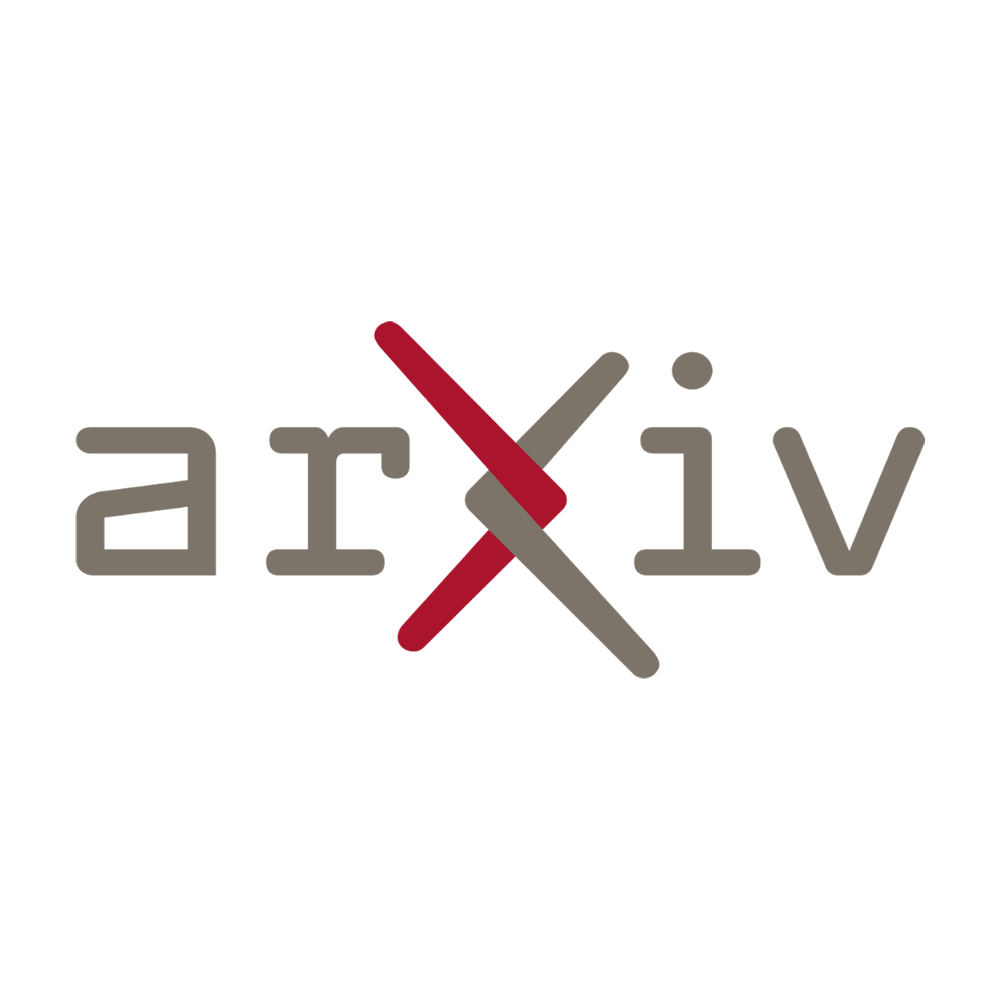
The article explores the growing use of intricate machine learning models in the field of education and the resulting concerns regarding their interpretability. As these models become more prevalent, there is a need to develop techniques that can provide explanations for their decisions and predictions. This article delves into the importance of explainability in machine learning and highlights the efforts being made to address this issue in the educational context.
The Role of Explainable AI in Enhancing Interpretability in Education
With the growing use of complex machine learning models in the field of education, concerns about their interpretability have emerged. The ability to understand and explain the decision-making processes of these AI systems is crucial, as it impacts their trustworthiness, ethical considerations, and overall effectiveness. In response to these concerns, there has been an increasing interest in developing explainability techniques to shed light on the inner workings of AI models, allowing educators and students to have a deeper understanding of their reasoning and recommendations.
The Challenges of Interpreting Machine Learning Models
Machine learning models, such as deep neural networks, are often referred to as “black boxes” due to their complex, non-linear nature. While these models can achieve impressive accuracy and performance, understanding how they arrive at their decisions can be challenging. In education, where transparency, fairness, and accountability are essential, the lack of interpretability poses significant obstacles.
When AI models are used to make decisions about students, such as predicting their academic performance or recommending personalized learning paths, it becomes crucial to ensure that these decisions are both accurate and explainable. For educators to trust and effectively utilize AI tools, they need to be able to comprehend the rationale behind these decisions. Similarly, students deserve to know why certain choices were made on their behalf and understand the factors that contributed to those recommendations.
Exploring Explainability Techniques
Several techniques have emerged to enhance the explainability of machine learning models in education:
- Feature Importance Analysis: By examining the importance of different input features, educators and students can gain insight into which factors influenced the AI model’s decisions the most. This provides a clearer understanding and helps build trust in the system.
- Rule Extraction: This technique aims to extract human-readable rules from complex AI models. By translating the learned patterns and decision rules into understandable formats, educators and students can grasp the underlying logic and reasoning employed by the model.
- Interactive Visualizations: Utilizing interactive visualizations, educators and students can explore the inner workings of AI models in an intuitive manner. These visualizations can display the decision-making process, highlight influential features, and allow users to interactively investigate model behavior.
By employing these techniques, educators and students gain the ability to go beyond blindly relying on AI recommendations. Instead, they become active participants in the decision-making process, learning from AI insights and making informed choices based on a deeper understanding of the underlying data patterns.
The Promise of Explainable AI in Education
Explainable AI not only addresses interpretability concerns but also opens up new avenues for collaboration and educational exploration. By making AI models more transparent and understandable, educators and students can work alongside these systems, contributing their expertise and insights to improve them.
Furthermore, explainable AI can be a valuable learning tool in itself. By providing explanations for model decisions, students can gain deeper insights into the subject matter, better understand their own learning preferences, and receive targeted recommendations for improvement. This synergy between AI and human intelligence has the potential to revolutionize education, fostering personalized and adaptive learning experiences.
Explainable AI not only addresses interpretability concerns but also opens up new avenues for collaboration and educational exploration.
As the field of education embraces AI and machine learning, it is crucial to prioritize the development and integration of explainability techniques. By doing so, we can ensure that AI models are not only accurate but also transparent, understandable, and accountable. The combination of AI’s computational power and human expertise has the potential to create a symbiotic relationship that enhances educational outcomes and prepares students for the challenges of the future.
address this issue. Complex machine learning models, such as deep neural networks, have shown great potential in improving various aspects of education, including personalized learning, student performance prediction, and automated grading systems. However, their black-box nature has raised concerns regarding their interpretability and transparency.
The lack of interpretability in these models is a significant challenge as it hinders the understanding of how they arrive at their decisions or predictions. This is particularly crucial in educational settings, where stakeholders, including teachers, students, and parents, need to comprehend the reasoning behind the model’s outputs to ensure trust and fairness.
To tackle this issue, researchers and educators are actively exploring various explainability techniques. These techniques aim to shed light on the inner workings of complex machine learning models and provide insights into the factors influencing their predictions. By doing so, they enhance transparency, accountability, and trust in the educational applications of these models.
One approach to improving interpretability is the use of attention mechanisms. Attention mechanisms allow models to focus on specific parts of input data that are deemed important for making predictions. By visualizing these attention weights, educators can understand which features or patterns the model is prioritizing, thus gaining insights into its decision-making process.
Another promising technique is the use of rule extraction methods. These methods aim to distill complex machine learning models into simpler rule-based models that are more interpretable. By extracting understandable rules from the black-box models, educators can gain insights into the decision rules employed by these models, facilitating better understanding and trust.
Additionally, researchers are exploring methods to provide explanations alongside model predictions. These explanations can take the form of natural language explanations or visualizations that highlight the key factors considered by the model. By presenting these explanations to stakeholders, educators can ensure transparency and enable informed decision-making based on the model’s outputs.
Looking ahead, the development of explainability techniques will continue to play a crucial role in the adoption and acceptance of complex machine learning models in education. As these techniques evolve, it is expected that educators will have access to more user-friendly tools that provide clear and actionable insights into how these models work. This will not only enhance their trust in the models but also enable them to leverage the models’ capabilities more effectively to support student learning and educational decision-making.
However, it is important to acknowledge that achieving full interpretability in complex machine learning models is a challenging task. As models become more sophisticated and complex, the trade-off between interpretability and performance becomes more pronounced. Striking the right balance between accuracy and interpretability will require ongoing research and collaboration between machine learning experts and education practitioners.
In conclusion, while the increasing use of complex machine learning models in education has raised concerns about their interpretability, the development of explainability techniques offers promising solutions. These techniques, such as attention mechanisms, rule extraction methods, and explanation generation, provide insights into the decision-making processes of these models. As these techniques continue to evolve, they will play a crucial role in enhancing transparency, trust, and informed decision-making in educational settings.
Read the original article