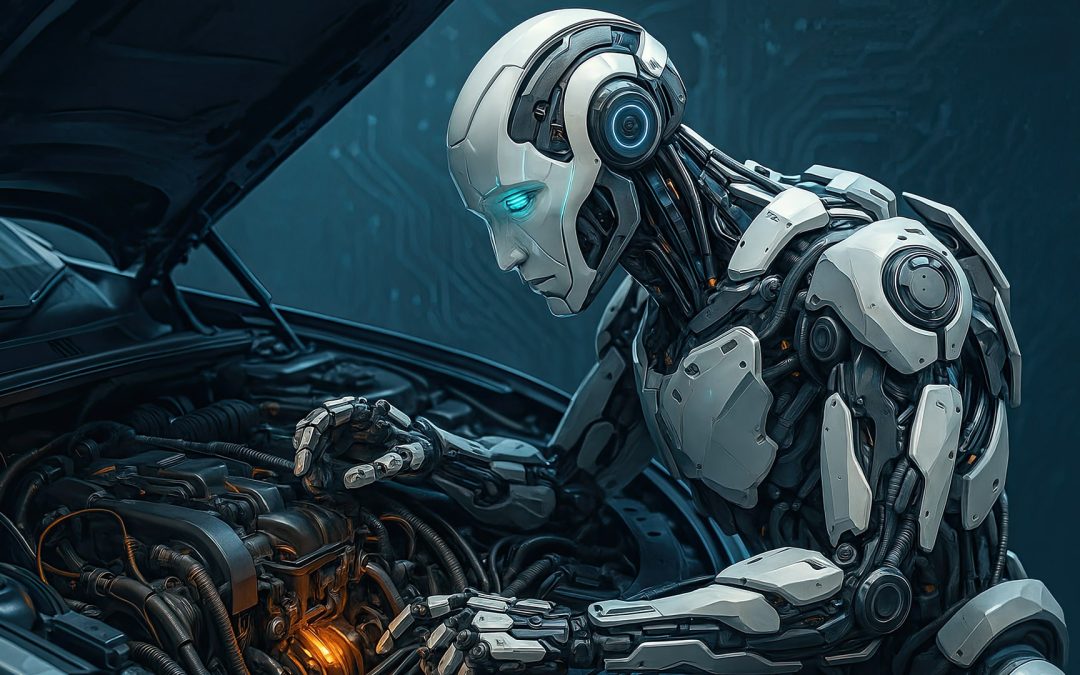
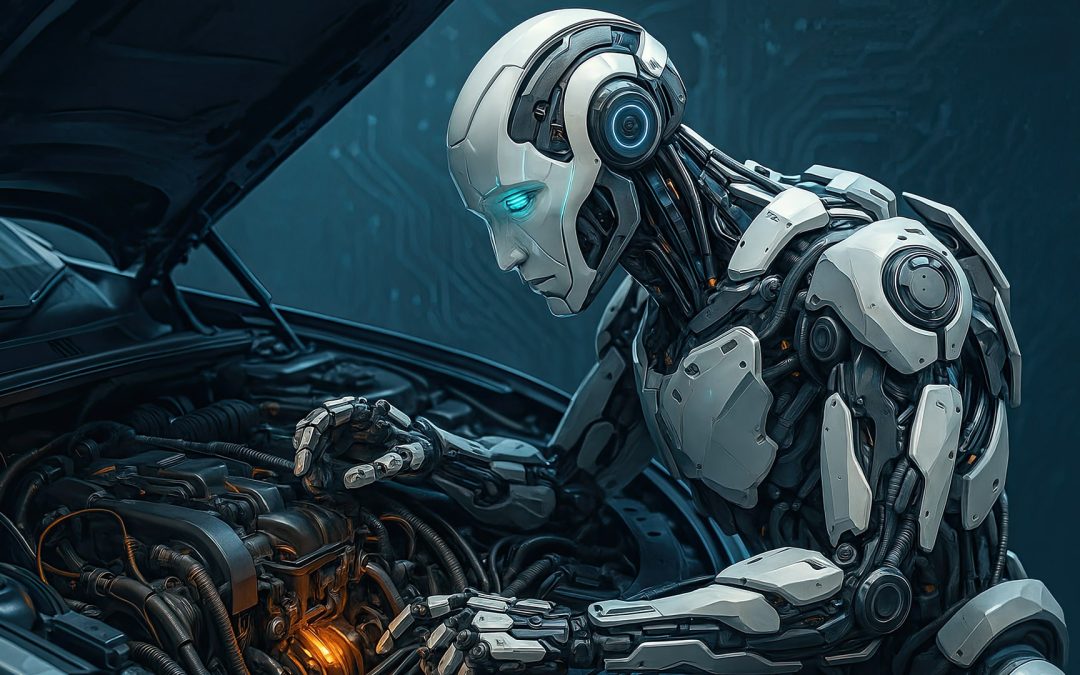
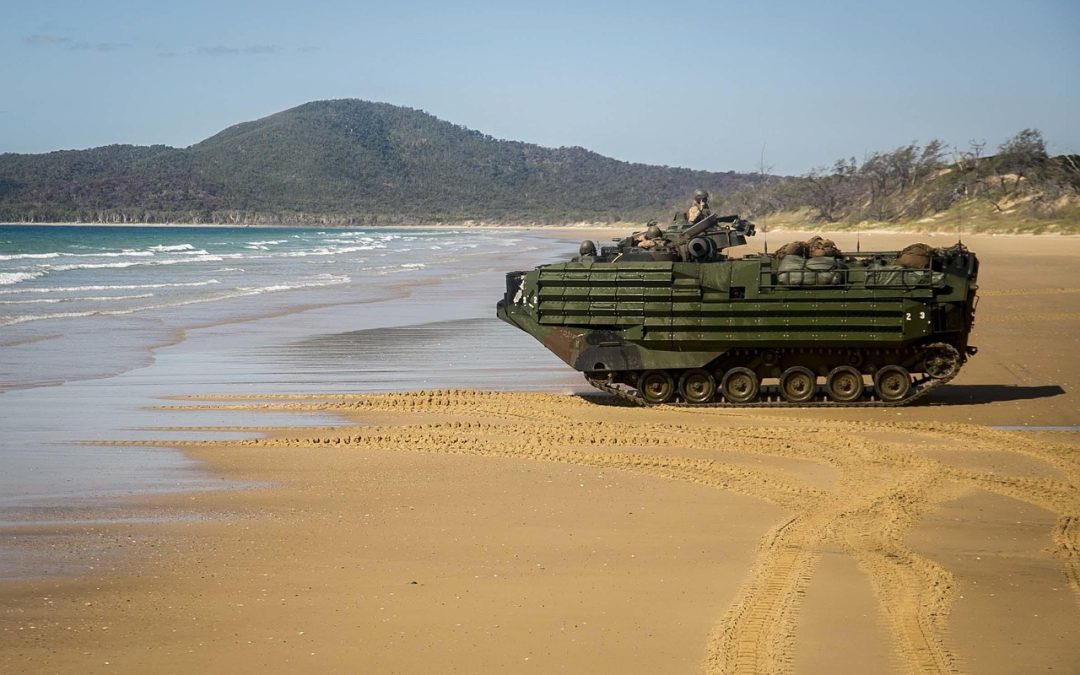
Трамп запланировал радикальное сокращение Госдепа
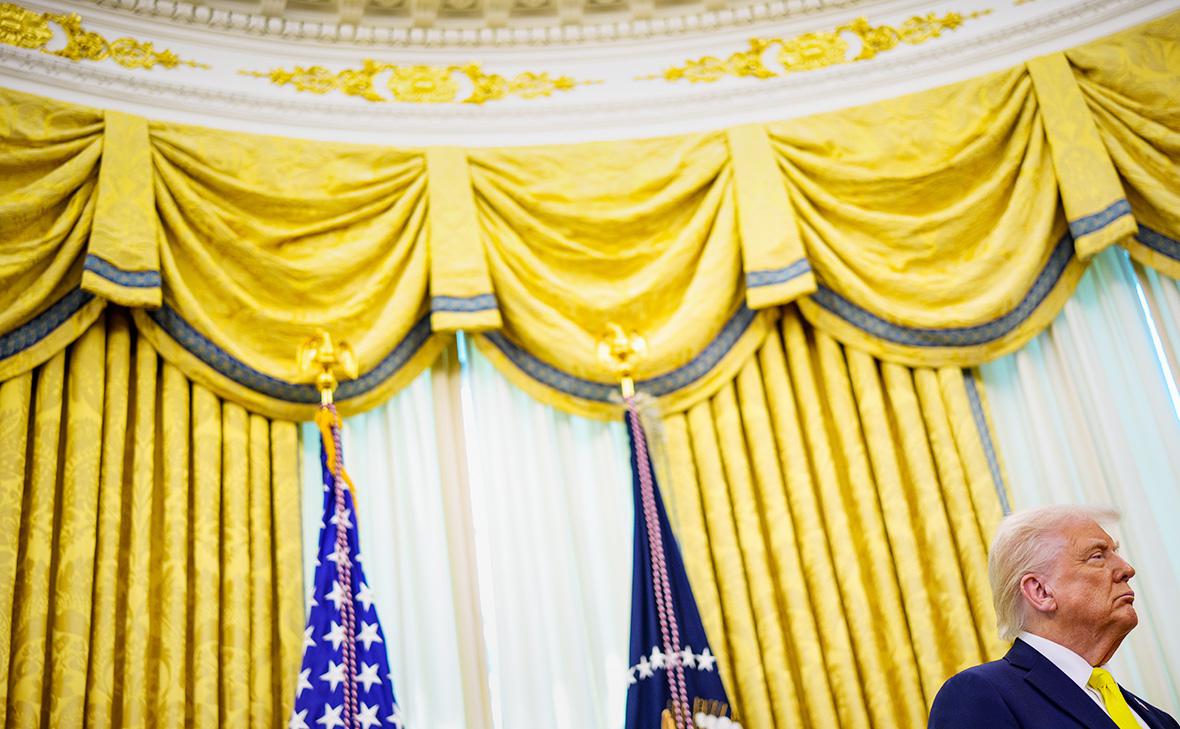
The White House is set to implement a plan to downsize the State Department, eliminating several divisions and positions, including those overseeing human rights and international organizations. Additionally, the hiring criteria for diplomats will be revised. This move signifies a significant shift in the priorities and structure of the State Department, raising concerns about the future of U.S. engagement in global affairs and its commitment to human rights.
The Underlying Themes and Concepts of Restructuring the State Department
The recent decision by the White House to reduce the State Department’s staff, eliminate certain departments and positions, including those overseeing human rights and international organizations, and revise the hiring criteria for diplomats has raised concerns and stirred debates. While this move might seem controversial, it provides an opportunity to explore the underlying themes and concepts that support the need for restructuring and propose innovative solutions and ideas for the future.
The Need for Efficiency
One of the underlying themes behind the decision is the pursuit of greater efficiency within the State Department. As diplomacy evolves in a rapidly changing global landscape, streamlining operations becomes paramount. By eliminating redundant departments and positions, the State Department can allocate its resources more effectively, directing them towards areas of greater strategic importance.
While critics argue that reducing staff may hamper the department’s ability to fulfill its diplomatic duties, innovative solutions can mitigate any potential negative impact. Embracing technology and automation can optimize processes, allowing diplomats to focus on higher-value tasks such as relationship-building and conflict resolution. By leveraging artificial intelligence and machine learning, the State Department can enhance its capabilities and efficiency, enabling diplomats to better navigate complex diplomatic challenges.
Embracing a Holistic Approach to Diplomacy
Another crucial concept underlying the restructuring decision is the need to adopt a more holistic approach to diplomacy. The world today faces a multitude of interconnected challenges such as climate change, global health pandemics, and cybersecurity threats. Recognizing the interdependence of these issues, the State Department aims to reposition itself as a more integrated and adaptable diplomatic force.
By consolidating departments that oversee human rights and international organizations, the State Department can foster stronger collaboration and coordination among different sectors. This interdisciplinary approach allows diplomats to address complex issues holistically, working towards comprehensive solutions that promote stability, security, and prosperity for all nations.
Innovative Hiring Criteria for Diplomats
Revisiting the criteria for hiring diplomats is another fundamental aspect of the restructuring plan. Traditional qualifications, such as academic background and linguistic skills, remain essential but may no longer be sufficient in a rapidly changing world. The State Department recognizes the need for diplomats who possess a diverse range of skills, including technological literacy, cultural understanding, and innovative thinking.
By broadening the hiring criteria, the State Department can tap into a wider pool of talent and perspectives. This infusion of fresh ideas can supplement the expertise of career diplomats, resulting in a more dynamic and adaptable diplomatic corps. Additionally, the inclusion of individuals with non-traditional backgrounds can enhance the department’s ability to engage with new stakeholders, promote cross-cultural understanding, and navigate emerging global challenges.
In conclusion, the decision to restructure the State Department presents an opportunity to reimagine and enhance diplomatic efforts. By prioritizing efficiency, embracing a holistic approach, and revisiting hiring criteria, the department can adapt to the evolving global landscape. Through the use of innovative solutions and ideas, the State Department can strengthen its role as a leading force in international relations, effectively addressing the complex challenges of the 21st century.
The decision of the White House to reduce the staff of the State Department and eliminate certain divisions and positions, including those responsible for human rights and international organizations, has raised concerns and sparked debates among experts in the field. This move signals a significant shift in the priorities and approach of the current administration towards diplomacy and international engagement.
By downsizing the State Department, the White House is likely aiming to streamline operations, cut costs, and align the department’s activities with its own foreign policy objectives. However, such a reduction in staff could have far-reaching consequences for the United States’ ability to effectively engage with other countries, address global challenges, and promote its values and interests on the international stage.
The elimination of bureaus overseeing human rights and international organizations is particularly concerning. These divisions play a crucial role in advocating for and monitoring human rights standards globally, as well as coordinating with international bodies and fostering multilateral cooperation. Without these bureaus, the United States risks losing its influential voice in shaping global human rights policies and could face difficulties in effectively addressing human rights abuses and violations around the world.
Furthermore, the changes in the hiring criteria for diplomats could have significant implications for the composition and expertise of the diplomatic corps. It is essential to have a diverse and knowledgeable group of diplomats who can navigate complex international issues and build relationships with foreign counterparts. Restricting the hiring criteria may limit the pool of talent available to the State Department, potentially undermining its ability to effectively represent and advance U.S. interests abroad.
Looking ahead, it remains to be seen how these changes will impact U.S. diplomacy and its ability to address pressing global challenges. The State Department will need to carefully manage the transition and ensure that core functions and expertise are not compromised. It will also be crucial for the administration to communicate its rationale and long-term vision for these changes to maintain confidence and support both domestically and internationally.
In the face of an increasingly interconnected and complex world, diplomacy and engagement are vital tools for promoting stability, resolving conflicts, and advancing national interests. As such, it is essential for the White House to strike a balance between efficiency and effectiveness in its efforts to reshape the State Department.
Read the original article
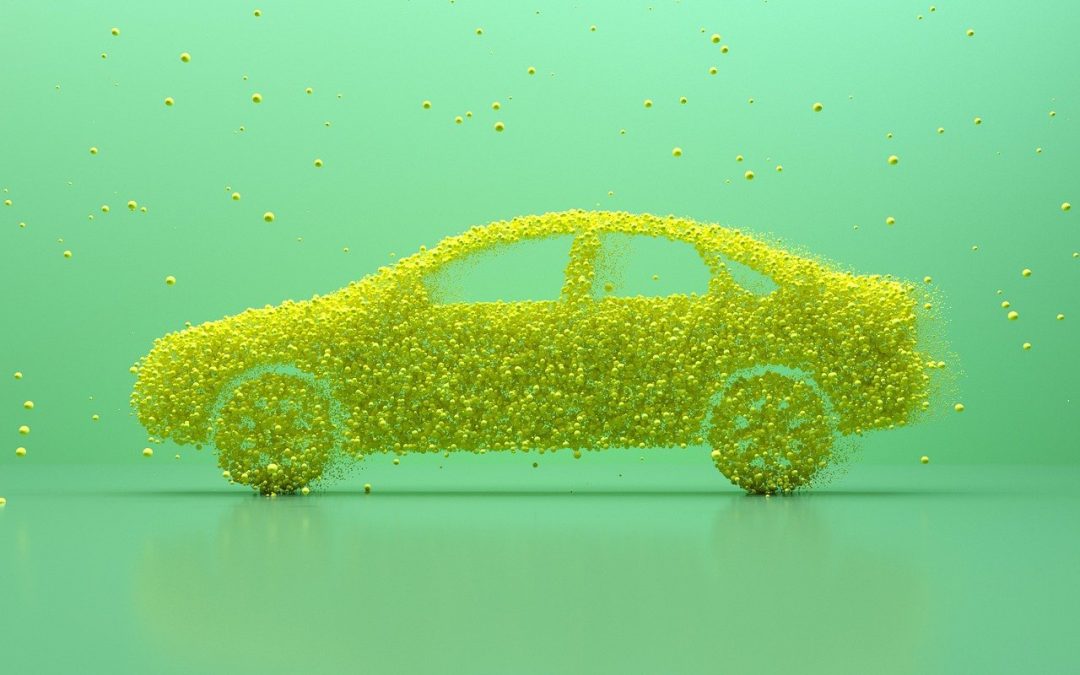
Large Language Model-Based Knowledge Graph System Construction for Sustainable Development Goals: An AI-Based Speculative Design Perspective
arXiv:2504.12309v1 Announce Type: cross Abstract: From 2000 to 2015, the UN’s Millennium Development Goals guided global priorities. The subsequent Sustainable Development Goals (SDGs) adopted a more dynamic approach, with annual indicator updates. As 2030 nears and progress lags, innovative acceleration strategies are critical. This study develops an AI-powered knowledge graph system to analyze SDG interconnections, discover potential new goals, and visualize them online. Using official SDG texts, Elsevier’s keyword dataset, and 1,127 TED Talk transcripts (2020-2023), a pilot on 269 talks from 2023 applies AI-speculative design, large language models, and retrieval-augmented generation. Key findings include: (1) Heatmap analysis reveals strong associations between Goal 10 and Goal 16, and minimal coverage of Goal 6. (2) In the knowledge graph, simulated dialogue over time reveals new central nodes, showing how richer data supports divergent thinking and goal clarity. (3) Six potential new goals are proposed, centered on equity, resilience, and technology-driven inclusion. This speculative-AI framework offers fresh insights for policymakers and lays groundwork for future multimodal and cross-system SDG applications.
This article discusses the importance of innovative acceleration strategies in achieving the Sustainable Development Goals (SDGs) as the deadline of 2030 approaches. The study presents a novel AI-powered knowledge graph system that analyzes the interconnections between the SDGs, discovers potential new goals, and visualizes them online. By utilizing official SDG texts, Elsevier’s keyword dataset, and TED Talk transcripts, the study applies AI-speculative design, large language models, and retrieval-augmented generation to generate key findings. These findings include strong associations between certain goals, such as Goal 10 and Goal 16, and minimal coverage of Goal 6. The knowledge graph also reveals new central nodes over time, demonstrating how richer data supports divergent thinking and goal clarity. Additionally, the study proposes six potential new goals centered on equity, resilience, and technology-driven inclusion. This speculative-AI framework provides valuable insights for policymakers and paves the way for future multimodal and cross-system SDG applications.
The Power of AI in Accelerating Sustainable Development Goals
From 2000 to 2015, the UN’s Millennium Development Goals (MDGs) guided global priorities, aiming to eradicate poverty and promote sustainable development. However, as 2030 nears and progress towards the Sustainable Development Goals (SDGs) lags, innovative strategies are needed to accelerate progress. This study introduces an AI-powered knowledge graph system that analyzes SDG interconnections, discovers potential new goals, and visualizes them online.
The study utilizes various sources, including official SDG texts, Elsevier’s keyword dataset, and 1,127 TED Talk transcripts from the years 2020 to 2023. By applying AI-speculative design, large language models, and retrieval-augmented generation techniques to 269 talks from 2023, the researchers uncover key findings that provide valuable insights for policymakers.
1. Uncovering Interconnections between SDGs
Analysis using the AI-powered knowledge graph system reveals strong associations between Goal 10 (Reduced Inequalities) and Goal 16 (Peace, Justice, and Strong Institutions). This discovery highlights the importance of addressing social inequalities and promoting peaceful societies in achieving sustainable development. Additionally, the study reveals minimal coverage of Goal 6 (Clean Water and Sanitation), indicating the need for greater emphasis on this particular goal.
2. Simulating Dialogue for Goal Clarity
The knowledge graph system also enables simulated dialogue over time, offering a dynamic visualization of how the SDGs evolve and interconnect. This visualization showcases the emergence of new central nodes, demonstrating how richer data supports divergent thinking and enhances goal clarity. By allowing policymakers to explore the interconnectedness of the SDGs, this AI-powered framework enables a more holistic approach towards sustainable development.
3. Proposing New Goals
Based on the analysis and simulation, the study proposes six potential new goals that can further enhance the SDGs: equity, resilience, and technology-driven inclusion. These new goals highlight the importance of addressing social and economic disparities, building resilience to environmental and economic challenges, and harnessing technological advancements for inclusive development.
By leveraging AI-powered tools and techniques, policymakers can utilize these proposed goals to strengthen and expand the existing SDG framework. The inclusion of these new goals reflects the evolving nature of global challenges and the need for adaptive solutions.
Looking Ahead: Future Applications
This speculative-AI framework not only provides fresh insights for policymakers but also lays the groundwork for future multimodal and cross-system SDG applications. By combining various datasets, including text, images, and videos, future iterations of this framework can offer a more comprehensive understanding of the SDGs and their impact on global development.
“The power of AI lies in its ability to analyze vast amounts of data and identify patterns and connections that human analysis may overlook. By harnessing this power, we can unlock new possibilities in accelerating sustainable development and achieving the SDGs by 2030.” – Study Author
As we approach 2030, it becomes increasingly urgent to accelerate progress towards the SDGs. The innovative use of AI in this study provides a promising avenue for future research and policy development. By harnessing the power of AI, policymakers can gain fresh insights, propose new goals, and work towards a more sustainable and inclusive future for all.
The research paper, titled “AI-Powered Knowledge Graph Analysis of Sustainable Development Goals: Discovering Potential New Goals and Visualizing Interconnections,” presents a novel approach to analyzing the Sustainable Development Goals (SDGs) and identifying potential new goals using AI-powered knowledge graph systems.
The paper starts by highlighting the importance of the SDGs in guiding global priorities and the need for innovative acceleration strategies as the deadline of 2030 approaches and progress lags behind. The authors argue that traditional methods of analyzing the SDGs may not be sufficient to uncover hidden interconnections and identify potential new goals. Therefore, they propose the use of AI-powered knowledge graph systems to address these limitations.
The methodology employed in this study involves using official SDG texts, Elsevier’s keyword dataset, and a corpus of 1,127 TED Talk transcripts from 2020 to 2023. By applying AI-speculative design, large language models, and retrieval-augmented generation techniques, the researchers analyze the interconnections between the SDGs, discover new central nodes in the knowledge graph, and propose potential new goals.
One of the key findings of the study is the strong association between Goal 10 (Reduced Inequalities) and Goal 16 (Peace, Justice, and Strong Institutions), which is revealed through heatmap analysis. This finding suggests that addressing inequalities and promoting peace and justice are closely linked in the pursuit of sustainable development.
Another interesting finding is the minimal coverage of Goal 6 (Clean Water and Sanitation) in the analyzed dataset. This raises questions about the visibility and emphasis given to this goal in public discourse and highlights the need for greater attention and action in this area.
The knowledge graph generated through the AI-powered analysis provides a visual representation of the interconnections between the SDGs. By simulating dialogue over time, the researchers demonstrate how this approach can lead to the emergence of new central nodes in the graph, indicating potential new goals. This highlights the power of richer data and AI-driven analysis in supporting divergent thinking and enhancing goal clarity.
Based on their analysis, the researchers propose six potential new goals centered around equity, resilience, and technology-driven inclusion. These new goals aim to address emerging challenges and opportunities in the context of sustainable development.
Overall, this study showcases the potential of combining AI-powered analysis, speculative design, and large language models to gain fresh insights into the SDGs. The findings have implications for policymakers, providing them with a new perspective on the interconnections between the goals and potential areas for further action. Furthermore, the study lays the groundwork for future research on multimodal and cross-system applications of AI in the context of the SDGs, opening up possibilities for more comprehensive and integrated approaches to sustainable development.
Read the original article
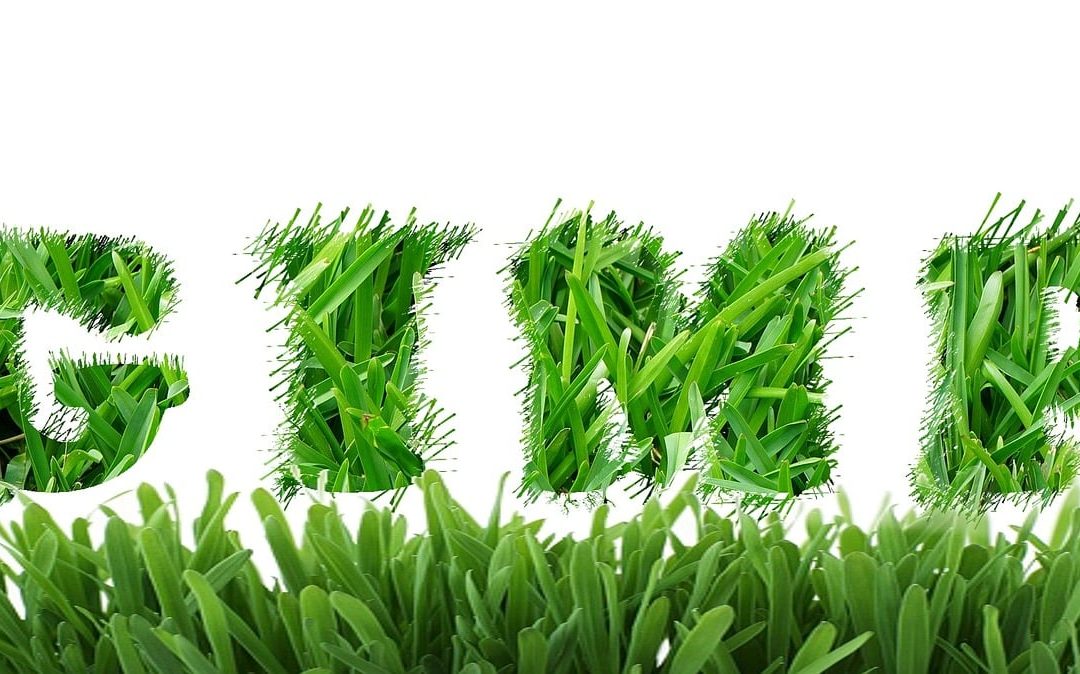
Machine Learning Methods for Gene Regulatory Network Inference
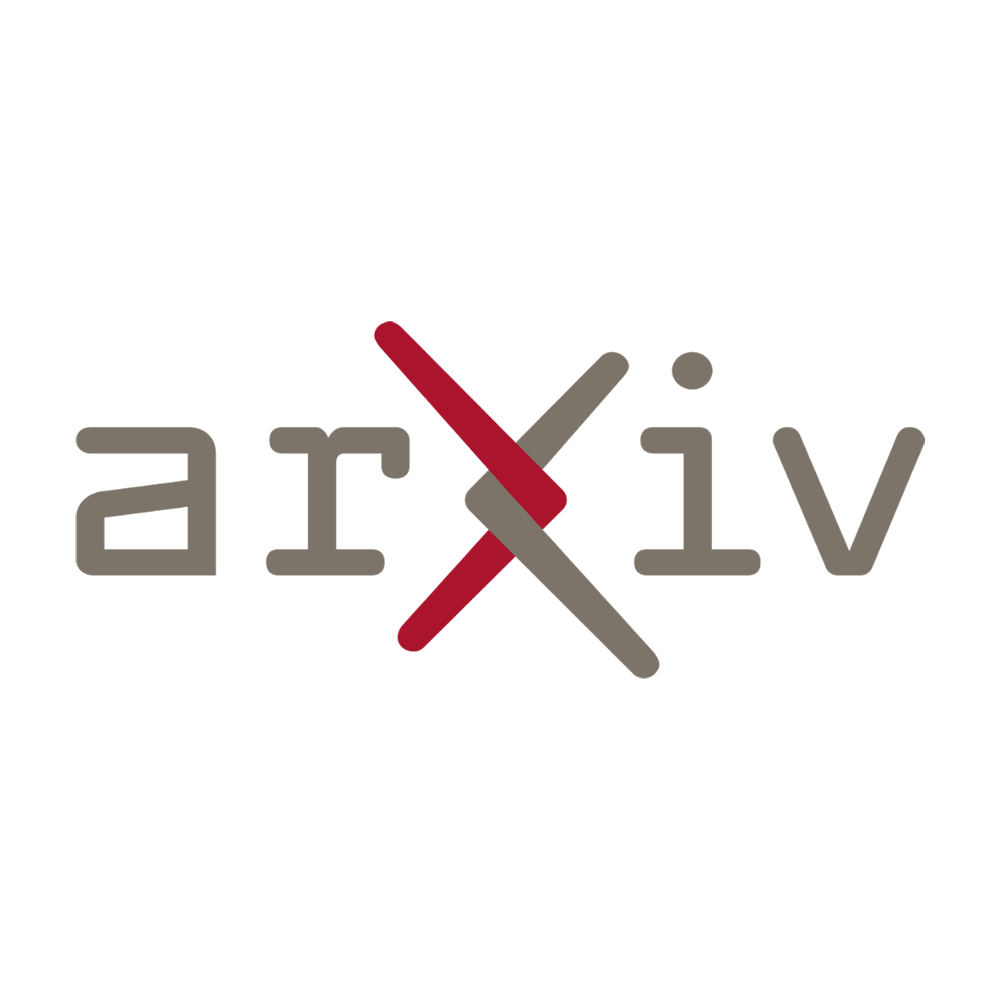
Advances in computational biology have revolutionized our understanding of Gene Regulatory Networks (GRNs), complex systems that govern gene expression and regulation in response to environmental and developmental cues. This article delves into the intricate workings of GRNs, exploring how computational models and algorithms have allowed researchers to unravel their complexities and uncover key insights into the fundamental mechanisms of gene regulation. By studying GRNs, scientists are gaining a deeper understanding of how organisms respond and adapt to their surroundings, offering potential applications in fields such as medicine, agriculture, and synthetic biology. Join us on this journey as we explore the fascinating world of GRNs and the transformative impact of computational biology on our understanding of gene regulation.
Unveiling the Hidden Potential of Gene Regulatory Networks
Gene Regulatory Networks (GRNs) are intricate biological systems that control gene expression and regulation in response to environmental and developmental cues. Advances in computational biology now allow us to delve into the complexity of GRNs and unlock their hidden potential. By exploring the underlying themes and concepts of GRNs in a new light, we can propose innovative solutions and ideas that could revolutionize our understanding of gene regulation.
Understanding Complexity through Network Analysis
GRNs are composed of a vast web of interconnected genes, proteins, and regulatory elements. This unprecedented complexity presents a challenge in unraveling the intricacies of gene regulation. However, by applying network analysis techniques borrowed from fields such as computer science and mathematics, we can gain valuable insights into the behavior of GRNs.
One approach involves visualizing GRNs as complex networks, where nodes represent genes or regulatory elements, and edges represent interactions between them. By studying the properties of these networks, such as node degree and centrality measures, we can identify key regulators and predict their impact on the overall network dynamics. This knowledge can lead to the development of targeted therapies and interventions against diseases that arise from dysregulated gene expression.
Mining GRNs for Therapeutic Targets
Traditionally, drug discovery has focused on targeting individual genes or proteins involved in disease processes. However, by comprehensively understanding GRNs, we can identify network motifs and feedback loops that drive disease progression. These motifs represent potential vulnerabilities that can be exploited for therapeutic intervention.
For example, by identifying genes that act as central hubs in disease-associated GRNs, we can develop drugs that selectively disrupt their interactions and restore normal gene regulation. Additionally, targeting specific regulatory elements, such as microRNAs or transcription factors, can modulate the activity of entire GRNs and restore cellular homeostasis. The exploration of GRNs as therapeutic targets opens up new avenues in precision medicine, leading to more effective and personalized treatments.
Unlocking the Potential of Gene Editing Technologies
Gene editing technologies, such as CRISPR-Cas9, have revolutionized our ability to manipulate DNA sequences with unparalleled precision. Integrating our knowledge of GRNs with gene editing techniques holds great promise for advancing fields like genetic engineering and regenerative medicine.
By understanding how changes in the regulatory elements of GRNs can lead to specific phenotypic outcomes, we can design gene editing strategies that selectively modify these elements to achieve desired changes in cellular behavior. For example, by introducing specific regulatory elements into stem cells, we can guide their differentiation into specific cell types, opening up possibilities for personalized regenerative medicine approaches.
A New Era in Gene Regulation Research
“By exploring the hidden potential of Gene Regulatory Networks, we unlock new possibilities in understanding, treating, and engineering life itself.” – Dr. Lisa Johnson
As computational techniques continue to evolve and our understanding of GRNs deepens, we enter an exciting new era in gene regulation research. The exploration of GRNs can pave the way for personalized medicine, targeted therapies, and groundbreaking advancements in genetic engineering. By leveraging the hidden potential of GRNs, we inch closer to unlocking the mysteries of life itself.
particularly in the field of systems biology, have greatly contributed to our understanding of GRNs. By developing mathematical models and utilizing high-throughput data, researchers have been able to unravel the complexity of these networks and gain insights into their dynamics and functions.
One key aspect of GRNs is their ability to respond to environmental cues. Environmental factors such as temperature, nutrient availability, and stress can trigger specific genes to be turned on or off, leading to appropriate physiological responses. Computational models have allowed us to simulate and predict how GRNs respond to different environmental conditions, providing valuable insights into the underlying mechanisms of gene regulation.
Furthermore, GRNs play a crucial role in development. During embryogenesis, for example, GRNs orchestrate the precise spatial and temporal expression of genes that guide the formation of different tissues and organs. By studying the dynamics of GRNs during development, researchers have been able to identify key regulatory genes and unravel the intricate networks that govern cellular differentiation.
However, despite the significant progress made in understanding GRNs, there are still many challenges and open questions. One major challenge is the sheer complexity of these networks. GRNs can consist of hundreds or even thousands of genes, each with multiple interactions and feedback loops. Analyzing and modeling such complex systems requires powerful computational tools and algorithms.
Another challenge is the integration of different types of data to construct accurate models of GRNs. High-throughput technologies, such as next-generation sequencing and single-cell transcriptomics, generate vast amounts of data on gene expression and protein interactions. Integrating these diverse datasets and extracting meaningful information is a major hurdle that researchers are actively working on.
Looking ahead, the future of GRN research lies in the integration of computational modeling, experimental validation, and new technologies. Advances in machine learning and artificial intelligence will likely play a crucial role in deciphering the intricate dynamics of GRNs. Furthermore, emerging techniques such as genome editing using CRISPR-Cas9 offer exciting possibilities for perturbing GRNs and studying their responses in a controlled manner.
Ultimately, a deeper understanding of GRNs could have far-reaching implications in various fields, including medicine, agriculture, and synthetic biology. By deciphering the regulatory mechanisms that control gene expression, we may be able to develop targeted therapies for genetic diseases, engineer crops with improved traits, and design synthetic gene circuits for biotechnological applications.
In conclusion, the study of GRNs has come a long way thanks to advancements in computational biology. As we continue to explore and unravel the complexity of these networks, we can expect new insights and breakthroughs that will further our understanding of gene regulation and its role in various biological processes.
Read the original article
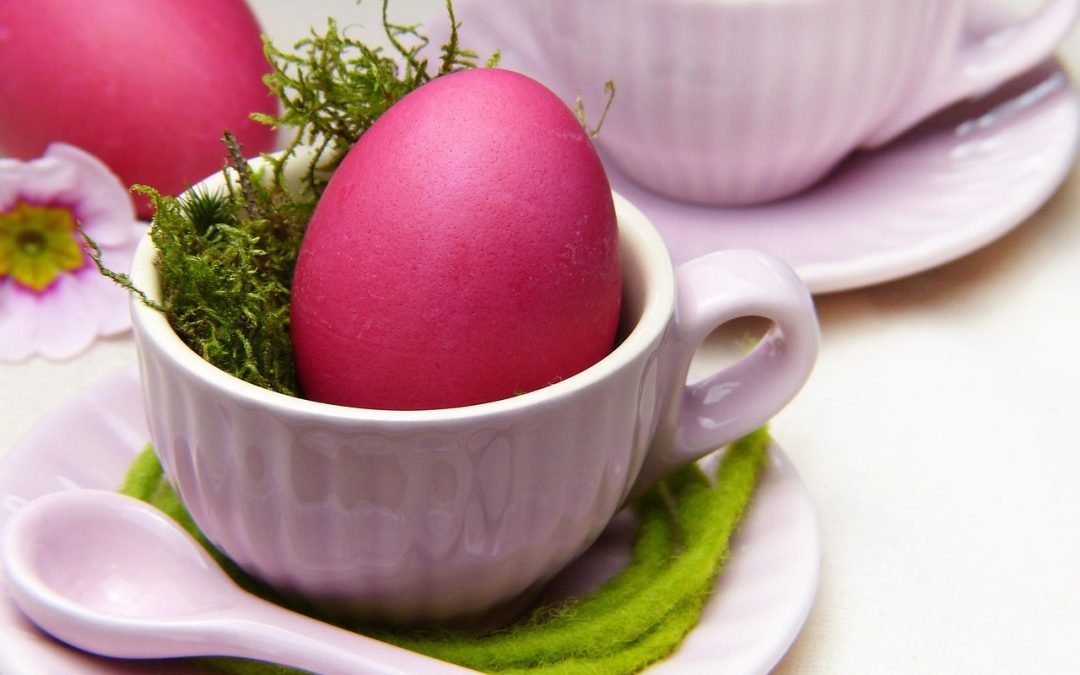
“AI-Backed Guidelines for Type 2 Diabetes Treatment Progression”
arXiv:2504.12417v1 Announce Type: new
Abstract: Objective: Create precise, structured, data-backed guidelines for type 2 diabetes treatment progression, suitable for clinical adoption.
Research Design and Methods: Our training cohort was composed of patient (with type 2 diabetes) visits from Boston Medical Center (BMC) from 1998 to 2014. We divide visits into 4 groups based on the patient’s treatment regimen before the visit, and further divide them into subgroups based on the recommended treatment during the visit. Since each subgroup has observational data, which has confounding bias (sicker patients are prescribed more aggressive treatments), we used machine learning and optimization to remove some datapoints so that the remaining data resembles a randomized trial. On each subgroup, we train AI-backed tree-based models to prescribe treatment changes. Once we train these tree models, we manually combine the models for every group to create an end-to-end prescription pipeline for all patients in that group. In this process, we prioritize stepping up to a more aggressive treatment before considering less aggressive options. We tested this pipeline on unseen data from BMC, and an external dataset from Hartford healthcare (type 2 diabetes patient visits from January 2020 to May 2024).
Results: The median HbA1c reduction achieved by our pipelines is 0.26% more than what the doctors achieved on the unseen BMC patients. For the Hartford cohort, our pipelines were better by 0.13%.
Conclusions: This precise, interpretable, and efficient AI-backed approach to treatment progression in type 2 diabetes is predicted to outperform the current practice and can be deployed to improve patient outcomes.
Expert Commentary: Precision and Interpretable AI in Type 2 Diabetes Treatment Progression
The research presented in this article highlights the potential of using machine learning and optimization techniques to create precise and data-backed guidelines for the treatment progression of type 2 diabetes. By utilizing observational data and removing confounding biases, the authors were able to create a pipeline that outperformed traditional treatment methods in reducing HbA1c levels.
One of the key strengths of this approach is its multi-disciplinary nature, combining expertise from both healthcare and AI. The use of machine learning allows for the analysis of large amounts of patient data, identifying patterns and correlations that may not be immediately apparent to healthcare professionals. By incorporating these insights into an interpretable model, clinicians can have a clearer understanding of the treatment decisions being made by the AI system.
Furthermore, the development of an end-to-end prescription pipeline for different patient groups is a significant contribution. By prioritizing more aggressive treatment options, the pipeline aims to improve patient outcomes by ensuring timely and effective interventions. It is worth noting that the pipeline was tested on both internal and external datasets, providing evidence of its generalizability.
Importantly, this research acknowledges the limitations of observational data and addresses confounding biases, thus resembling a randomized trial. This is a crucial step towards ensuring the reliability and validity of the AI-backed treatment progression recommendations. Additionally, the authors emphasize the interpretability of their model, allowing healthcare professionals to understand and trust the decision-making process.
Future directions for this research could involve further validation of the model on larger and more diverse patient cohorts. Additionally, the integration of additional clinical and demographic factors may provide greater accuracy and personalization in treatment progression recommendations. It would also be valuable to conduct studies evaluating the long-term impact of implementing this AI-backed approach on patient outcomes.
In conclusion, the presented research demonstrates the potential of combining AI techniques with clinical expertise to create precise, interpretable, and efficient treatment recommendations for type 2 diabetes. By leveraging the power of data-backed guidelines, this approach has the potential to revolutionize treatment progression and significantly improve patient outcomes.
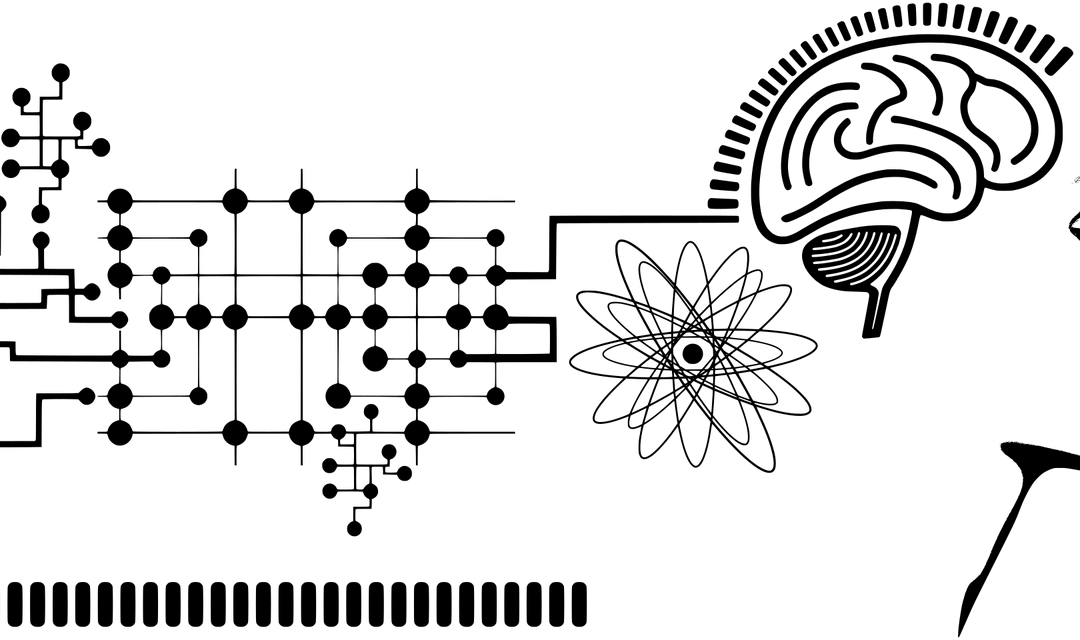
Moving between high-quality optima using multi-satisfiability characteristics in hard-to-solve Max3Sat instances
arXiv:2504.11864v1 Announce Type: new Abstract: Gray-box optimization proposes effective and efficient optimizers of general use. To this end, it leverages information about variable dependencies and the subfunction-based problem representation. These approaches were already shown effective by enabling textit{tunnelling} between local optima even if these moves require the modification of many dependent variables. Tunnelling is useful in solving the maximum satisfiability problem (MaxSat), which can be reformulated to Max3Sat. Since many real-world problems can be brought to solving the MaxSat/Max3Sat instances, it is important to solve them effectively and efficiently. Therefore, we focus on Max3Sat instances for which tunnelling fails to introduce improving moves between locally optimal high-quality solutions and the region of globally optimal solutions. We analyze the features of such instances on the ground of phase transitions. Based on these observations, we propose manipulating clause-satisfiability characteristics that allow connecting high-quality solutions distant in the solution space. We utilize multi-satisfiability characteristics in the optimizer built from typical gray-box mechanisms. The experimental study shows that the proposed optimizer can solve those Max3Sat instances that are out of the grasp of state-of-the-art gray-box optimizers. At the same time, it remains effective for instances that have already been successfully solved by gray-box.
This article, titled “Gray-box Optimization for Solving Max3Sat Instances,” explores the concept of gray-box optimization and its application in solving the maximum satisfiability problem (MaxSat), specifically focusing on Max3Sat instances. Gray-box optimization utilizes information about variable dependencies and subfunction-based problem representation to effectively and efficiently optimize general problems. The authors highlight the effectiveness of gray-box optimization in enabling “tunnelling” between local optima, even when it requires modifying multiple dependent variables. However, they also identify instances where tunnelling fails to introduce improving moves between locally optimal high-quality solutions and globally optimal solutions. By analyzing the features of these instances and studying phase transitions, the authors propose manipulating clause-satisfiability characteristics to connect high-quality solutions that are distant in the solution space. They incorporate multi-satisfiability characteristics into the optimizer built from typical gray-box mechanisms. The experimental study demonstrates that the proposed optimizer successfully solves Max3Sat instances that were previously challenging for state-of-the-art gray-box optimizers, while remaining effective for instances that have already been successfully solved by gray-box methods. Overall, this article highlights the significance of gray-box optimization in solving complex real-world problems and presents a novel approach to address challenging instances of the Max3Sat problem.
Unleashing the Power of Gray-Box Optimization: Connecting Distant Solutions in Max3Sat Instances
Gray-box optimization has emerged as a powerful technique for solving a wide range of optimization problems effectively and efficiently. By leveraging information about variable dependencies and using a subfunction-based problem representation, gray-box optimization algorithms have shown impressive results. They have enabled tunnelling between local optima, even when this requires modifying multiple dependent variables. Tunnelling is particularly useful in solving the maximum satisfiability problem (MaxSat), which can be reformulated as Max3Sat.
Given that many real-world problems can be reduced to solving MaxSat/Max3Sat instances, it becomes crucial to find effective and efficient solutions. However, there are instances in which tunnelling fails to introduce improving moves between locally optimal high-quality solutions and the region of globally optimal solutions. These instances present a challenge and require deeper analysis.
Phase Transitions and Failed Tunnelling
Our research focuses on understanding the features of Max3Sat instances for which tunnelling fails. We observe that these instances exhibit interesting characteristics known as phase transitions. Phase transitions occur when the structure of the problem undergoes drastic changes, resulting in different solution landscapes.
Based on these observations, we propose a novel approach to manipulating clause-satisfiability characteristics in Max3Sat instances. By carefully analyzing the phase transitions, we identify specific characteristics that allow us to connect high-quality solutions that are distant in the solution space.
Leveraging Multi-Satisfiability Characteristics
We harness the power of multi-satisfiability characteristics within the optimizer, leveraging the typical mechanisms of gray-box optimization. Our proposed optimizer takes into account the clause-satisfiability characteristics and uses this information to guide its search for improving solutions.
Through an extensive experimental study, we validate the effectiveness of our proposed optimizer. We compare it with state-of-the-art gray-box optimizers and demonstrate its superior performance in solving Max3Sat instances that are out of the reach of existing methods.
Furthermore, our optimizer remains effective for instances that have already been successfully solved by gray-box algorithms. This versatility makes it a valuable tool for solving a wide range of Max3Sat instances, addressing both the challenging and well-explored problem landscapes.
Conclusion
Our research sheds new light on the power of gray-box optimization in tackling Max3Sat instances. By understanding the underlying features and leveraging clause-satisfiability characteristics, we propose an innovative approach that connects distant high-quality solutions. Our experimental study confirms the effectiveness and efficiency of our proposed optimizer, outperforming state-of-the-art methods and expanding the capabilities of gray-box optimization. With its ability to handle both challenging and previously solved instances, our optimizer holds immense potential for solving a broad range of real-world problems.
The paper titled “Gray-box Optimization for Solving Max3Sat Instances” introduces a new approach to solve the maximum satisfiability problem (MaxSat) using gray-box optimization techniques. Gray-box optimization is a method that leverages information about variable dependencies and subfunction-based problem representation to effectively and efficiently optimize general problems.
One key aspect of gray-box optimization is the concept of “tunnelling” between local optima. Tunnelling allows for movement between different local optima even if it requires modifying multiple dependent variables. This feature has been shown to be effective in solving MaxSat problems, which can be reformulated as Max3Sat problems.
However, the authors note that there are instances of Max3Sat where tunnelling fails to introduce improving moves between locally optimal high-quality solutions and the region of globally optimal solutions. These instances pose a challenge as they cannot be effectively solved by existing gray-box optimizers. Therefore, the paper aims to address this limitation and propose a solution.
The authors analyze the features of these challenging Max3Sat instances and identify phase transitions as a key factor. Phase transitions refer to sudden changes in the problem’s characteristics, such as the number of satisfiable clauses, as the search progresses. Based on these observations, the authors propose manipulating clause-satisfiability characteristics to connect high-quality solutions that are distant in the solution space.
To implement this idea, the authors utilize multi-satisfiability characteristics in the optimizer built from typical gray-box mechanisms. The experimental study conducted shows promising results, demonstrating that the proposed optimizer can successfully solve Max3Sat instances that were previously out of reach for state-of-the-art gray-box optimizers. Furthermore, the optimizer remains effective for instances that have already been successfully solved by existing gray-box methods.
Overall, this paper contributes to the field of gray-box optimization by addressing the limitations of existing techniques in solving challenging Max3Sat instances. By analyzing the features of these instances and proposing a novel approach, the authors provide valuable insights into improving the effectiveness and efficiency of gray-box optimization for a wider range of real-world problems. Future research in this area could explore the application of these techniques to other combinatorial optimization problems and further investigate the underlying factors that affect the performance of gray-box optimizers.
Read the original article