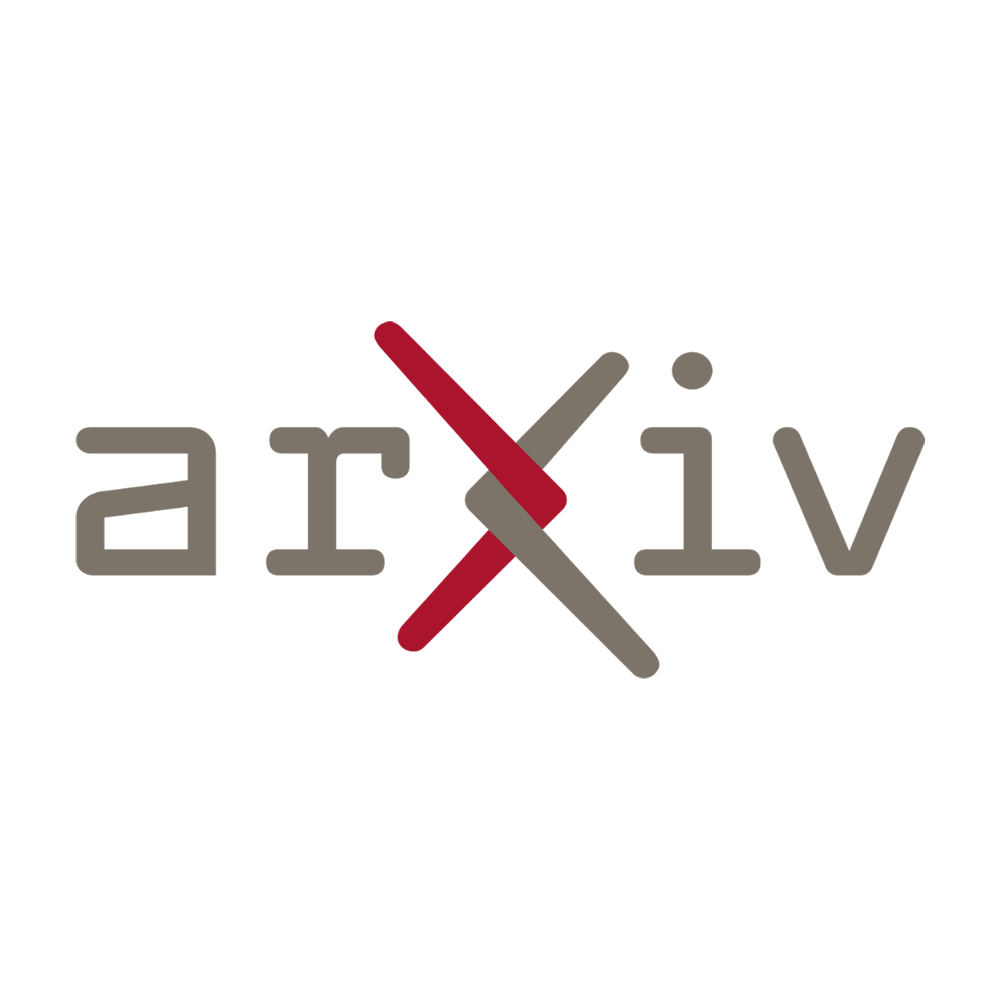
Multimodal sentiment analysis has emerged as a crucial field that seeks to combine information from diverse sources to accurately determine sentiment. This approach becomes particularly important when there are no annotations available for individual sources. Consequently, researchers have focused on developing innovative techniques to leverage the power of multiple modalities in order to enhance sentiment inference. In this article, we delve into the challenges faced in multimodal sentiment analysis and explore the cutting-edge strategies employed to effectively integrate information from various sources, ultimately providing a comprehensive understanding of sentiment.
Multimodal Sentiment Analysis: Exploring Underlying Themes and Proposing Innovative Solutions
Multimodal sentiment analysis is a cutting-edge field that focuses on effectively integrating information from various sources to infer sentiment. While traditional sentiment analysis approaches often rely on textual information alone, multimodal analysis explores the combination of various modalities such as text, images, audio, and video, leading to more comprehensive and accurate sentiment understanding.
In many cases, however, there are no annotations available for unimodal labels, which poses a challenge for training and testing multimodal sentiment analysis models. Therefore, researchers have been actively working on designing innovative solutions and approaches to overcome this limitation.
The Integration of Multiple Modalities
One key theme in multimodal sentiment analysis is the integration of multiple modalities. By combining information from different sources, such as textual content, facial expressions, tone of voice, and visual cues, researchers aim to capture a more holistic representation of sentiment.
“The combination of textual information with visual and auditory signals can lead to a deeper understanding of emotion and sentiment in multimodal data.”
For example, by analyzing facial expressions in videos or images, the model can detect emotions like happiness, sadness, or anger, which may not be explicitly mentioned in the accompanying text. This integration enables a more nuanced interpretation of sentiment, allowing researchers to uncover subtle emotions that might have otherwise been overlooked.
Unsupervised Learning Approaches
Another important concept in multimodal sentiment analysis is the exploration of unsupervised learning approaches. When there are no annotations available for unimodal labels, training models using traditional supervised learning methods becomes challenging.
Unsupervised learning techniques, on the other hand, focus on extracting sentiment patterns from multimodal data without relying on pre-existing annotations. These techniques leverage advanced algorithms to uncover hidden structures and relationships within the data, enabling the model to learn sentiment directly from the input.
Active Learning and Human-in-the-Loop Systems
Active learning and human-in-the-loop systems play a crucial role in enhancing the performance of multimodal sentiment analysis models. By involving human experts in the annotation process, these systems can ensure the availability of labeled data for training the models.
Active learning algorithms select the most informative samples from the unlabeled dataset for human experts to label. As the model gradually learns from the labeled samples, it becomes more accurate in its sentiment predictions. This iterative process allows for the efficient training of the model while minimizing the need for extensive manual labeling.
Conclusion
Multimodal sentiment analysis holds immense potential in various fields, including social media monitoring, market research, and even mental health diagnosis. By exploring the integration of multiple modalities, leveraging unsupervised learning approaches, and incorporating active learning and human-in-the-loop systems, researchers are making significant strides in enhancing the accuracy and applicability of multimodal sentiment analysis.
As this field continues to evolve, it opens up new opportunities for understanding human emotions and sentiments in diverse contexts, contributing to more advanced and intelligent systems that can effectively interpret our feelings and perceptions.
of the existing sentiment analysis approaches focus on utilizing text data alone. However, with the increasing availability of multimodal data such as images, videos, and audio, there is a growing need for methods that can effectively leverage these additional modalities to enhance sentiment analysis.
One of the key challenges in multimodal sentiment analysis is the fusion of information from different modalities. Each modality provides unique cues and context that can contribute to a more comprehensive understanding of sentiment. For example, in analyzing sentiment in a video, facial expressions, body language, and tone of voice can provide valuable insights that are not captured by text alone. Therefore, developing effective fusion techniques that can combine these modalities in a meaningful way is crucial.
Another important aspect is feature representation. Traditional text-based sentiment analysis often relies on lexical and syntactic features extracted from the text. However, in multimodal sentiment analysis, we need to consider how to represent the visual, acoustic, and textual features in a unified manner. This requires the development of novel feature extraction techniques that can capture the complementary information from different modalities.
An additional challenge is the lack of labeled data for multimodal sentiment analysis. While there are large-scale labeled datasets available for text sentiment analysis, annotated datasets for multimodal sentiment analysis are still relatively scarce. This scarcity hinders the development and evaluation of multimodal sentiment analysis models. Therefore, researchers need to explore techniques such as transfer learning and domain adaptation to leverage existing labeled data from related tasks and domains.
Looking ahead, the future of multimodal sentiment analysis holds great promise. As more and more multimedia content is generated and shared on social media platforms, the demand for accurate and efficient sentiment analysis tools will continue to rise. This opens up opportunities for the development of novel deep learning architectures that can effectively handle multimodal data. Additionally, advancements in natural language processing, computer vision, and audio processing will further enhance the capabilities of multimodal sentiment analysis systems.
In conclusion, multimodal sentiment analysis is an emerging field that aims to leverage information from multiple modalities to enhance sentiment analysis. It faces challenges in fusion techniques, feature representation, and the scarcity of labeled data. However, with the rapid advancements in technology and the increasing availability of multimodal data, there is great potential for the development of robust and accurate multimodal sentiment analysis systems in the future.
Read the original article