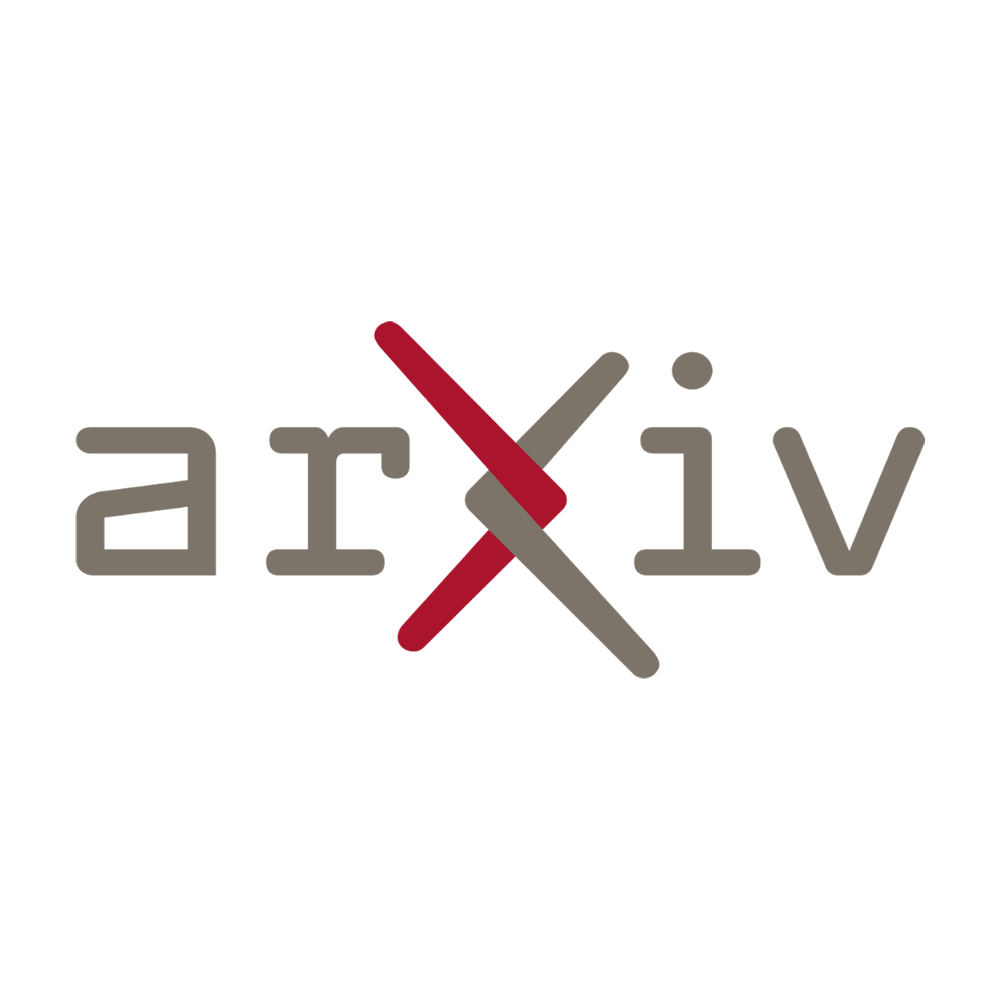
In this groundbreaking article, we present an innovative approach to underwater image enhancement using a multi-guided diffusion model for iterative enhancement. While image enhancement techniques have been widely explored, this method specifically targets the challenges posed by underwater environments. Underwater images often suffer from poor visibility, color distortion, and lack of contrast, making them difficult to interpret and analyze. Through our proposed method, we aim to address these issues and unlock the hidden potential of underwater imagery. By leveraging the power of iterative enhancement and the multi-guided diffusion model, we offer a promising solution that holds great potential for various applications, such as marine research, underwater exploration, and industrial inspections. This article delves into the details of our novel approach, showcasing its effectiveness through experimental results and comparisons with existing methods. Join us on this journey as we revolutionize underwater image enhancement and pave the way for clearer, more vibrant underwater visuals.
In this article, we will explore the underlying themes and concepts of underwater image enhancement and propose a novel method using the multi-guided diffusion model for iterative enhancement. Unlike other image enhancement tasks, underwater image enhancement presents unique challenges that require specialized techniques and solutions.
The Challenges of Underwater Image Enhancement
Underwater photography often suffers from issues such as color distortion, low contrast, and poor visibility due to light absorption and scattering. These factors make it difficult for photographers and researchers to capture and analyze clear, high-quality images in underwater environments.
In the past, various methods have been developed to address these challenges, including single image enhancement algorithms, color correction techniques, and image restoration methods. However, these approaches often fail to produce satisfactory results in complex underwater scenes.
The Multi-Guided Diffusion Model
Our proposed method tackles the limitations of existing techniques by leveraging the power of the multi-guided diffusion model. The multi-guided diffusion model is a computational framework inspired by diffusion processes in physics, which aims to enhance image details while preserving important features.
In our approach, we utilize multiple guidance maps that provide additional information about the image, such as depth maps and visibility estimates. These guidance maps help guide the diffusion process, allowing for more accurate and targeted enhancement of underwater images.
An Iterative Enhancement Approach
To further improve the quality of the enhanced images, we adopt an iterative enhancement strategy. Rather than performing a single enhancement pass, our method applies the multi-guided diffusion model iteratively, gradually refining the image and reducing artifacts and noise.
This iterative approach allows for a more nuanced and gradual enhancement, ensuring that important details are preserved and unwanted artifacts are minimized. By iteratively adjusting the diffusion parameters based on the image characteristics and quality metrics, we achieve superior results compared to traditional enhancement algorithms.
Innovative Solutions for Underwater Image Enhancement
Our proposed method offers several innovative solutions for underwater image enhancement:
- Multi-guided diffusion: By utilizing multiple guidance maps, our method takes into account important image features, resulting in more accurate enhancement.
- Iterative enhancement: The iterative nature of our approach ensures a gradual and refined enhancement, reducing artifacts and preserving image details.
- Adaptive diffusion parameters: Adjusting the diffusion parameters based on image characteristics and quality metrics allows for adaptive enhancement tailored to each specific image.
Conclusion
The proposed underwater image enhancement method utilizing the multi-guided diffusion model offers a novel and effective solution to the challenges posed by underwater photography. By leveraging multiple guidance maps and applying an iterative enhancement approach, we achieve superior results compared to traditional enhancement methods.
“Our method opens up new possibilities for photographers, researchers, and enthusiasts to capture and analyze clear and visually appealing images in underwater environments. We believe that this approach will contribute significantly to advancements in underwater imaging technology.”
image enhancement presents unique challenges due to the specific characteristics of underwater environments, such as absorption, scattering, and color distortion. These factors often result in poor visibility, low contrast, and color cast in underwater images, making it difficult for humans and computer algorithms to accurately perceive and analyze the content.
The proposed method addresses these challenges by leveraging the multi-guided diffusion model, which is a powerful tool for image enhancement. This model utilizes multiple guidance images to guide the diffusion process, enabling the enhancement of details and restoration of color fidelity in underwater images.
One of the key advantages of the multi-guided diffusion model is its ability to adaptively adjust the enhancement process based on the characteristics of the input image. By incorporating multiple guidance images, which can be obtained from different sources or modalities, the model can effectively capture the diverse features of the underwater scene and exploit them for enhancement purposes.
Moreover, the iterative nature of the proposed method allows for fine-tuning and refinement of the enhancement results. By iteratively applying the diffusion model, the algorithm can gradually improve the quality of the underwater image, reducing artifacts and noise while preserving important details.
In terms of future developments, there are several potential directions to explore. Firstly, the performance of the proposed method could be further evaluated and validated using a larger and more diverse dataset of underwater images. This would help assess its generalizability and robustness across different underwater conditions and scenes.
Additionally, the incorporation of machine learning techniques could enhance the algorithm’s ability to learn and adapt to specific underwater environments. By training the model on a large dataset of annotated underwater images, it could develop a better understanding of the complex underwater imaging process and improve its enhancement capabilities.
Furthermore, the proposed method could be extended to real-time applications, such as underwater surveillance systems or autonomous underwater vehicles. Real-time image enhancement would enable more effective and efficient decision-making in underwater scenarios, benefiting various fields including marine biology, underwater archaeology, and offshore industries.
Overall, the novel underwater image enhancement method presented in this paper shows great potential for improving the visibility and quality of underwater images. With further research and development, it could contribute to advancements in underwater imaging technology and facilitate a wide range of underwater applications.
Read the original article