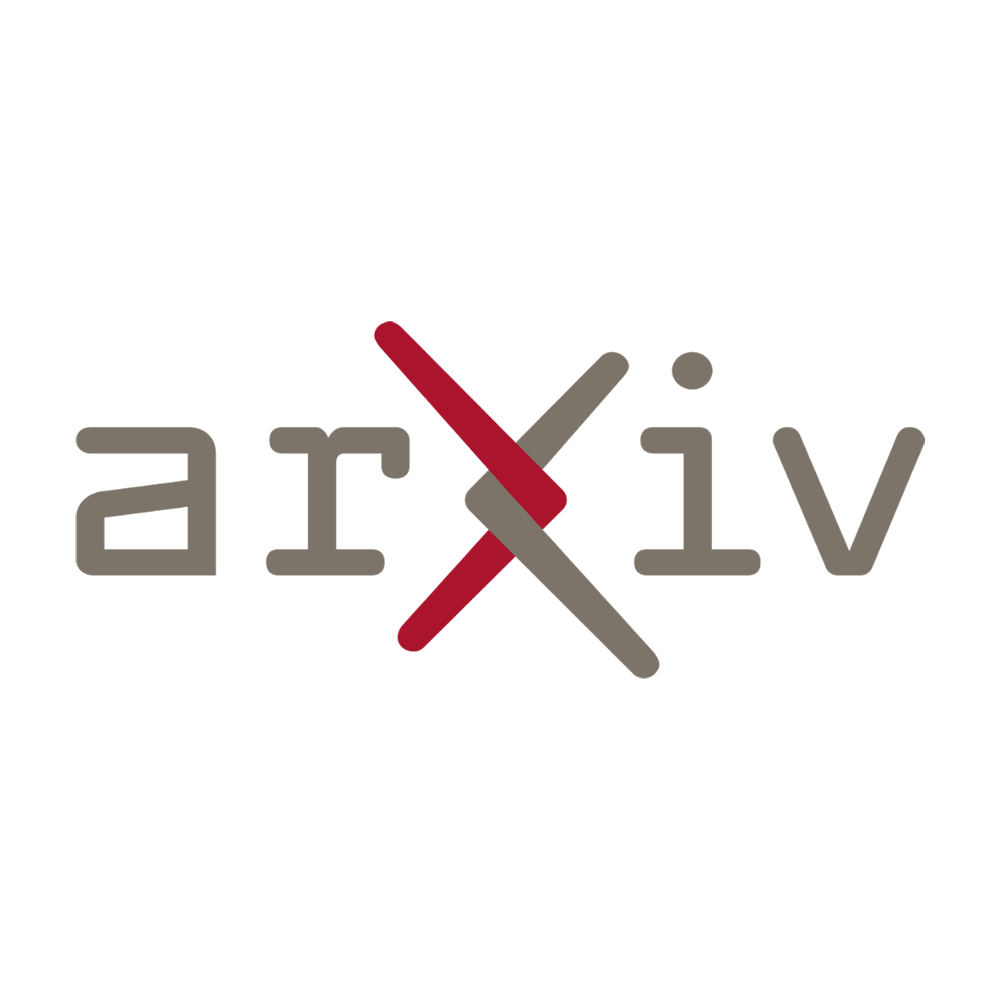
action recognition, which aims to recognize actions from videos captured by multiple cameras. However, these approaches often struggle to handle multiple concurrent or sequential actions. To address this limitation, researchers have turned their attention to multi-label multi-view action recognition. This emerging field aims to not only recognize multiple actions but also identify their occurrence in a sequential or concurrent manner. In this article, we delve into the core themes of multi-label multi-view action recognition, exploring the existing work, challenges, and potential solutions. By doing so, we hope to shed light on the advancements in this field and pave the way for more accurate and comprehensive action recognition in complex video scenarios.
Exploring Multi-Label Multi-View Action Recognition in a New Light
In the field of computer vision, multi-label multi-view action recognition plays a vital role in understanding and analyzing human activities from video data captured by multiple cameras. It involves the recognition of multiple concurrent or sequential actions, which can provide valuable insights for various applications such as video surveillance, human-computer interaction, and sports analysis.
Existing work in this area has primarily focused on multi-view action recognition, mainly in the context of security surveillance systems. However, expanding the scope of this research to encompass multi-label recognition opens up new possibilities and challenges. By considering multiple concurrent or sequential actions, we can gain a more comprehensive understanding of human activities.
The Importance of Multi-Label Recognition
Conventional action recognition may provide accurate results for videos that contain only a single action. But in real-world scenarios, people often perform multiple actions simultaneously or in quick succession. For example, a person walking and talking on the phone or a soccer player dribbling the ball and shooting at the goal. Recognizing and tracking these multiple actions can significantly enhance the precision and applicability of computer vision systems in various domains.
Innovative Solutions for Multi-Label Recognition
Addressing the challenges of multi-label multi-view action recognition requires innovative solutions that go beyond traditional methods. Here, I propose two novel approaches:
1. Temporal Segmentation and Classification
Instead of treating the entire video as a single sequence, we can approach multi-label recognition by segmenting the video temporally into smaller action segments. Each segment can then be classified independently based on its visual features and the context of neighboring segments. By considering the temporal sequence, we can better distinguish and identify individual actions within the video.
2. Knowledge Transfer and Fusion
Another promising solution is to leverage the knowledge from existing single-label action recognition models and transfer it to the multi-label recognition task. By training a network on a large-scale dataset of single-action videos and utilizing transfer learning techniques, we can fine-tune the network to recognize multiple actions simultaneously. Additionally, fusing the outputs of multiple models trained on different cameras can further improve the accuracy and robustness of the system.
The Future of Multi-Label Multi-View Action Recognition
As technology advances and computational resources become more accessible, the potential applications of multi-label multi-view action recognition continue to expand. By incorporating innovative solutions and ideas, we can enhance the capabilities of computer vision systems in domains such as autonomous vehicles, virtual reality, and healthcare.
Multi-label multi-view action recognition has the potential to revolutionize how we analyze and understand human activities from video data. By exploring new approaches and leveraging advanced techniques, we can pave the way for a more accurate and comprehensive understanding of human behavior.
In conclusion, multi-label multi-view action recognition presents an exciting and challenging research area that can significantly contribute to various domains. Through temporal segmentation, classification, knowledge transfer, and fusion techniques, we can unlock the full potential of this technology and pave the way for innovative applications in the future.
action recognition, which involves recognizing actions from videos captured by multiple cameras. However, this approach typically considers only a single action label per video clip, ignoring the fact that multiple actions can occur simultaneously or sequentially in real-world scenarios.
The emergence of multi-label multi-view action recognition addresses this limitation by aiming to recognize multiple concurrent or sequential actions from untrimmed videos captured by multiple cameras. This advancement in the field opens up a wide range of possibilities for applications such as surveillance, sports analysis, and human-computer interaction.
One of the key challenges in multi-label multi-view action recognition is the fusion of information from multiple camera views. Each camera captures a different perspective of the same action, and effectively integrating these views is crucial for accurate recognition. Traditional methods have often relied on handcrafted features and simple fusion techniques, which may not fully exploit the complementary information provided by multiple views.
To overcome this limitation, recent research has focused on deep learning-based approaches for multi-label multi-view action recognition. Convolutional neural networks (CNNs) have shown promising results in various computer vision tasks, and they have been successfully applied to action recognition. By leveraging the power of CNNs, researchers are able to automatically learn discriminative features from the raw video data, enabling more effective fusion of multi-view information.
Another important aspect of multi-label multi-view action recognition is the temporal modeling of actions. Recognizing sequential actions is challenging because it involves understanding the temporal dependencies between different actions. Traditional methods often rely on handcrafted temporal features or simple temporal modeling techniques, which may not capture the complex dynamics of actions accurately.
To address this issue, researchers have explored the use of recurrent neural networks (RNNs) and long short-term memory (LSTM) networks for temporal modeling in multi-label multi-view action recognition. These deep learning-based models are capable of capturing long-term dependencies and temporal context, allowing for more accurate recognition of sequential actions.
Looking ahead, there are several directions in which the field of multi-label multi-view action recognition could evolve. Firstly, there is a need for larger and more diverse datasets to train and evaluate these models. Currently, the availability of such datasets is limited, which can hinder the development and benchmarking of new algorithms.
Secondly, the fusion of multi-view information could be further explored. While deep learning-based approaches have shown promise, there is still room for improvement in terms of how the information from multiple views is combined. Developing more effective fusion strategies could lead to better recognition performance.
Lastly, the incorporation of contextual information could enhance the performance of multi-label multi-view action recognition systems. Actions do not occur in isolation, and considering the context in which they occur can provide valuable cues for recognition. This could involve incorporating scene understanding, object detection, or even human pose estimation to improve the overall recognition accuracy.
In conclusion, multi-label multi-view action recognition is an emerging field that aims to recognize multiple concurrent or sequential actions from untrimmed videos captured by multiple cameras. Recent advancements in deep learning-based approaches and temporal modeling have shown promising results. However, there are still challenges to overcome, such as improving the fusion of multi-view information and incorporating contextual cues. With further research and development, multi-label multi-view action recognition has the potential to revolutionize various domains, including surveillance, sports analysis, and human-computer interaction.
Read the original article