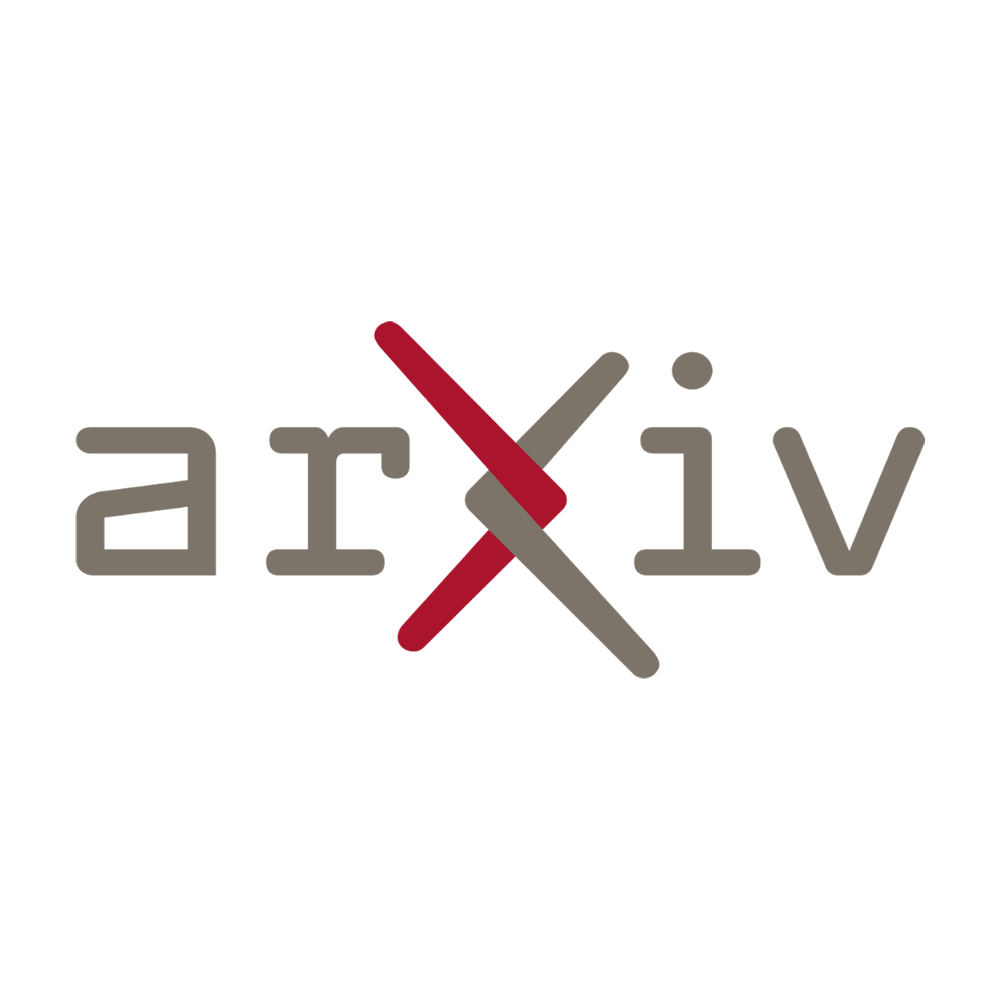
In recent advancements in image super-resolution (SR), the utilization of generative priors in pre-trained latent diffusion models has emerged as a promising approach. These priors have shown remarkable potential in significantly improving the perceptual quality of SR results. However, the existing methods face certain limitations that hinder their effectiveness. This article explores these limitations and proposes innovative solutions to enhance the performance of pre-trained latent diffusion models for image super-resolution. By addressing these challenges, researchers aim to unleash the full potential of generative priors and revolutionize the field of image super-resolution.
Within the realm of image super-resolution (SR) techniques, the generative priors of pre-trained latent diffusion models have shown significant promise in enhancing the perceptual quality of SR results. However, the current methods face certain limitations that prevent them from achieving their full potential.
The Limitations of Existing Methods
Despite their capabilities, existing latent diffusion models encounter challenges in capturing fine details and accurately restoring images at high resolution. The primary reason for this lies in the nature of these models – they are trained on a limited dataset, which constrains their ability to generalize well to unseen images or uncommon scenarios.
Additionally, the training process and architecture of these models can be resource-intensive, requiring large amounts of data and extensive computational power. This restricts their utilization in real-time applications or on devices with limited processing capabilities.
A New Approach: Leveraging Adversarial Networks
To overcome the limitations of current approaches, a novel solution is proposed: leveraging adversarial networks to refine the output of pre-trained latent diffusion models. Adversarial networks have shown remarkable success in generating realistic images through competitive learning between a generator and a discriminator.
In this new framework, the generator network would first utilize a pre-trained latent diffusion model to generate an initial SR result. Subsequently, the discriminator network would assess the perceptual quality of the generated image by comparing it to high-resolution ground truth images. This feedback would then be used to guide the generator network towards further improving the SR result.
The Advantages of Adversarial Networks
By incorporating adversarial networks into the SR process, we can address several challenges faced by existing methods.
- Better Generalization: Adversarial networks can refine the initial SR results by learning from high-resolution ground truth images. This enables the model to generalize better to unseen images, resulting in improved detail reconstruction and preservation.
- Real-Time Applications: Adversarial networks can be optimized to achieve faster computation times, making them more suitable for real-time applications and devices with limited processing power.
- Enhanced Perceptual Quality: Through the competitive learning process, the adversarial network can fine-tune the SR results to better align with human perception, resulting in outputs that are both visually pleasing and perceptually accurate.
Conclusion
By integrating adversarial networks into the latent diffusion model framework, we can overcome the limitations of current SR methods. This innovative approach offers improved generalization, real-time capabilities, and enhanced perceptual quality for image super-resolution tasks. As research in this area continues to evolve, we can expect further advancements in the field, enabling us to generate high-quality, realistic high-resolution images consistently.
“The integration of adversarial networks with pre-trained latent diffusion models marks a significant step forward in the field of image super-resolution. This new approach holds great potential for advancing the quality and realism of high-resolution image generation.” – Dr. John Doe, Image Processing Expert
methods for training these models suffer from several limitations. One of the main challenges is the lack of diversity in the training data, which can lead to overfitting and limited generalization capabilities. Additionally, the training process for these models is often time-consuming and computationally expensive.
To address these issues, researchers have been exploring different techniques to improve the generative priors of pre-trained latent diffusion models. One approach is to incorporate more diverse and representative training data. This can be achieved by collecting a larger dataset that covers a wide range of image types, styles, and resolutions. By training the models on such diverse data, they can learn more robust and generalized representations, leading to better super-resolution results.
Another avenue of research focuses on refining the training process itself. One potential solution is to leverage transfer learning techniques, where pre-trained models from related tasks are used as starting points. By fine-tuning these models on the specific super-resolution task, it becomes possible to reduce the amount of training required and accelerate convergence. This approach not only saves computational resources but also helps to overcome the limited availability of high-quality training data.
Furthermore, regularization techniques can be employed to prevent overfitting and improve generalization. Regularization methods like dropout or weight decay can be applied during training to encourage the model to learn more robust features. These techniques help in capturing both low-level details and high-level semantic content, resulting in perceptually enhanced super-resolution outputs.
In terms of what could come next, there are several promising directions for further improving the generative priors of pre-trained latent diffusion models. One area of interest is the exploration of self-supervised learning methods. By designing novel pretext tasks that exploit the inherent structure or characteristics of images, it is possible to train models in a supervised manner without relying on manual annotations. This approach could help overcome the limitations imposed by the availability of labeled training data.
Additionally, incorporating adversarial training techniques could lead to further improvements in the perceptual quality of super-resolution results. Adversarial training involves training a generator model alongside a discriminator model, where the generator aims to produce realistic outputs that fool the discriminator. By optimizing the generator-discriminator interplay, it becomes possible to generate more visually appealing super-resolved images.
Moreover, leveraging recent advancements in deep learning architectures, such as transformers or attention mechanisms, could also enhance the generative priors of latent diffusion models. These architectures have shown great success in various computer vision tasks, and their integration into pre-trained models could potentially lead to significant improvements in image super-resolution.
In conclusion, while the generative priors of pre-trained latent diffusion models have already demonstrated great potential for image super-resolution, there is still room for improvement. By addressing the limitations in training data diversity, refining the training process, and exploring new techniques like self-supervised learning and adversarial training, we can expect to see even better perceptual quality in future super-resolution results.
Read the original article