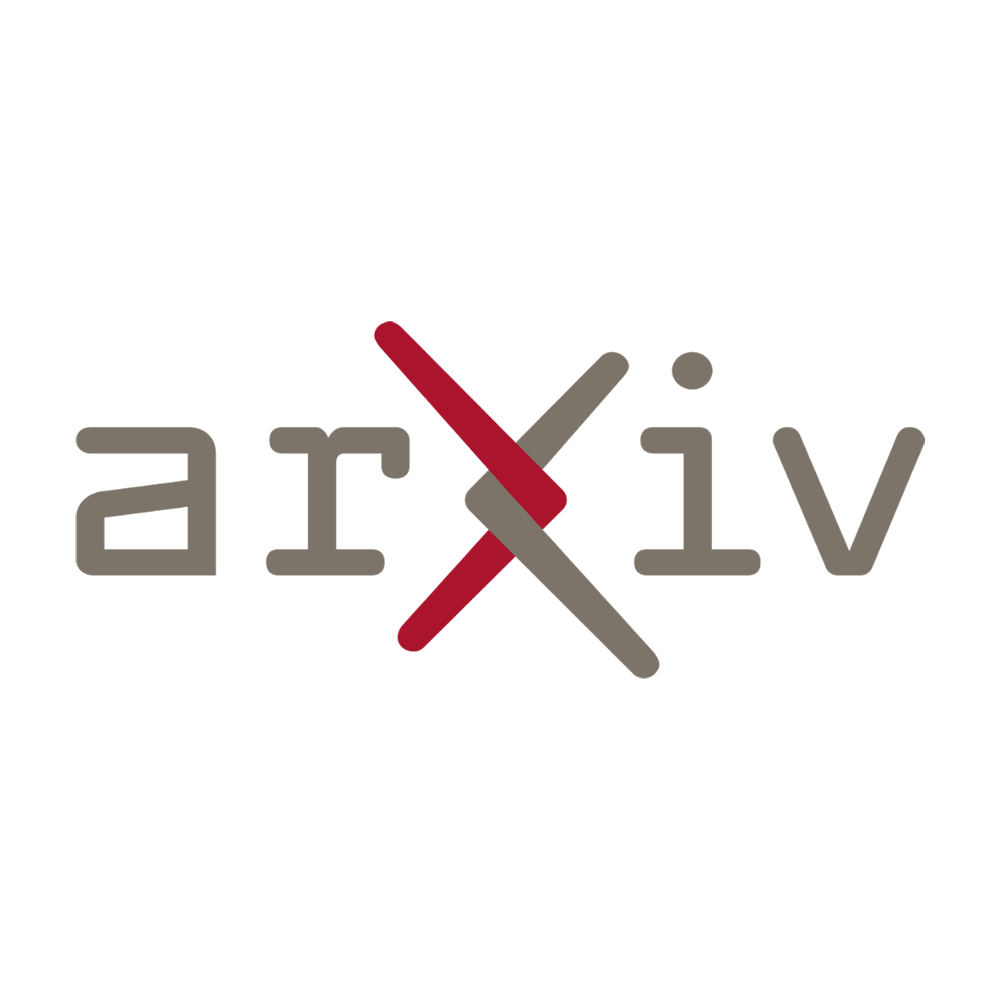
In the realm of 3D object detection, recent advancements in self-training techniques have brought about significant enhancements in unsupervised domain adaptation (UDA). These techniques primarily rely on the utilization of pseudo labels, specifically 3D boxes, to bridge the gap between labeled and unlabeled data. By harnessing the power of self-training, these methods have revolutionized the field of 3D UDA, paving the way for more accurate and efficient detection of objects in three-dimensional space.
Unleashing the Potential of Unsupervised Domain Adaptation for 3D Object Detection
Recent advancements in self-training techniques have brought remarkable improvements in unsupervised domain adaptation (UDA) for 3D object detection. These innovative methods typically rely on the use of pseudo labels, generating 3D boxes that mimic the actual object annotations. However, there is much more to explore and uncover within this emerging field.
Exploring the Underlying Themes
When delving into the realm of UDA for 3D object detection, two prevalent themes emerge – domain adaptation and self-training.
Domain Adaptation: Domain adaptation refers to the process of transferring knowledge from a source domain (where labeled data is available) to a target domain (where labeled data is scarce or nonexistent). The goal is to bridge the domain gap and enable accurate predictions in the target domain.
Self-Training: Self-training techniques leverage the output of a trained model to generate pseudo labels for unlabeled data. These pseudo labels are then used to refine the model iteratively, gradually improving its performance on the target domain.
By exploring these themes, researchers have developed promising approaches that push the boundaries of UDA for 3D object detection.
Innovative Solutions and Ideas
Building upon the existing techniques, we propose the following innovative solutions and ideas to further enhance UDA for 3D object detection:
- Integrating Multi-Modal Data: Incorporating multiple data modalities, such as RGB images, LiDAR point clouds, and depth maps, can provide richer information for domain adaptation. By fusing these modalities effectively, the model can better understand the target domain and improve detection accuracy.
- Adaptive Pseudo Labeling: Rather than treating all pseudo labels equally, exploring adaptive pseudo labeling strategies can help in prioritizing the labeling of challenging instances or uncertain predictions. This adaptive approach can lead to more robust models with improved generalization capabilities.
- Domain-Specific Optimization: Fine-tuning the model’s architecture and parameters specifically for the target domain can maximize performance. By adapting the model to the target domain’s characteristics, it can effectively handle domain-specific challenges and nuances.
These solutions aim to address some of the limitations and challenges faced by current UDA techniques, ultimately paving the way for more accurate and versatile 3D object detection systems.
Conclusion
Unsupervised domain adaptation for 3D object detection is a rapidly evolving field, driven by innovative self-training techniques. By exploring the underlying themes of domain adaptation and self-training, researchers have achieved impressive results. However, there is still a vast landscape of possibilities waiting to be explored.
By integrating multi-modal data, employing adaptive pseudo labeling strategies, and fine-tuning the model specifically for the target domain, we can unlock the full potential of UDA for 3D object detection. These solutions offer innovative ways to overcome challenges and further advance the field, ultimately leading to smarter and more reliable 3D object detection systems.
based on the predictions made by a pre-trained model on unlabeled target domain data. This process allows the model to learn from the unlabeled data and adapt to the target domain without requiring any labeled data in that domain.
The use of self-training techniques in unsupervised domain adaptation for 3D object detection is a promising approach. Traditionally, domain adaptation has relied on labeled data from the target domain, which can be expensive and time-consuming to obtain. By leveraging unlabeled data and generating pseudo labels, self-training techniques offer a more cost-effective and scalable solution.
One key advantage of self-training techniques is their ability to exploit the inherent structures and patterns present in the unlabeled data. The pre-trained model’s predictions on this data can be used to generate pseudo labels, which provide supervision for the model during the adaptation process. This allows the model to align its understanding of 3D objects with the target domain, even without explicit labels.
However, there are challenges associated with self-training techniques for 3D UDA. One major challenge is the quality of the pseudo labels. Since these labels are generated based on the predictions of the pre-trained model, they may not always accurately represent the true annotations. This can lead to errors and inconsistencies in the adaptation process, affecting the overall performance of the model.
To address this issue, researchers are exploring various strategies to improve the quality of pseudo labels. One approach is to incorporate uncertainty estimation into the self-training process. By considering the uncertainty of the pre-trained model’s predictions, the model can assign higher confidence to reliable pseudo labels and discard or reevaluate uncertain ones. This helps in reducing the impact of incorrect pseudo labels on the adaptation process.
Another area of research focuses on refining the initial pseudo labels through iterative training. Instead of relying solely on the initial pseudo labels, the model can be trained on the adapted data and then generate new pseudo labels based on the updated predictions. This iterative process can help in refining the pseudo labels over time, leading to improved adaptation performance.
Looking ahead, the future of self-training techniques in unsupervised domain adaptation for 3D object detection seems promising. As researchers continue to explore ways to enhance the quality of pseudo labels and improve the adaptation process, we can expect further advancements in this field. Additionally, the combination of self-training techniques with other approaches, such as domain-specific augmentation or meta-learning, could unlock even higher performance gains in 3D UDA.
Read the original article