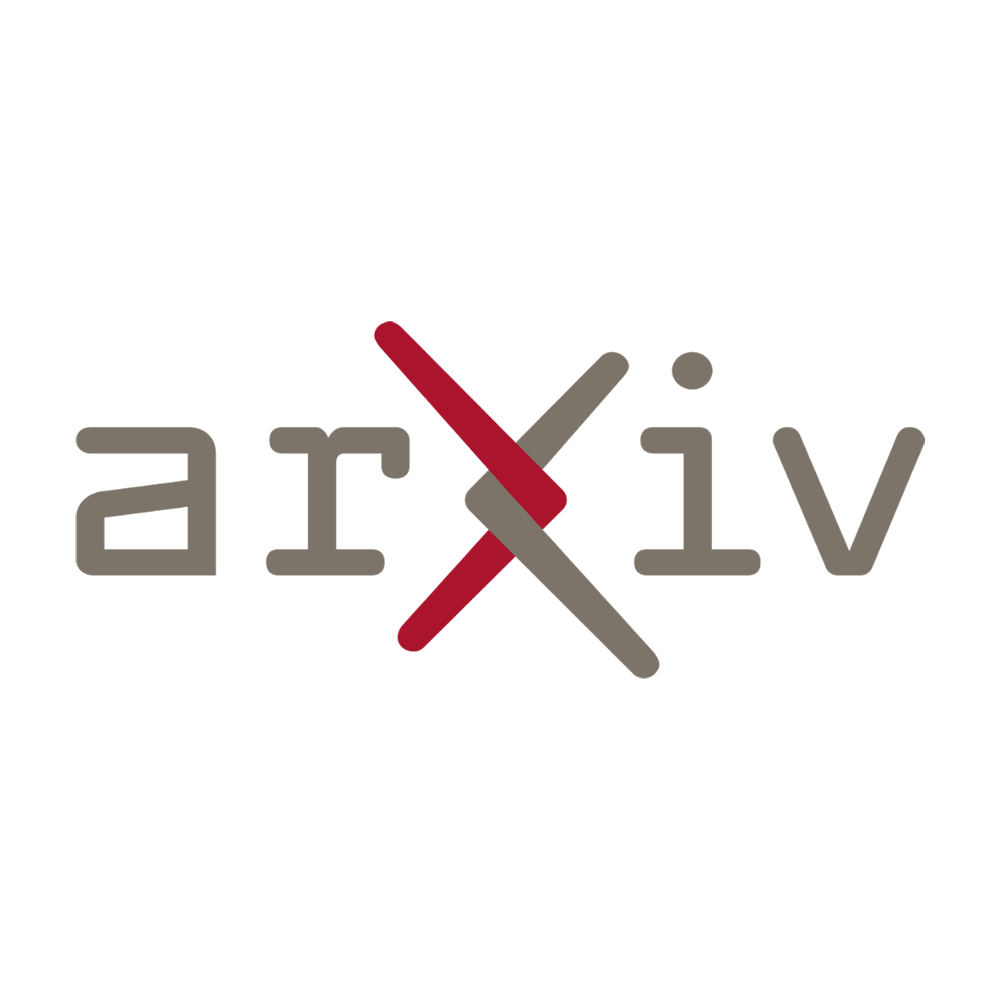
In the fast-paced world we live in, it is crucial to have tools that can adapt to changing environments and handle complex data. In this article, we present an innovative online mathematical framework for survival analysis that does just that. Our framework not only allows for real-time adaptation to dynamic environments but also handles censored data, providing accurate estimations of event time. With this cutting-edge tool, researchers and analysts can now navigate the complexities of survival analysis with ease, unlocking valuable insights in various fields such as healthcare, finance, and social sciences.
Survival Analysis in a Dynamic Environment: A New Mathematical Framework
Survival analysis has long been an essential tool in various fields such as medicine, engineering, and economics. It involves the study of time-to-event data, where events can be anything from the occurrence of a disease to the failure of a mechanical component. Traditionally, survival analysis has focused on analyzing static environments with complete data. However, in today’s fast-paced and ever-changing world, it is crucial to have a framework that can adapt to dynamic environments and handle censored data.
The Challenges of Dynamic Environments
In many real-world scenarios, the factors affecting event times can change over time. For example, in healthcare, the effectiveness of a treatment can vary over different periods as new drugs or therapies are introduced. Similarly, in engineering, the failure rate of a component may change as it ages or when external conditions vary. Traditional survival analysis methods often fail to account for these dynamic factors, leading to inaccurate estimations and predictions.
Censored data poses another challenge in survival analysis. Censoring occurs when the event of interest has not yet occurred for some individuals by the end of the study or observation period. Handling censored data requires sophisticated methods that can properly incorporate this partial information into the analysis.
An Online Mathematical Framework
Addressing the limitations of existing approaches, we propose an online mathematical framework for survival analysis. This framework allows real-time adaptation to dynamic environments and handles censored data in a robust manner. Our method combines elements from machine learning, statistical modeling, and optimization techniques to provide accurate estimations and predictions even in rapidly changing scenarios.
The core idea behind our framework is to continuously update and refine the survival models as new data becomes available. By leveraging online learning algorithms, we can adapt the models to changing conditions and make adjustments to the estimated survival probabilities. This dynamic approach ensures that the analysis stays relevant and reliable in real-time.
Innovative Solutions and Ideas
Our framework offers several innovative solutions to common challenges in survival analysis:
- Adaptive Survival Modeling: By using online learning algorithms, our framework can adapt the survival models to changing environments. This allows for more accurate estimations of event times, especially when the underlying factors are dynamic.
- Handling Censored Data: Our framework incorporates censored data by utilizing advanced statistical techniques. It considers the partial information provided by censored observations, improving the accuracy of the analysis.
- Real-time Predictions: With its ability to adapt to dynamic environments, our framework enables real-time predictions of event times. This is particularly valuable in situations where timely decisions need to be made, such as healthcare interventions or preventative maintenance in engineering.
- Flexible Implementation: Our framework can be implemented in various domains and can handle different types of event data. It provides a flexible solution that can be customized to specific needs and requirements.
Survival analysis in a dynamic environment requires an innovative and adaptive approach. Our online mathematical framework offers a robust solution for handling dynamic factors and censored data. By continuously updating the models and incorporating new information in real time, our framework provides accurate estimations and predictions. This opens up new possibilities for decision-making in fields such as healthcare, engineering, and beyond.
and survival probabilities in complex scenarios, such as medical research and actuarial science, where time-to-event data is commonly encountered. Survival analysis, also known as time-to-event analysis, is a statistical technique used to analyze the time it takes for an event of interest to occur, such as death, failure of a system, or occurrence of a disease.
The development of an online mathematical framework for survival analysis is a significant advancement in this field. Traditionally, survival analysis has been performed using static models that assume the data is fixed and does not change over time. However, in many real-world applications, the data is dynamic and subject to censoring, where the event of interest has not yet occurred for some subjects at the time of analysis.
By introducing an online framework, researchers and practitioners can now adapt their models and estimates in real time as new data becomes available. This is particularly valuable in situations where the environment is constantly changing, such as in clinical trials or monitoring the progression of diseases.
One key advantage of this framework is its ability to handle censored data. Censoring occurs when the event of interest has not occurred for some subjects within the study period or follow-up time. Traditional methods often treat censored observations as missing data or exclude them from the analysis, leading to biased results. The online framework, however, incorporates these censored observations and provides more accurate estimates of survival probabilities and event times.
Moreover, the online nature of this framework allows for continuous updating of estimates as new data points are collected. This feature is particularly useful in scenarios where data collection is ongoing or when there are delays in obtaining complete information. Researchers can now make more informed and timely decisions based on the most up-to-date information available.
Looking ahead, there are several potential avenues for further development and application of this online mathematical framework for survival analysis. One direction could be to incorporate machine learning techniques to enhance predictive capabilities and identify patterns in the data that may not be captured by traditional parametric models. Additionally, the framework could be extended to handle competing risks, where multiple events of interest may occur, and the occurrence of one event may affect the probability of others.
Furthermore, the implementation of this framework in real-world settings, such as healthcare systems or insurance industries, could provide valuable insights into predicting patient outcomes, optimizing treatment strategies, or assessing risk profiles. By continuously updating survival estimates based on newly collected data, healthcare providers and insurers can make more accurate assessments of individual patient risk and tailor interventions accordingly.
In conclusion, the introduction of an online mathematical framework for survival analysis is a significant advancement in the field. Its ability to adapt to dynamic environments and handle censored data opens up new possibilities for accurate estimation of event times and survival probabilities. This framework has the potential to revolutionize various domains, including medical research, healthcare, and actuarial science, by enabling real-time decision-making and personalized interventions based on the most up-to-date information available.
Read the original article