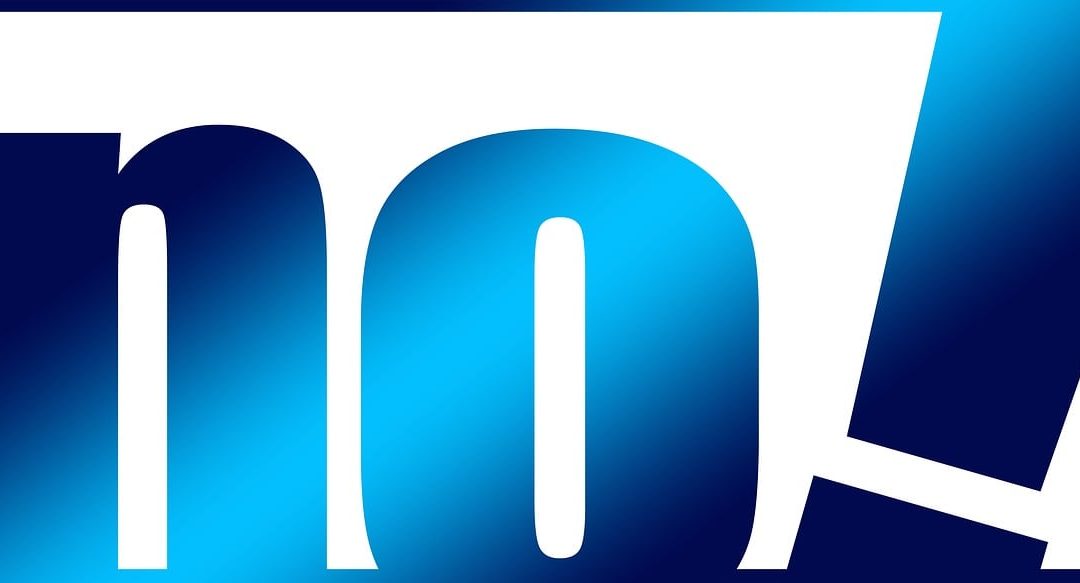
Enhancing Uplink Transmission with MU-LCFSC Framework
Expert Commentary: Improving Uplink Transmission with MU-LCFSC Framework
In this paper, the authors address the issue of uplink transmission over a multiple-input multiple-output multiple access channel (MIMO-MAC). They propose a multi-user learnable CSI fusion semantic communication (MU-LCFSC) framework that incorporates channel state information (CSI) into the semantic encoders and decoders to enhance the attention weight distribution and improve the overall performance.
The main contribution of this paper lies in the integration of CSI as side information into the semantic encoders and decoders. By doing so, the authors are able to generate a feature mask map that allows for a more robust attention weight distribution. This is crucial in scenarios where multiple users share the same channel and interference is a major challenge.
One interesting aspect of the proposed framework is the cooperative successive interference cancellation procedure conducted at the decoding end. This procedure, along with the cooperative mask ratio generator, enables flexible control of the mask elements of the feature mask maps. This flexibility is essential in adapting to different channel conditions and optimizing the overall system performance.
To validate the effectiveness of the proposed MU-LCFSC framework, the authors present numerical results comparing it to DeepJSCC-NOMA. The results demonstrate the superiority of MU-LCFSC, as it achieves a higher peak signal-to-noise ratio (PSNR) by 3 dB. This improvement in performance is substantial and highlights the potential of the proposed framework in enhancing uplink transmission efficiency.
In conclusion, the authors successfully address the challenges of uplink transmission in MIMO-MAC scenarios through the proposed MU-LCFSC framework. By incorporating CSI into the semantic encoders and decoders, they are able to generate a more robust attention weight distribution, leading to improved performance. The cooperative successive interference cancellation procedure and cooperative mask ratio generator further enhance the flexibility of the framework. The numerical results validate the superiority of the proposed framework over DeepJSCC-NOMA in terms of PSNR. Moving forward, it would be interesting to see the application of the MU-LCFSC framework in practical scenarios and its impact on real-world wireless communication systems.