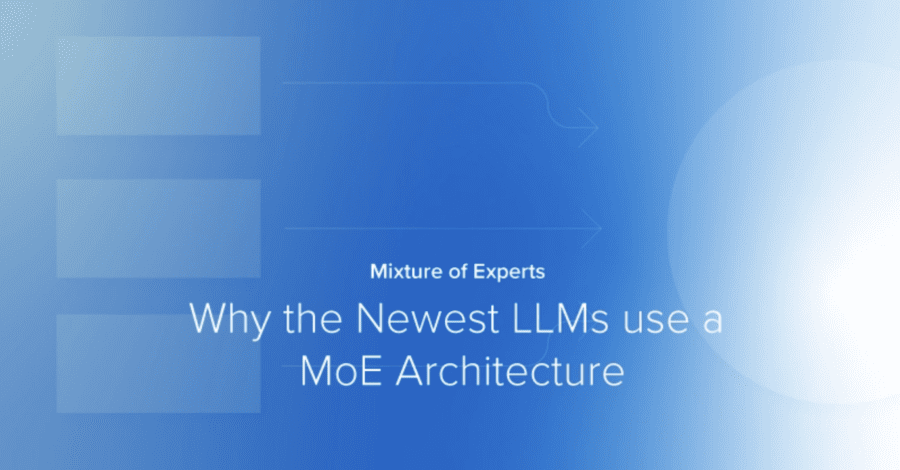
Analysis of Mixture of Experts: Long-term Implications and Future Developments
The Mixture of Experts (MoE) architecture in the field of machine learning presents fascinating prospects. It essentially refers to a blend of different expert, lite and full-fledged sub-models, each focused on a specific aspect of a problem, working in harmony to solve complex tasks. This innovative approach carries enormous potential as it brings together the strengths of multiple specialists to tackle a vast array of problem domains.
Long-Term Implications
Indeed, this paradigm shift will result in manifold implications. For a start, the MoE architecture can lead to a more comprehensive understanding and processing of complex data systems, as each expert sub-model can focus on its own specialty. Additionally, this model suggests the possibility of adaptive learning, where the main system learns to delegate tasks to the appropriate expert based on their performance and expertise in past problem-solving tasks.
The MoE approach is a move towards decentralized problem-solving, offering a more dynamic, adaptable, and nuanced way of data processing and decision making.
On another note, this architecture also encourages continual learning and could open doors for the inclusion of new expert models as technology and understanding of data evolve. Hence, this model has the potential to be future-proof, adapting and evolving alongside technological advancements.
Future Developments
Given the enormous potential of the MoE approach, it’s quite apparent that we can anticipate several key developments in the future. Machine learning models built on the MoE architecture could become more complex, with an increasing number of experts to handle specific domains of a problem. This could result in an unprecedented sophistication of problem-solving capabilities.
The evolution towards systems that allocate tasks more efficiently to the most qualified expert models is another future development that appears probable. Over time, we may even see a rise in the self-improvement of expert models, driven by continual learning from the larger system’s experience of which experts perform best in what circumstances.
Actionable Advice
- Invest in Education: To harness the potential of the MoE architecture, invest in machine learning education and training. As the sector evolves, it’s crucial to stay at the helm of cutting-edge tendencies.
- Build Adaptable Models: When developing machine learning systems, aim at creating adaptable models that can incorporate new technologies and understandings. This will make the system future-proof and enhance its capacity to resolve increasingly intricate issues.
- Focus on Decentralization: Considering the trend toward decentralization in MoE architecture, strive to develop systems that can delegate tasks effectively based on each expert model’s specialization and past performance.
In conclusion, the Mixture of Experts architecture is set to transform the machine learning landscape in fascinating ways. By embracing this model, we can tap into a more dynamic, adaptable and understanding-driven mode of problem resolution. The road ahead is full of opportunities and challenges and it’s up to us to leverage them wisely.