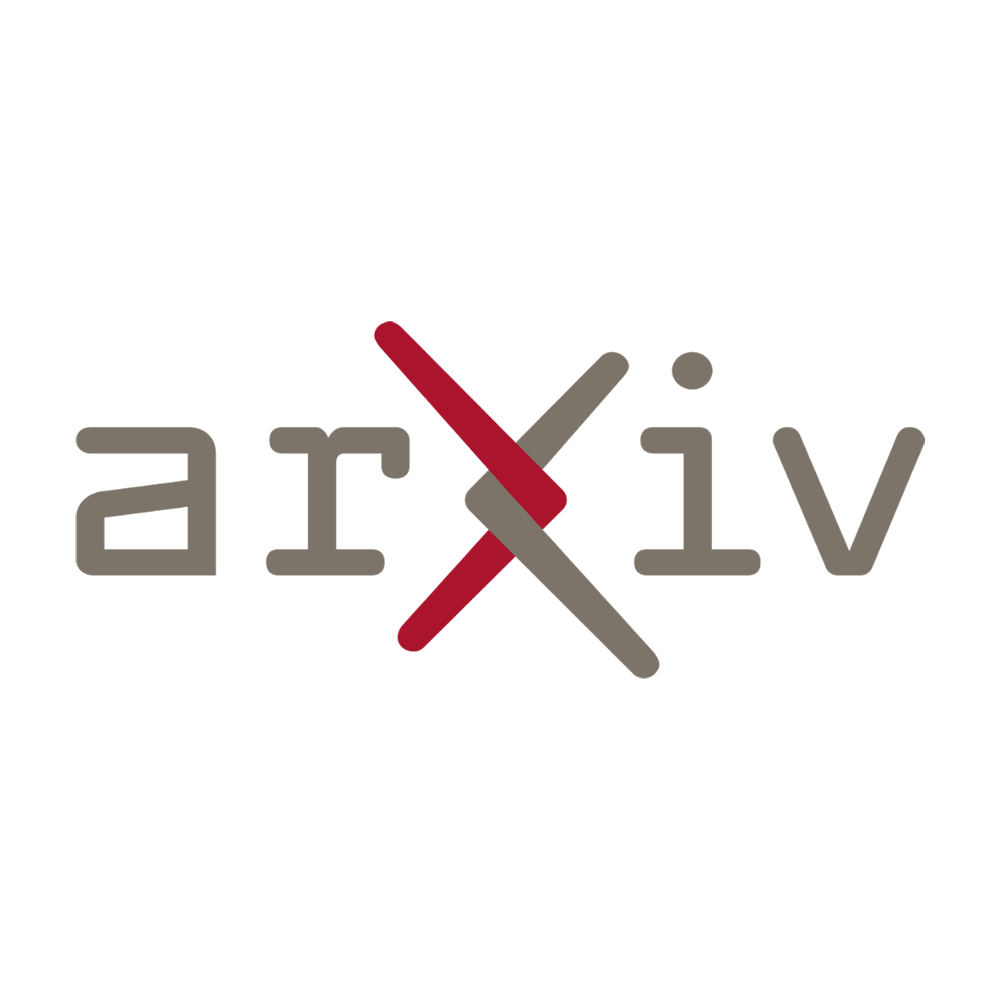
In the realm of graph-based machine learning, a groundbreaking method has emerged: Clifford Group Equivariant Simplicial Message Passing Networks. This innovative approach allows for E(n)-equivariant message passing on simplicial complexes, enabling researchers to harness the power of graph neural networks in a more versatile and flexible manner. By integrating the expressivity of simplicial complexes with the steerable properties of Clifford groups, this method opens up new avenues for understanding and analyzing complex data structures. In this article, we delve into the core themes of this novel technique, exploring its potential applications and the implications it holds for the future of graph-based machine learning.
We introduce Clifford Group Equivariant Simplicial Message Passing Networks, a method for steerable E(n)-equivariant message passing on simplicial complexes. Our method integrates the expressivity of deep learning with the mathematical formalism of equivariant representations, allowing for powerful and flexible modeling of complex data.
Understanding Equivariant Message Passing
In many real-world applications, data is naturally structured in the form of networks or graphs. Traditional deep learning methods struggle to effectively utilize this structural information, often treating the data as independent samples. Equivariant message passing is a powerful approach that takes into account the underlying structure of the data, enabling more accurate and robust predictions.
However, existing equivariant message passing methods are primarily designed for regular Euclidean spaces such as images or grids. Simplicial complexes, which generalize graphs to higher dimensions, present a more challenging and interesting setting. Clifford Group Equivariant Simplicial Message Passing Networks address this challenge and provide a solution for effectively processing and modeling simplicial complexes.
The Power of E(n)-Equivariant Representations
Our method leverages the power of E(n)-equivariant representations, which describe how geometric transformations act on high-dimensional data. This enables us to capture and preserve important geometric properties of simplicial complexes, such as rotational or reflection symmetries.
By aligning the message passing operations with the underlying symmetries of the data, our method is able to learn and extract meaningful features across different dimensions. This results in more informative representations and better generalization capabilities.
Steerable Message Passing
One of the key contributions of Clifford Group Equivariant Simplicial Message Passing Networks is the concept of steerable message passing. Similar to steerable filters in signal processing, steerable message passing allows us to selectively focus on specific geometric transformations during information propagation.
By using the mathematical machinery of the Clifford group, a group that generalizes rotations and reflections in higher dimensions, we can steer the message passing operations to align with desired transformations. This provides us with a level of control and interpretability in our models, enabling us to model and understand data with complex symmetries.
Applications and Future Directions
Clifford Group Equivariant Simplicial Message Passing Networks have the potential to revolutionize the analysis and modeling of complex data with structural dependencies. The ability to effectively capture and leverage geometric symmetries can greatly enhance various fields, including computer vision, graph learning, and molecular biology.
In the future, we envision further advancements in the integration of equivariant message passing with deep learning architectures. Additionally, exploring the application of our method to real-world datasets and domains will help uncover the true potential of E(n)-equivariant message passing on simplicial complexes.
Conclusion: Clifford Group Equivariant Simplicial Message Passing Networks bring together the power of deep learning and equivariant representations to effectively model and analyze complex data structured as simplicial complexes. By providing a steerable approach to message passing, our method offers a flexible and interpretable solution for capturing geometric symmetries and dependencies. This work opens up new possibilities for advancing the field of machine learning on structured data.
of neural networks with the geometric structure of simplicial complexes, allowing for more efficient and effective learning tasks in various domains.
Simplicial complexes are fundamental mathematical objects that capture the connectivity and higher-order relationships within a dataset. By extending the concept of message passing to simplicial complexes, the Clifford Group Equivariant Simplicial Message Passing Networks (CGE-SMPN) offer a powerful framework for analyzing and processing complex data with inherent geometric structure.
One of the key advantages of CGE-SMPN is its ability to preserve equivariance properties. E(n)-equivariance refers to the invariance of neural network operations under Euclidean transformations, such as rotations, translations, and reflections. By incorporating equivariance into message passing on simplicial complexes, CGE-SMPN can effectively handle geometric transformations and capture important spatial patterns.
Furthermore, the integration of Clifford group theory enhances the expressivity of CGE-SMPN. The Clifford group is a mathematical framework that generalizes rotations in higher dimensions, making it well-suited for complex data analysis. By leveraging this theory, CGE-SMPN can capture and model intricate relationships in high-dimensional spaces, enabling more accurate and robust learning.
The implications of CGE-SMPN are vast and have potential applications across multiple domains. For instance, in computational biology, where molecular structures can be represented as simplicial complexes, CGE-SMPN can aid in drug discovery by predicting the interactions between molecules more accurately. Similarly, in computer vision, CGE-SMPN can enhance object recognition by capturing the geometric relationships between different parts of an object.
Looking ahead, there are several exciting directions for further exploration. One potential avenue is the extension of CGE-SMPN to handle dynamic simplicial complexes, where the connectivity and relationships between entities change over time. This would open up possibilities for analyzing time-varying data such as social networks or dynamic molecular systems.
Another area of interest could be the incorporation of uncertainty estimation within CGE-SMPN. Uncertainty quantification is crucial in many applications, such as autonomous driving or medical diagnosis, where knowing the confidence level of predictions is essential. By incorporating uncertainty estimation techniques, CGE-SMPN could provide more reliable and interpretable results.
In summary, Clifford Group Equivariant Simplicial Message Passing Networks offer a promising approach for leveraging the geometric structure of simplicial complexes in combination with neural networks. Their ability to preserve equivariance properties and leverage the expressivity of Clifford group theory opens up new possibilities for analyzing complex data. As researchers continue to explore and refine this methodology, we can expect further advancements and applications that push the boundaries of machine learning in domains where geometric relationships play a crucial role.
Read the original article