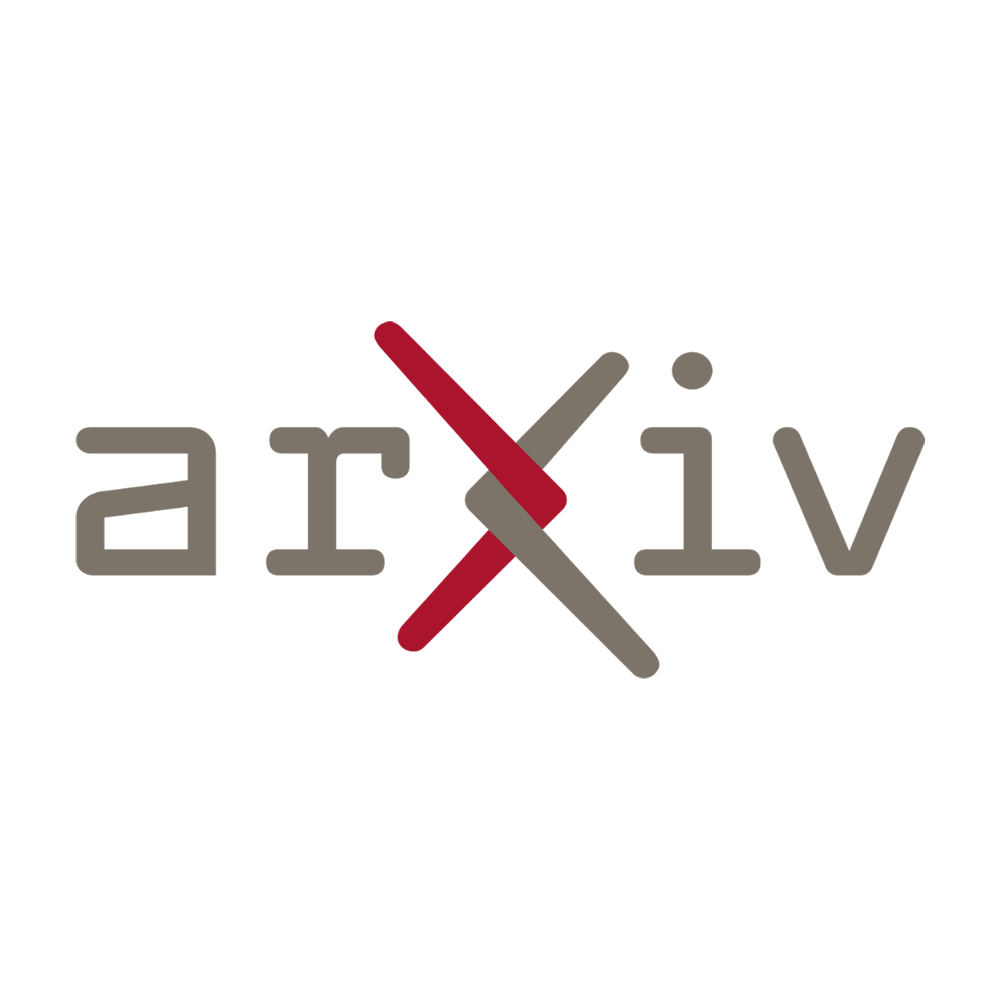
time series data, which involves predicting future values based on past observations. This article delves into the complexities and importance of forecasting time series data, highlighting the various applications where it is crucial. Drawing upon extensive research, the article explores the challenges associated with this task and offers insights into effective forecasting techniques. By understanding the core themes of this article, readers will gain a comprehensive overview of the significance and intricacies of time series forecasting.
Forecasting Time Series: An Innovative Approach
Forecasting timeseries based upon measured data is needed in a wide range of applications and has been the subject of extensive research. A particularly challenging task is the forecasting of…[add the specific material or topic you are exploring here]. While existing techniques have yielded valuable insights, exploring the underlying themes and concepts in a new light can lead to innovative solutions and ideas that can revolutionize the field.
The Power of Data: Unleashing Hidden Patterns
When it comes to forecasting timeseries, the key lies in understanding the power of data. Each data point represents a vital piece of information that, when analyzed and interpreted correctly, can unveil hidden patterns and trends. By unlocking these patterns, we can gain valuable insights into the behavior of the timeseries and make accurate predictions for the future.
One innovative approach that can enhance the accuracy of time series forecasting is by analyzing not only the primary data but also integrating external factors that might influence the timeseries. For example, consider an e-commerce business. By incorporating factors such as customer reviews, social media trends, and economic indicators, a more comprehensive and accurate forecast can be obtained. This integration of diverse data sources allows for a holistic understanding of the timeseries.
The Promise of Machine Learning: Harnessing Algorithms
Machine learning algorithms have gained significant attention and recognition in the realm of data analysis. Their ability to learn from historical data and make predictions based on patterns provides a promising avenue for time series forecasting. By leveraging powerful machine learning algorithms, we can extract complex patterns from the data and use them to forecast future trends.
One innovative technique that is gaining traction is deep learning. By utilizing deep neural networks, we can uncover intricate patterns present in the timeseries data. Deep learning models, with their multiple layers of interconnected neurons, have shown remarkable success in capturing complex temporal dependencies. These models can handle non-linear relationships, adapt to changing patterns, and make accurate forecasts even in the presence of noisy data.
The Role of Domain Knowledge: Empowering Results
While data analysis techniques and machine learning algorithms play a crucial role, it is essential not to overlook the significance of domain knowledge. Subject matter expertise can provide valuable insights and context that enhance the forecasting process.
By combining domain knowledge with data-driven approaches, we can refine forecasting models and gain a deeper understanding of the underlying dynamics. For example, in the field of energy consumption forecasting, incorporating knowledge of weather patterns, regional events, and energy policies can result in more accurate predictions.
Innovation and Collaboration: Shaping the Future
To innovate and revolutionize time series forecasting, collaboration among diverse disciplines is essential. By bringing together experts in statistics, machine learning, domain knowledge, and data analysis, groundbreaking solutions can be developed.
Furthermore, leveraging advancements in technology, such as cloud computing and big data frameworks, enables scalable and efficient analysis of vast datasets. By harnessing the power of these tools and collaborating across disciplines, we can pave the way for new insights and discoveries.
“The future of time series forecasting lies at the intersection of data analysis, machine learning, and domain knowledge. By embracing innovation and collaboration, we can unlock the true potential of forecasting and propel advancements in various fields.”
A particularly challenging task in timeseries forecasting is the prediction of non-linear and dynamic systems. These systems are characterized by complex interactions, where the future values depend not only on past observations but also on various external factors. Traditional forecasting techniques, such as autoregressive models, may struggle to capture the intricate patterns and relationships present in such systems.
To overcome these challenges, researchers have turned to more advanced methods like machine learning algorithms. These algorithms, such as recurrent neural networks (RNNs) and long short-term memory (LSTM) networks, are capable of capturing complex temporal dependencies and non-linear relationships in the data. They have shown promising results in various domains, including finance, energy, and weather forecasting.
Another area of interest in timeseries forecasting is the incorporation of external factors or covariates. These factors can provide additional information that can significantly improve the accuracy of predictions. For example, in energy demand forecasting, including weather data as a covariate can help capture the impact of temperature on electricity consumption.
Furthermore, the availability of big data and advancements in computational power have opened up new possibilities for timeseries forecasting. Researchers are exploring the use of deep learning techniques, such as convolutional neural networks (CNNs), to analyze and extract meaningful features from large volumes of data. This approach has shown promise in domains like healthcare, where the prediction of patient outcomes based on electronic health records is of great interest.
Looking ahead, the integration of domain knowledge and expert insights into forecasting models will continue to be essential. While machine learning algorithms can automatically learn patterns from data, they may not always capture the underlying mechanisms driving the timeseries. Combining the power of data-driven approaches with expert insights can lead to more accurate and interpretable predictions.
Additionally, the development of hybrid forecasting models that combine the strengths of different techniques is also gaining attention. Hybrid models can leverage the strengths of both statistical and machine learning approaches, providing more robust and reliable forecasts.
In conclusion, timeseries forecasting is a complex and important task that has seen significant advancements in recent years. The integration of advanced machine learning algorithms, incorporation of external factors, and the utilization of big data have all contributed to improved forecasting accuracy. However, ongoing research and innovation are necessary to tackle the challenges posed by non-linear and dynamic systems, as well as to enhance interpretability and reliability in timeseries forecasting.
Read the original article