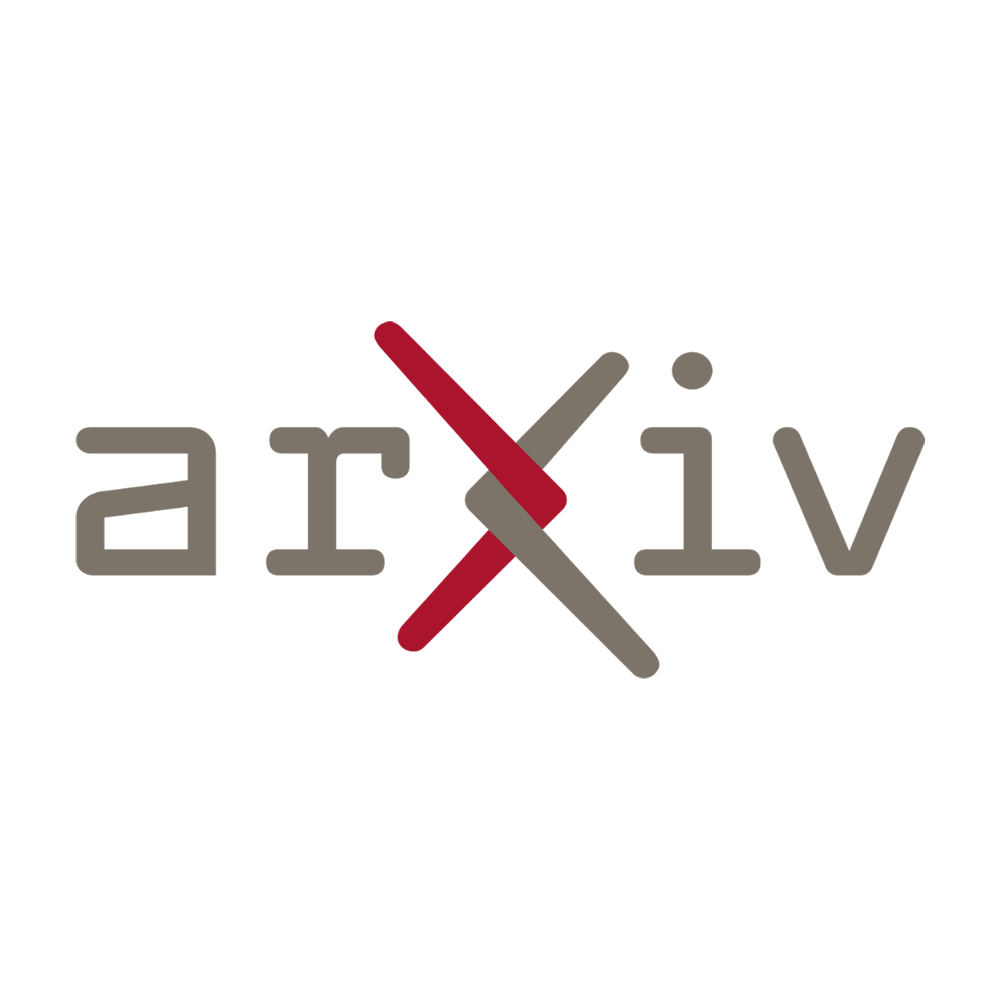
In the ever-evolving field of artificial intelligence, training diffusion models for audiovisual sequences has emerged as a powerful technique. By harnessing the potential of these models, researchers have been able to unlock a multitude of generation tasks by learning conditional distributions of both audio and visual elements. This groundbreaking approach opens up exciting possibilities for creating dynamic and immersive experiences that seamlessly combine the two modalities. In this article, we delve into the core themes of training diffusion models for audiovisual sequences, exploring how this innovative method is revolutionizing the world of AI and paving the way for new and exciting applications.
Exploring the Power of Training Diffusion Models for Audiovisual Sequences
When it comes to generating audiovisual content, the ability to produce realistic and coherent sequences is of utmost importance. Training diffusion models offer a unique solution to this challenge by learning conditional distributions of input-output combinations between the audio and visual modalities. This opens up a plethora of possibilities for various generation tasks, revolutionizing the world of multimedia.
Understanding Diffusion Models
Before delving into the potential applications of training diffusion models for audiovisual sequences, it is important to grasp the fundamentals of diffusion models themselves. Diffusion models are probabilistic models that estimate the probability distribution of a target sample based on a given sequence of data points.
The principle behind diffusion models is simple yet powerful. By iteratively applying small, discrete transformations to the target sample, diffusion models can gradually transform it into a sample following the desired probability distribution. This process, known as diffusion, breaks down complex tasks into simpler steps, making it ideal for training complex and high-dimensional data sequences.
Expanding to Audiovisual Sequences
Traditionally, audio and visual modalities have been treated separately in research and application domains. However, training diffusion models enable the fusion of these modalities, allowing for the generation of audiovisual sequences that possess synchronized and coherent content.
One key advantage of training diffusion models for audiovisual sequences is the ability to align the audio and visual components seamlessly. By learning the conditional distribution between the audio and visual modalities, the models can generate realistic audiovisual sequences that are temporally and semantically consistent. This paves the way for various applications in areas such as video synthesis, lip syncing, and audio-driven animation.
Potential Applications
The introduction of training diffusion models for audiovisual sequences unlocks a realm of innovative possibilities. Let’s explore a few potential applications:
- Automatic Dubbing: By training diffusion models on large datasets of audio and video pairs with corresponding translations, we can generate dubbed versions of videos in different languages, without the need for extensive manual effort.
- Immersive Multimedia: Diffusion models can facilitate the generation of immersive multimedia experiences. By conditioning the model on user interactions, we can create personalized audiovisual content that adapts to the viewer’s preferences and engagement.
- Virtual Reality (VR): Training diffusion models can enhance the realism of virtual reality environments. By integrating audiovisual sequences generated by the models, we can create immersive VR experiences that provide users with a deeper sense of presence and engagement.
Achieving Fidelity and Creativity
While training diffusion models for audiovisual sequences opens up countless opportunities, ensuring the fidelity and creativity of the generated content remains a crucial aspect. Striking the right balance between realism and creative exploration is a challenge that must be addressed.
“Diffusion models possess the potential to revolutionize audiovisual content generation, but it is of utmost importance to constantly fine-tune and refine the models to maintain the desired quality and diversity in the generated sequences. Pushing the boundaries of what is possible without sacrificing the essence of human creativity should be the driving force.”
As researchers and practitioners venture into the uncharted territories of audiovisual content generation, it is essential to embrace continuous improvement and innovation.
Conclusion
The training of diffusion models for audiovisual sequences introduces a new paradigm in multimedia generation. By learning conditional distributions between audio and visual modalities, these models revolutionize the generation of synchronized and coherent audiovisual content. The endless possibilities in fields such as automatic dubbing, immersive multimedia, and virtual reality emphasize the transformative power of these models. However, it is important to strike a delicate balance between fidelity and creativity, constantly pushing boundaries while respecting human ingenuity. There is no doubt that training diffusion models for audiovisual sequences heralds a new era of multimedia design and innovation.
Training diffusion models for audiovisual sequences is an exciting development in the field of machine learning. By learning conditional distributions of both audio and visual inputs, these models can generate a wide range of outputs, opening up new possibilities for creative applications.
One key advantage of training diffusion models for audiovisual sequences is their ability to capture the complex dependencies between audio and visual information. By jointly modeling these modalities, the models can learn to generate realistic and synchronized audiovisual content. This has huge implications for tasks such as video synthesis, where generating coherent audiovisual sequences is crucial.
Additionally, training diffusion models for audiovisual sequences can enable novel tasks such as audio-driven video generation. By conditioning the model on a given audio input, it can generate corresponding video frames that are synchronized with the audio. This has potential applications in areas such as automatic lip-syncing in video editing or even creating realistic animations from audio descriptions.
Another interesting aspect of training diffusion models for audiovisual sequences is their potential for cross-modal understanding. By learning the conditional distributions of different audiovisual input-output combinations, these models can gain insights into the underlying relationships between the two modalities. This can have implications for tasks such as audio-visual scene understanding, where the model can learn to associate specific audio cues with corresponding visual scenes.
Looking ahead, one possible direction for further exploration is to incorporate additional modalities, such as textual information, into the diffusion models. This could allow for even richer and more diverse generation tasks, where the model can generate audiovisual sequences based on textual descriptions or generate textual descriptions based on audiovisual inputs. This multi-modal approach has the potential to unlock new possibilities in areas like video captioning or audiovisual storytelling.
Moreover, improving the scalability and efficiency of training diffusion models for audiovisual sequences is an important area of future research. As these models become more complex, training them on large-scale datasets can be computationally expensive. Developing techniques to accelerate the training process or optimize the model architecture can help make these models more accessible and practical for real-world applications.
In conclusion, training diffusion models for audiovisual sequences holds great promise for generating realistic and synchronized audiovisual content, enabling tasks like video synthesis, audio-driven video generation, and cross-modal understanding. Further advancements in this field, including incorporating additional modalities and improving scalability, will undoubtedly lead to even more exciting applications and advancements in the future.
Read the original article