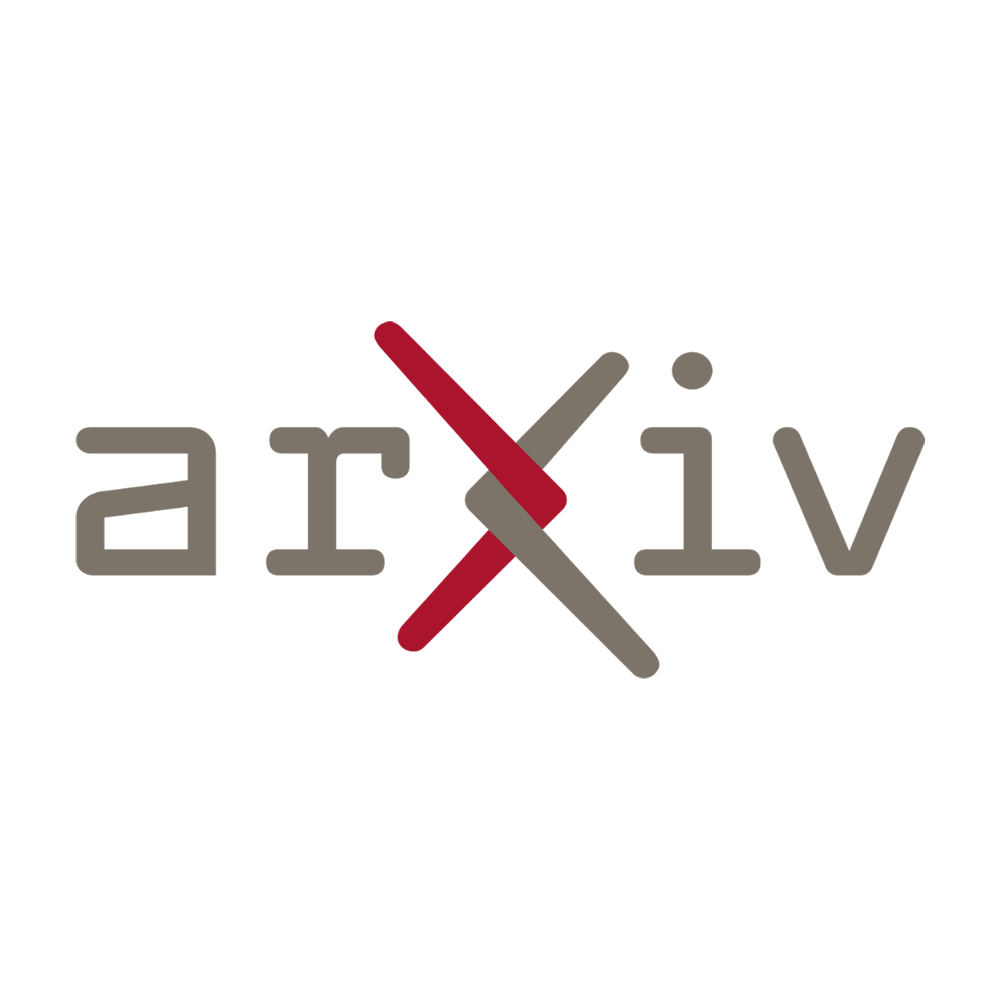
revolutionizing wildfire forecasting by leveraging vast amounts of data and sophisticated algorithms. These models have the potential to predict the behavior and spread of wildfires with unprecedented accuracy, enabling authorities to take proactive measures to mitigate their devastating impact. By analyzing a wide range of variables, including weather patterns, fuel moisture content, and historical fire data, deep learning models can provide invaluable insights into the likelihood and severity of wildfires. This article explores the advancements in deep learning techniques for wildfire forecasting and highlights their potential to revolutionize fire management strategies, ultimately saving lives and protecting ecosystems.
Reimagining Wildfire Forecasting with Deep Learning
Wildfire forecasting has long been a challenging task for scientists and authorities, given the complex interplay of variables such as weather conditions, vegetation types, and human activities. Traditional forecasting methods often struggle to provide accurate predictions, leaving communities vulnerable to the devastating impact of wildfires. However, there is hope on the horizon as deep learning models show promise in revolutionizing the way we predict and mitigate wildfires.
The Power of Deep Learning
Deep learning, a subset of artificial intelligence, has proven its potential in various fields, from image recognition to natural language processing. By training complex neural networks on vast amounts of data, deep learning models can identify subtle patterns and correlations that human experts may overlook.
When it comes to wildfire forecasting, harnessing the power of deep learning can offer significant improvements. These models can incorporate a vast array of variables, including weather data, historical wildfire patterns, topography, vegetation maps, and even social media data. By analyzing and synthesizing this wealth of information, deep learning models can provide more accurate and timely predictions.
Integrating Real-Time Data
One of the most exciting aspects of deep learning models is their ability to integrate real-time data into the forecasting process. Traditional methods often rely on historical data and predefined rules, limiting their adaptability to rapidly changing conditions. Deep learning models, on the other hand, can constantly update their predictions as new data becomes available.
Imagine a system that continuously monitors weather conditions, satellite imagery, sensor data, and social media feeds, combining this information with historical patterns. By assessing the interplay of these variables in real-time, deep learning models can provide up-to-the-minute wildfire forecasts, empowering authorities and communities to take proactive measures to prevent or mitigate the spread of fires.
Empowering Early Intervention
Another crucial aspect of deep learning models for wildfire forecasting is their potential to enable early intervention. By accurately predicting the likelihood and potential trajectory of wildfires, authorities can mobilize resources and implement targeted preventive measures before the situation escalates.
Deep learning models can identify factors such as vulnerable areas, high-risk ignition sources, and the likelihood of rapid fire spread based on environmental conditions. This information can be used to strategize fire prevention efforts, allocate firefighting resources, and even plan evacuation routes accurately. By leveraging the power of deep learning, we can reduce the loss of lives, property, and ecological damage caused by wildfires.
Bridging the Gap: Collaboration and Data Sharing
While deep learning models offer great potential, their success relies heavily on collaboration and data sharing. To train accurate models, we need access to comprehensive and diverse datasets that encompass various geographical regions, climate types, and socio-economic factors.
Researchers, scientific institutions, governments, and technology companies must collaborate to collect and share data, ensuring that deep learning models capture the complexity of wildfire dynamics accurately. Open-source initiatives and partnerships are vital in this regard, fostering innovation and advancing the collective understanding of wildfire forecasting.
It is only through interdisciplinary collaboration and a shared commitment to data-driven solutions that we can harness the full potential of deep learning in wildfire forecasting.
A Safer, More Resilient Future
Incorporating deep learning models into wildfire forecasting holds the promise of a safer and more resilient future. By leveraging the power of artificial intelligence and real-time data integration, we can significantly improve the accuracy and timeliness of wildfire predictions. This, in turn, enables early intervention and empowers communities to take proactive measures to safeguard lives and property.
However, we must remember that deep learning models are not a panacea; they are tools that require continual refinement and adaptation. Ongoing research, validation, and improvement are essential to maximize their potential and address any limitations.
By embracing innovation, collaboration, and a data-driven approach, we can reimagine wildfire forecasting and create a future where lives and landscapes are protected from the devastating impact of wildfires.
improving wildfire forecasting by leveraging their ability to process vast amounts of data and identify complex patterns. These models have the potential to revolutionize the field of wildfire prediction and provide more accurate and timely information to firefighters, land managers, and communities at risk.
One of the key advantages of deep learning models is their ability to handle large and diverse datasets. They can incorporate data from various sources, including satellite imagery, weather forecasts, historical fire data, and even social media feeds. By analyzing these inputs, deep learning models can identify hidden relationships and patterns that may not be apparent to human experts.
Moreover, deep learning models can capture the dynamic nature of wildfires, taking into account the changing weather conditions and vegetation characteristics. This allows for real-time predictions and the ability to update forecasts as new data becomes available. By continuously learning from new information, these models can adapt and improve over time, enhancing their predictive accuracy.
However, it is important to note that deep learning models are not a silver bullet, and there are challenges that need to be addressed. One of the main challenges is the availability and quality of data. Accurate and up-to-date data is crucial for training and validating these models. Additionally, the interpretability of deep learning models can be a concern. Understanding how and why a model makes a particular prediction is essential for gaining trust and acceptance from stakeholders.
To overcome these challenges, collaborations between researchers, government agencies, and technology companies are crucial. By pooling resources and expertise, we can ensure the development of robust and reliable deep learning models for wildfire forecasting. Furthermore, efforts should be made to integrate these models into existing wildfire management systems and workflows, allowing for seamless integration and adoption.
Looking ahead, the future of wildfire forecasting lies in the continued advancement of deep learning models, coupled with the integration of other emerging technologies such as remote sensing and Internet of Things (IoT) devices. These technologies can provide real-time data on various environmental variables, further enhancing the accuracy and timeliness of wildfire predictions.
In conclusion, deep learning models hold great promise for wildfire forecasting, offering the potential to revolutionize the field and improve our ability to predict and mitigate the devastation caused by wildfires. However, ongoing research, collaboration, and data availability are crucial to harnessing the full potential of these models and ensuring their successful integration into wildfire management practices.
Read the original article