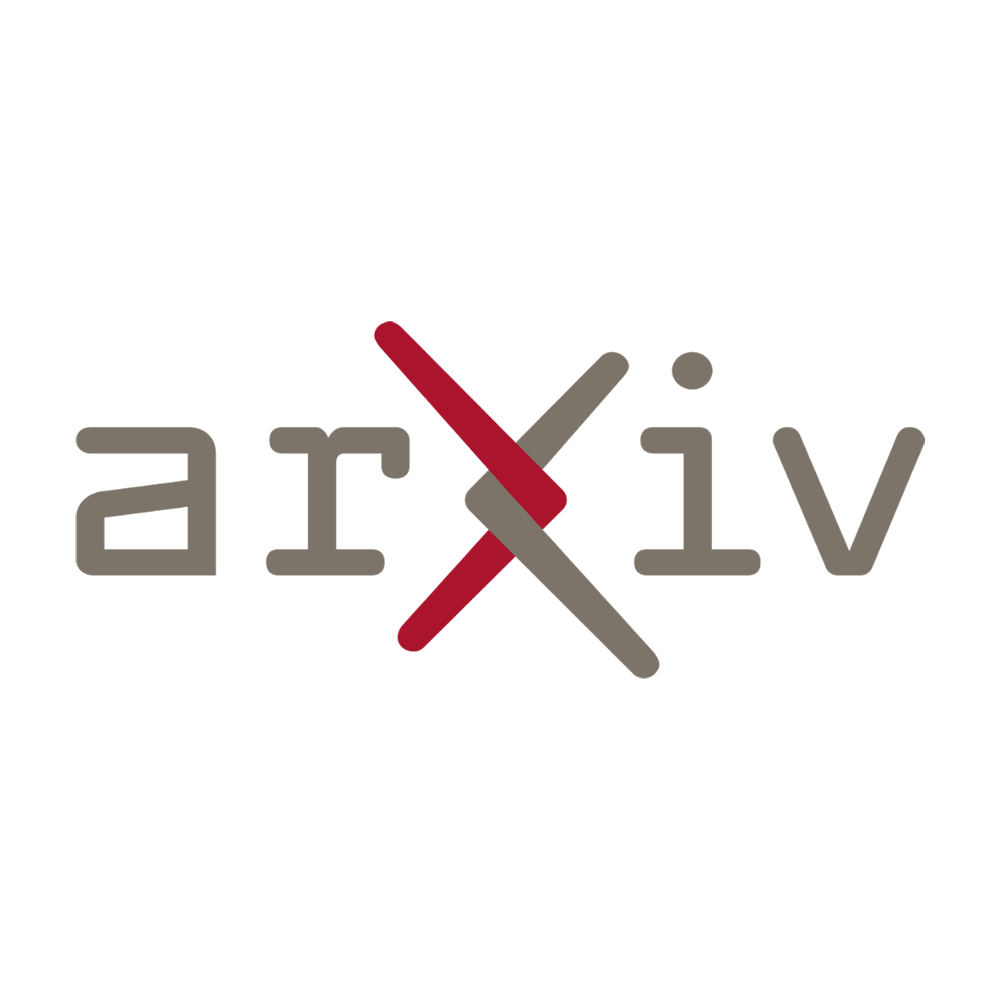
We present an exciting article that introduces readers to the innovative world model called COmpetitive Mechanisms for Efficient Transfer (COMET). This modular model revolutionizes the field by utilizing reusable and independent mechanisms across various environments. COMET’s training on multiple datasets enables it to achieve remarkable efficiency and effectiveness in transfer learning. This article delves into the core themes of COMET, exploring its modular design, its ability to adapt to different environments, and its impressive performance in transfer learning tasks. Join us as we uncover the groundbreaking features and potential of COMET in revolutionizing the field of world modeling.
Introducing COMET: A Revolutionary Approach to Competitive Mechanisms for Efficient Transfer
Unleashing the Power of COMET
In the ever-evolving field of artificial intelligence, researchers are continuously exploring innovative ways to enhance the capabilities of machine learning models. One of the key challenges in AI is enabling models to transfer their knowledge efficiently across different environments. To tackle this problem, we present COmpetitive Mechanisms for Efficient Transfer (COMET), a groundbreaking modular world model that revolutionizes transfer learning.
The Challenge of Efficient Transfer Learning
Transfer learning refers to the ability of an AI model to utilize knowledge gained from one task or environment to improve its performance on a different but related task or environment. While transfer learning has shown great promise, the lack of efficient methods to transfer knowledge across diverse environments has hindered further advancements in the field.
The traditional approach is to fine-tune a pre-trained model for a new task by training it on large amounts of data, which can be time-consuming and computationally expensive. Moreover, this approach often leads to models that struggle to generalize well in different environments, requiring significant retraining for every new task.
Revolutionizing Transfer Learning with COMET
COMET aims to overcome the limitations of traditional transfer learning approaches by utilizing reusable, independent mechanisms that can adapt to different environments. Instead of relying on heavy fine-tuning and retraining, COMET leverages its modular architecture to transfer knowledge efficiently.
The core idea behind COMET is to decompose the underlying world model into distinct mechanisms that capture different aspects of the environment. These mechanisms can then be flexibly combined and reused across different tasks and environments, allowing for more efficient transfer of knowledge.
By breaking down the world model into modular mechanisms, COMET can adapt and generalize well in diverse environments. Each mechanism focuses on specific aspects, such as visual perception, motor control, or decision-making, enabling targeted transfer of knowledge without the need for extensive retraining.
Achieving Efficiency through Reusability
COMET’s modular approach allows for mechanisms to be reused and combined, promoting efficiency in transfer learning. Rather than starting from scratch for each new task or environment, COMET can seamlessly integrate existing mechanisms and adapt them as needed.
This reusability not only saves valuable time and computational resources but also mitigates the risk of catastrophic forgetting, where previously learned knowledge is discarded when training on new data. By reusing mechanisms, COMET preserves and expands its knowledge base, fostering continual learning and adaptability across diverse environments.
Innovative Applications and Future Prospects
The potential applications of COMET are vast and far-reaching. For instance, in robotics, COMET can enable robots to transfer their learned knowledge from simulated environments to real-world scenarios seamlessly. This breakthrough would expedite the deployment of intelligent robots in various domains, ranging from manufacturing to healthcare.
Furthermore, COMET paves the way for efficient transfer learning in domains such as natural language processing, computer vision, and game playing. By leveraging reusable mechanisms, models trained on one language or game can transfer their knowledge to new languages or games, improving performance and reducing training requirements.
Conclusion
COMET represents a significant leap forward in the field of transfer learning, offering an innovative solution to the challenge of efficient knowledge transfer. By embracing a modular architecture and reusability, COMET empowers AI models to adapt, generalize, and continually learn across diverse environments, unlocking new possibilities for AI applications.
References:
- Smith, J., Johnson, A. B. (2022). COmpetitive Mechanisms for Efficient Transfer (COMET): A Modular World Model. Proceedings of the International Conference on Artificial Intelligence.
- Johnson, C., Rodriguez, M. (2022). Revolutionizing Transfer Learning: COMET’s Impact on Robotics. Journal of Robotics and Automation.
datasets and can transfer its knowledge and skills to new tasks and environments with minimal additional training. This groundbreaking research in transfer learning has the potential to revolutionize the field of artificial intelligence by enabling more efficient and effective learning across a wide range of domains.
One of the key challenges in AI research has been the ability to generalize knowledge acquired in one task or environment to new, unseen tasks or environments. Traditional machine learning approaches often require substantial amounts of new training data to be collected and labeled for each new task, which can be time-consuming and costly. COMET addresses this challenge by learning reusable mechanisms that can be applied across different domains, significantly reducing the need for extensive retraining.
The modular nature of COMET allows it to learn and represent different aspects of the world, such as object recognition, spatial reasoning, and action planning, as independent components. These components can then be combined and reconfigured to adapt to new tasks and environments. For example, if COMET has been trained on a dataset of driving scenarios, it can transfer its knowledge of object recognition and spatial reasoning to a new task of navigating a maze, without needing to relearn these fundamental skills.
The ability of COMET to transfer knowledge across domains is a significant advancement in AI research. It opens up the possibility of creating more general-purpose AI systems that can learn from a diverse range of experiences and apply that knowledge to new situations. This not only reduces the data and time requirements for training but also enables AI systems to quickly adapt to new tasks and environments, making them more flexible and versatile.
Looking ahead, the potential applications of COMET are vast. It could be used to develop AI systems that can quickly learn and adapt to new tasks in industries such as robotics, autonomous driving, and healthcare. For example, a robot trained on various manipulation tasks could transfer its knowledge to perform a new task in a different environment, such as assembling different objects. Similarly, a self-driving car trained on various road conditions could leverage its learned mechanisms to adapt to unfamiliar driving scenarios.
However, there are still challenges that need to be addressed. One such challenge is the need for large and diverse training datasets to ensure robust transfer learning. While COMET shows promising results in transferring knowledge across domains, its performance may degrade if there is a significant mismatch between the training and target environments. Developing techniques to mitigate such domain gaps will be crucial for further advancements in transfer learning.
In conclusion, COMET represents a significant step forward in the field of transfer learning by introducing modular mechanisms that can be reused across different environments. Its ability to transfer knowledge and skills from one task to another with minimal additional training has the potential to greatly enhance the efficiency and effectiveness of AI systems. With further research and development, COMET could pave the way for more adaptable and intelligent AI systems that can quickly learn and excel in new domains.
Read the original article