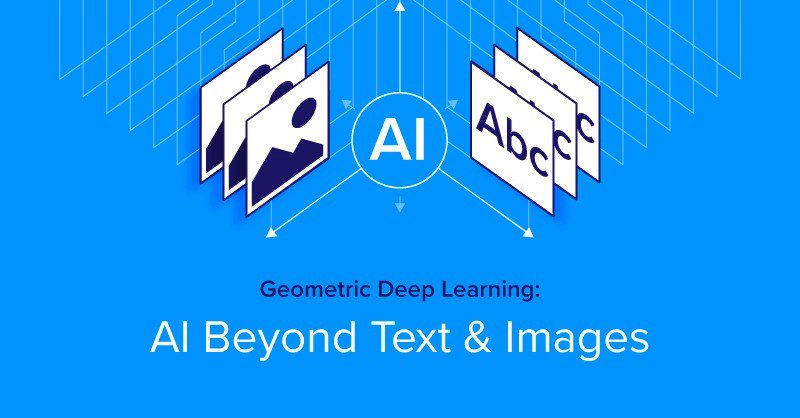
Geometric Deep Learning: Revolutionizing the Field of AI
The integration of Geometric Deep Learning (GDL) into Artificial Intelligence (AI) supports the ability to handle and process complex, non-Euclidean data structures. This groundbreaking advancement provides several promising opportunities, paving the way for notable improvements in various fields such as drug discovery, 3D modeling, and network analysis.
The Implications of Geometric Deep Learning
GDL’s profound ability to process irregular data structures could have remarkable long-term implications. Traditional AI methods often necessitate data to be structured in tabular or Euclidean formats. However, this requirement often inhibits the comprehension and processing of complex, irregular data sets involved in many modern scientific and technological processes.
With GDL, this barrier is effectively obliterated. The technology affords the ability to handle and dissect complex unstructured data. Consequently, it presents the possibility of making significant strides in various scientific fields such as drug discovery, where complex structures of chemical or genetic compounds need to be understood and manipulated.
Possible Future Developments
The advancement in Geometric Deep Learning technology promises exciting future developments within the AI sector. From a potential revolution in drug discovery processes to enhancements in 3D modeling and network analysis, the integration of GDL into traditional algorithms could provide an unprecedented depth and scope of analysis.
Specifically, in the field of drug discovery, GDL could potentials help in interpreting complex molecular structures and interactions. It can also expedite the process by presenting more accurate predictions of how new drugs might interact with a variety of biological systems.
Within the realm of 3D modeling, GDL could offer significant improvements in the manipulation and representation of data. This could ultimately aid architectural planning, video game designs, and other fields that require 3D modeling.
Additionally, for network analysis, GDL may provide a more exhaustive understanding of how data points within a network connect and interact. This could prove invaluable for improving the efficiency of transport systems, optimizing computer networks, or analyzing social networks.
Actionable Advice
Based on these insights, it is worthwhile for organizations dealing with complex, non-Euclidean data structures to consider integrating Geometric Deep Learning into their AI systems. Doing so could provide them with a competitive edge by allowing them to interpret and manipulate complex data more efficiently.
- Drug discovery organizations: Consider leveraging GDL to expedite and enhance the drug discovery process through better understanding of complex biological systems and molecular interactions.
- 3D modeling businesses: Utilize GDL to improve the accuracy and efficiency of your 3D modeling processes, potentially leading to significant time and cost savings.
- Companies dealing with network analysis: Implement GDL to gain deeper insights into network interactions and improve the efficiency of your systems.
In conclusion, the advent of Geometric Deep Learning presents immense potential and opportunities within the realm of AI. Stakeholders across various industries should consider leveraging this technology to optimize their operations and research capabilities.