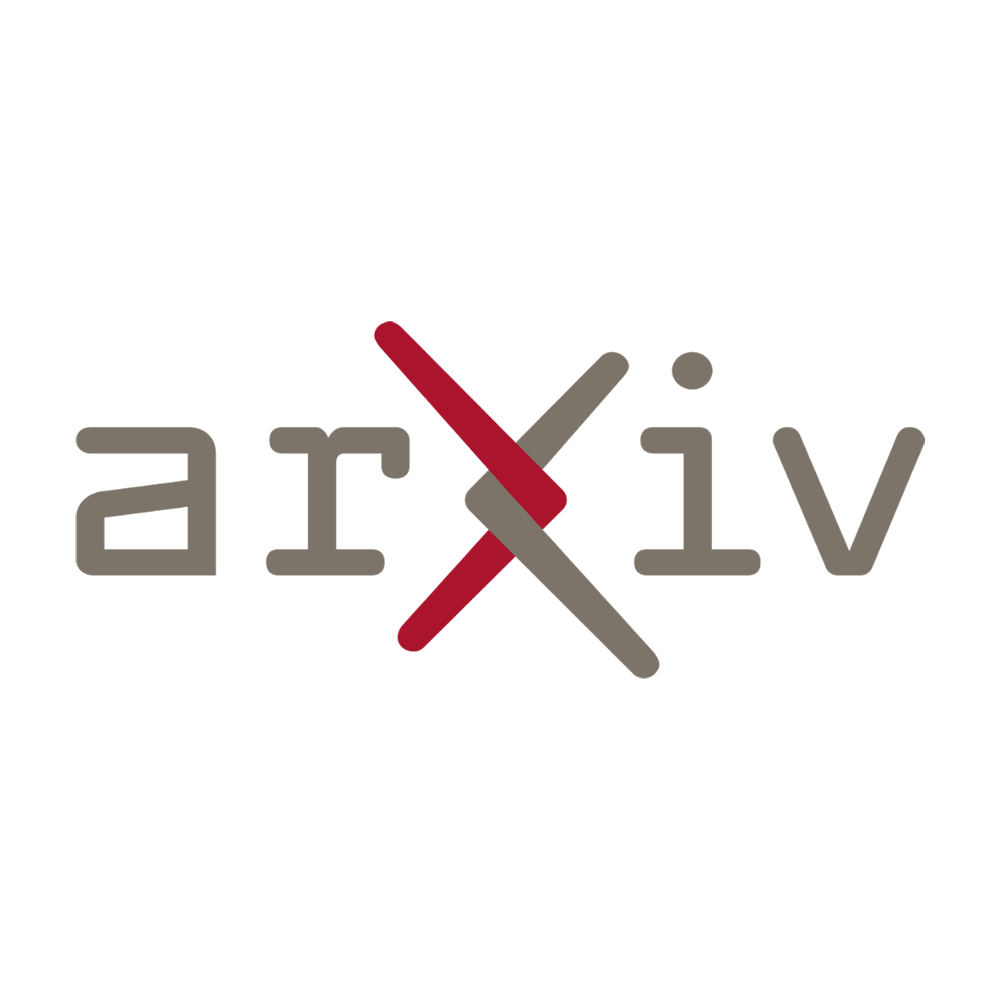
In the realm of research, an intriguing direction has emerged – the generation of interleaved text and images. This fascinating area requires models to create both images and text pieces in any order, presenting a unique challenge for researchers. Despite being a relatively new field, promising advancements have been made, opening up exciting possibilities for the future. This article delves into the core themes surrounding interleaved text-and-image generation, exploring the challenges faced by researchers and the potential implications of their findings.
Interleaved text-and-image generation has been an intriguing research direction, where the models are required to generate both images and text pieces in an arbitrary order. Despite the emerging advancements in this field, there still remains untapped potential and opportunities for further innovation.
The Power of Integration
In order to truly push the boundaries of interleaved text-and-image generation, it is essential to explore the power of integration between these two mediums. Often, text and images are treated as separate entities, with the focus on generating them individually. However, by finding ways to seamlessly integrate the text and image generation processes, we can unlock a whole new realm of possibilities.
Imagine a model that not only generates a coherent paragraph of text, but also produces accompanying visualizations at the same time. This integration would allow for a much richer and more engaging user experience. By combining textual explanations with visually appealing images, complex concepts can be conveyed more effectively to the audience.
Breaking the Traditional Order
In the current approach to interleaved text-and-image generation, the order of generation is often predetermined. However, by breaking free from these constraints, we can introduce a level of flexibility and creativity that was previously unexplored.
Instead of being limited to generating text and images in a fixed order, models should have the ability to dynamically switch back and forth between the two mediums. This would enable a more fluid and interactive generation process, where the model can respond to user inputs and adapt its output accordingly.
Innovative Solutions
One innovative solution to further enhance interleaved text-and-image generation is to introduce a reinforcement learning framework. By incorporating feedback from users and rewards for generating high-quality content, the models can continuously improve and refine their output.
Additionally, the utilization of unsupervised learning techniques can play a crucial role in fueling progress in this field. By leveraging large amounts of unlabeled data, models can learn the underlying patterns and structures of both text and images, leading to more accurate and creative generation processes.
The Future of Interleaved Text-and-Image Generation
The future of interleaved text-and-image generation holds immense potential. As models become more proficient in generating both text and images, we can envision applications in various domains such as educational tools, storytelling, and content creation.
By integrating these two mediums in innovative ways and breaking the traditional order of generation, we can build models that truly excel in creating engaging and informative content. Continued research and exploration in this field will undoubtedly lead to exciting advancements and transformative solutions.
interest and progress in this field, there are still several challenges and opportunities for further exploration.
One of the main challenges in interleaved text-and-image generation is achieving a coherent and meaningful relationship between the generated text and the corresponding image. This requires the model to understand the semantic connections and dependencies between visual and textual elements. While current models have shown promising results, there is still room for improvement in capturing the nuanced interactions between images and text.
Another challenge lies in generating diverse and creative outputs. Many existing models tend to produce generic and predictable combinations of text and image, lacking novelty and uniqueness. To address this, researchers could explore incorporating techniques from creative writing and visual arts to encourage more imaginative and unconventional outputs.
Furthermore, there is a need for better evaluation metrics in this domain. Traditional evaluation methods, such as BLEU scores for text generation, may not adequately capture the quality and coherence of the combined text-and-image outputs. Developing novel evaluation metrics that consider both visual and textual aspects would be valuable for assessing the performance of models in this area.
In terms of future directions, one potential avenue is exploring the use of multimodal pre-training. Pre-training models on large-scale multimodal datasets, such as images with corresponding captions, could help in learning better representations of visual and textual information. This could potentially lead to more effective and coherent generation of interleaved text and images.
Additionally, incorporating user feedback and preferences could enhance the user-centric aspect of interleaved text-and-image generation. By allowing users to provide feedback or adjust the output according to their preferences, the models can be fine-tuned to generate content that better aligns with individual needs and expectations.
Overall, interleaved text-and-image generation is a fascinating research direction with numerous opportunities for advancement. By addressing the challenges of coherence, diversity, and evaluation metrics, and by exploring multimodal pre-training and user-centric approaches, we can expect to see significant progress in generating compelling and meaningful combinations of text and images in the future.
Read the original article