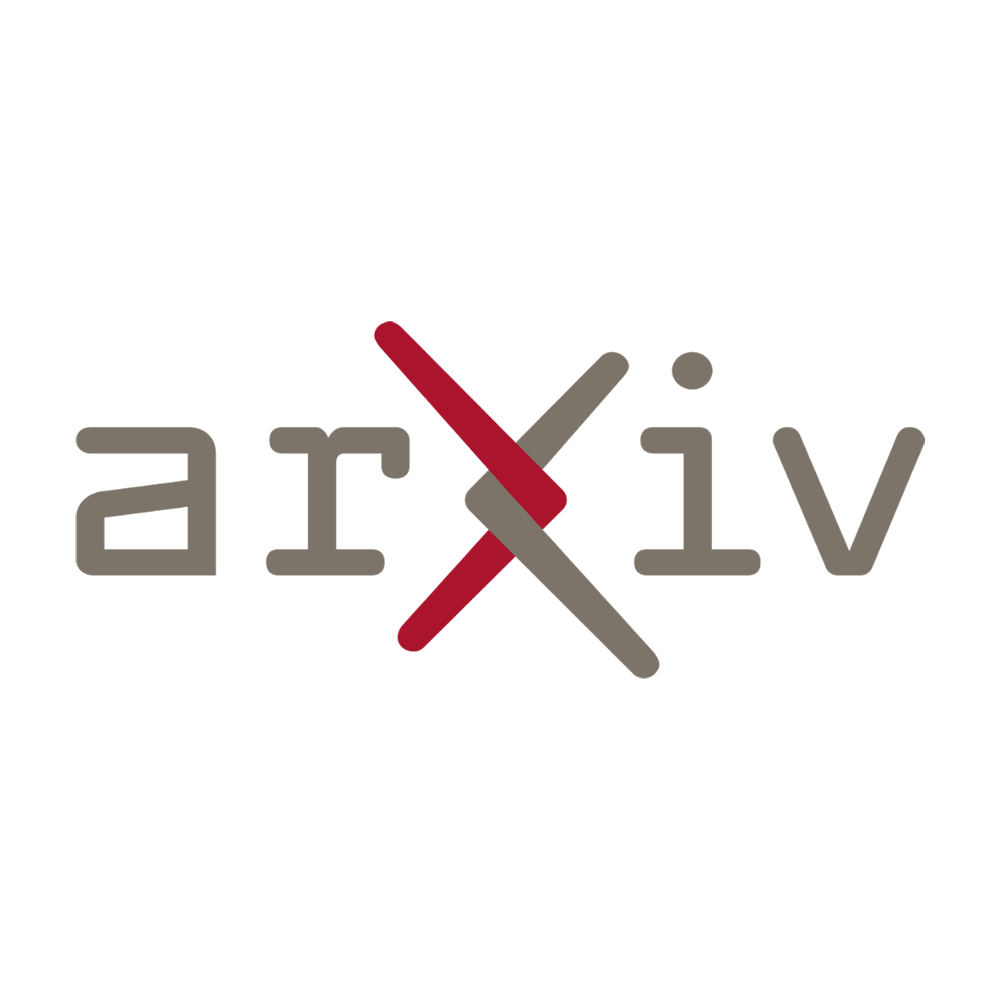
In the world of computer vision and graphics, scene reconstruction from multi-view images is a critical challenge. It involves reconstructing a three-dimensional representation of a scene using multiple images taken from different viewpoints. This process has seen significant advancements with the introduction of neural implicit surface reconstruction methods. These innovative techniques have proven to deliver exceptional results, enabling the creation of high-quality and realistic three-dimensional models. In this article, we delve into the advancements made in this field and explore the potential implications for various industries that rely on accurate scene reconstruction.
The Power of Neural Implicit Surface Reconstruction in Computer Vision and Graphics
In the world of computer vision and graphics, scene reconstruction from multi-view images has long been a significant challenge. Recent innovations in the field have seen the emergence of neural implicit surface reconstruction methods, which have proven to be a game-changer in terms of their ability to generate high-quality results.
A New Horizon in Scene Reconstruction
The traditional approach to scene reconstruction involves using explicit representations, such as meshes or point clouds, to capture the geometry of objects in a given space. However, these explicit representations often fall short when it comes to capturing fine details or dealing with complex shapes.
Neural implicit surface reconstruction, on the other hand, takes a different route. Instead of explicitly representing the surface geometry, these methods employ neural networks to implicitly encode the surface as a continuous function. This approach allows for a more flexible representation, capable of capturing intricate details while being robust to noise and uncertainties.
Unleashing the Power of Neural Networks
One of the key advantages of neural implicit surface reconstruction is its ability to leverage the power of neural networks. By training these networks on large datasets containing ground truth geometry information, they can learn to generalize and generate accurate surface reconstructions even from limited or noisy input data.
This opens up new possibilities in various domains. For example, in the field of virtual reality, where realistic object rendering is crucial for immersive experiences, neural implicit surface reconstruction can provide highly detailed and visually pleasing models. In robotics, this technology can enable improved perception and manipulation capabilities, allowing robots to interact with their environment more effectively.
Challenges and Innovative Solutions
While neural implicit surface reconstruction holds tremendous potential, it also faces its fair share of challenges. One such challenge is the training of these networks, which can be computationally expensive and require large amounts of annotated data. Additionally, the issue of generalizability to unseen or novel object classes is an ongoing area of research.
However, researchers have been actively exploring innovative solutions to overcome these challenges. Transfer learning techniques, for instance, have shown promise in reducing the data requirements for training neural implicit surface reconstruction networks. By leveraging knowledge learned from related tasks or datasets, these techniques allow for more efficient and effective network training.
Shaping the Future of Computer Vision and Graphics
The advancements in neural implicit surface reconstruction methods are undoubtedly reshaping the landscape of computer vision and graphics. As researchers continue to push the boundaries of what is possible, we can expect to see even more sophisticated models capable of capturing both the geometry and appearance of objects with remarkable accuracy.
Moreover, these innovations can lead to various practical applications. From realistic virtual environments to improved robot perception, the potential is vast. Industries such as gaming, architecture, film production, and healthcare could all benefit from the ability to generate highly detailed and lifelike scene reconstructions.
Neural implicit surface reconstruction is revolutionizing the way we reconstruct scenes from images. Its ability to capture intricate details, leverage the power of neural networks, and overcome challenges through innovative solutions is propelling computer vision and graphics into an exciting future.
however, they often require a large number of input views to accurately reconstruct the scene. This can be computationally expensive and may not be feasible in certain scenarios.
One potential direction for future research is to explore ways to improve the efficiency of multi-view scene reconstruction using neural implicit surface methods. This could involve developing novel architectures or techniques that can achieve comparable results with fewer input views. By reducing the number of views required, these methods could significantly speed up the reconstruction process and make it more practical for real-time applications.
Another interesting avenue for exploration is the integration of other modalities, such as depth information or semantic cues, into the multi-view reconstruction pipeline. By leveraging additional sources of information, it may be possible to further enhance the quality and accuracy of the reconstructed scenes. For example, depth information can provide valuable geometric constraints that can help refine the reconstructed surfaces, while semantic cues can aid in understanding the objects and their relationships in the scene.
Furthermore, there is room for improvement in handling challenging scenarios, such as scenes with transparent or reflective surfaces. These surfaces often introduce ambiguities and complexities in the reconstruction process, as they can lead to inconsistent depth estimates across different views. Developing robust techniques that can effectively handle such scenarios would be a significant contribution to the field.
In addition to improving the reconstruction quality, it is crucial to consider the scalability of these methods. As the number of input views increases, the computational requirements also grow. Therefore, finding ways to optimize the resource utilization and memory footprint of neural implicit surface reconstruction algorithms will be important for their widespread adoption.
Overall, the recent advancements in neural implicit surface reconstruction have opened up exciting possibilities for scene reconstruction from multi-view images. By addressing the challenges of efficiency, integration of additional modalities, handling complex scenarios, and scalability, we can expect future research to push the boundaries of what is achievable in this field. These advancements will have significant implications not only in computer vision and graphics but also in applications such as augmented reality, virtual reality, and robotics.
Read the original article