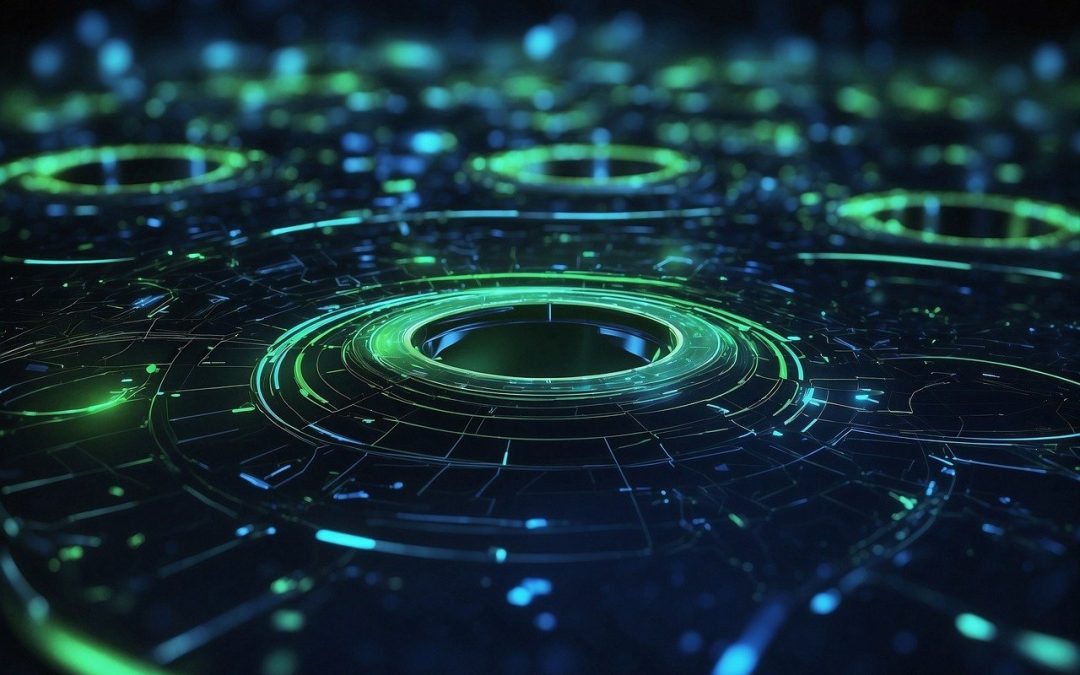
“HyperCausalLP: Enhancing Causal Network Completion with Mediator Links”
Abstract:
Causal networks are often incomplete with missing causal links. This is due to various issues, such as missing observation data. Recent approaches to the issue of incomplete causal networks have used knowledge graph link prediction methods to find the missing links.
In the causal link A causes B causes C, the influence of A to C is influenced by B which is known as a mediator. Existing approaches using knowledge graph link prediction do not consider these mediated causal links.
This paper presents HyperCausalLP, an approach designed to find missing causal links within a causal network with the help of mediator links. The problem of missing links is formulated as a hyper-relational knowledge graph completion. The approach uses a knowledge graph link prediction model trained on a hyper-relational knowledge graph with the mediators.
The approach is evaluated on a causal benchmark dataset, CLEVRER-Humans. Results show that the inclusion of knowledge about mediators in causal link prediction using hyper-relational knowledge graph improves the performance on an average by 5.94% mean reciprocal rank.
Expert Commentary:
Causal networks are essential for understanding complex systems and their dynamics. However, incomplete causal networks pose a challenge as they limit our ability to fully comprehend the underlying causal relationships. This limitation can arise from various factors, such as missing observation data.
Recent approaches have focused on utilizing knowledge graph link prediction methods to address the problem of missing causal links. These methods aim to leverage the existing information in the causal network to predict the missing links accurately.
One aspect that has been often overlooked in previous approaches is the role of mediators in the causal network. In a causal chain where A causes B causes C, the influence of A on C is mediated by B. Understanding these mediator links is crucial for developing a more comprehensive understanding of causal relationships.
The HyperCausalLP approach presented in this paper takes into account the mediator links to find missing causal links within a causal network. By formulating the problem as a hyper-relational knowledge graph completion, the approach combines the knowledge of mediators with the existing causal network information.
To enable the prediction of missing links, the approach utilizes a knowledge graph link prediction model trained on a hyper-relational knowledge graph that includes mediators. This training enhances the ability of the model to capture and leverage the mediator links effectively.
The evaluation of HyperCausalLP on the CLEVRER-Humans benchmark dataset demonstrates promising results. The inclusion of mediator knowledge in the causal link prediction improves the performance, as indicated by the average 5.94% mean reciprocal rank improvement.
Overall, this approach fills an important gap in existing methods for incomplete causal networks by considering the mediated causal links. By incorporating knowledge about mediators in the prediction process, the HyperCausalLP approach provides a more accurate and comprehensive understanding of causal relationships within a network.
Future research in this area could explore the application of HyperCausalLP to larger and more complex causal networks, as well as investigate the impact of different types of mediators on the performance of the approach. Additionally, considering the uncertainty and confidence levels associated with predicted causal links could be a valuable direction for further enhancements in the field.