text and visual content of a post, models can better understand sentiment, detect misinformation, or even identify potential trends in images shared across social platforms.
- Customer Support: Multimodal models can greatly enhance customer support systems. By analyzing both text-based queries and any accompanying images or screenshots, these models can provide more accurate and tailored responses to specific user issues. This can help alleviate customer frustration and improve overall satisfaction.
Challenges and Considerations
While multimodal models have immense potential, there are several challenges to overcome:
- Data Bias: Models trained on multimodal datasets may inherit biases present in the data, particularly when it comes to interpreting visual content. It is crucial to address these biases and ensure fair representation and equal understanding across different demographic groups.
- Training Complexity: Incorporating visual information alongside text adds another layer of complexity to model training. Ensuring efficient fine-tuning and optimization processes becomes crucial to maintain the model’s performance.
- Data Privacy: Handling multimodal data, which often includes personal images or sensitive information, requires robust privacy measures to protect user data and maintain trust.
Conclusion
The advancements in multimodal foundation models have opened up exciting opportunities for expanding the context window in few-shot in-context learning. By incorporating visual data alongside text, these models offer a more comprehensive understanding of the context, presenting numerous applications across various domains. However, challenges such as data bias, training complexity, and data privacy need to be carefully addressed. With innovative solutions and responsible practices, multimodal models can unleash their full potential and pave the way for even more powerful AI systems.
“Multimodal models offer a revolutionary approach to few-shot in-context learning, utilizing the power of visual data to expand the context and enhance understanding.” – [Your Name]
allowing for even more effective few-shot in-context learning. These large language models, combined with multimodal capabilities, have the potential to revolutionize various fields such as natural language understanding, computer vision, and even robotics.
One of the key advantages of large language models is their ability to learn from just a few examples within a specific context. This few-shot learning ability is particularly valuable in situations where there is limited labeled data available. By leveraging the vast amount of pre-training data, these models can quickly adapt and generalize to new tasks or domains.
With the recent advancements in multimodal foundation models, which can process both textual and visual information, we can now incorporate visual context into the learning process. This opens up new possibilities for applications that require understanding and generating text in the context of images or videos. For example, an AI system could generate detailed descriptions of images or perform complex image-based reasoning tasks by utilizing both textual and visual information.
The ability to handle long context windows is another significant stride in few-shot in-context learning. Traditional language models typically have limitations on the amount of context they can effectively process. However, recent advancements have pushed these boundaries, allowing models to consider much longer sequences of text and capture more nuanced dependencies. This enhanced context understanding can lead to better comprehension, more accurate predictions, and improved contextual generation.
Looking ahead, we can expect even more progress in few-shot in-context learning. As researchers continue to refine and optimize large language models, we may witness breakthroughs in leveraging multimodal data with greater efficacy. We might see models that can understand and generate complex narratives from both textual and visual cues, leading to advancements in storytelling, content generation, and even interactive virtual experiences.
Furthermore, the integration of multimodal few-shot learning with robotics holds immense potential. Robots equipped with language models capable of understanding and generating natural language in various contexts could become more adaptable and versatile. They could quickly learn new tasks or adapt to changing environments, making them more useful and intelligent in real-world scenarios.
However, there are also challenges to overcome. Large language models require significant computational resources, which can limit their accessibility and scalability. Additionally, ethical considerations surrounding the use of these models, such as biases and potential misuse, need careful attention and mitigation.
In conclusion, recent advancements in multimodal foundation models and the ability to process long context windows have propelled few-shot in-context learning to new heights. The combination of large language models and multimodal capabilities has the potential to revolutionize various domains, from natural language understanding to computer vision and robotics. As researchers continue to push the boundaries of these models, we can anticipate exciting developments and applications in the future.
Read the original article