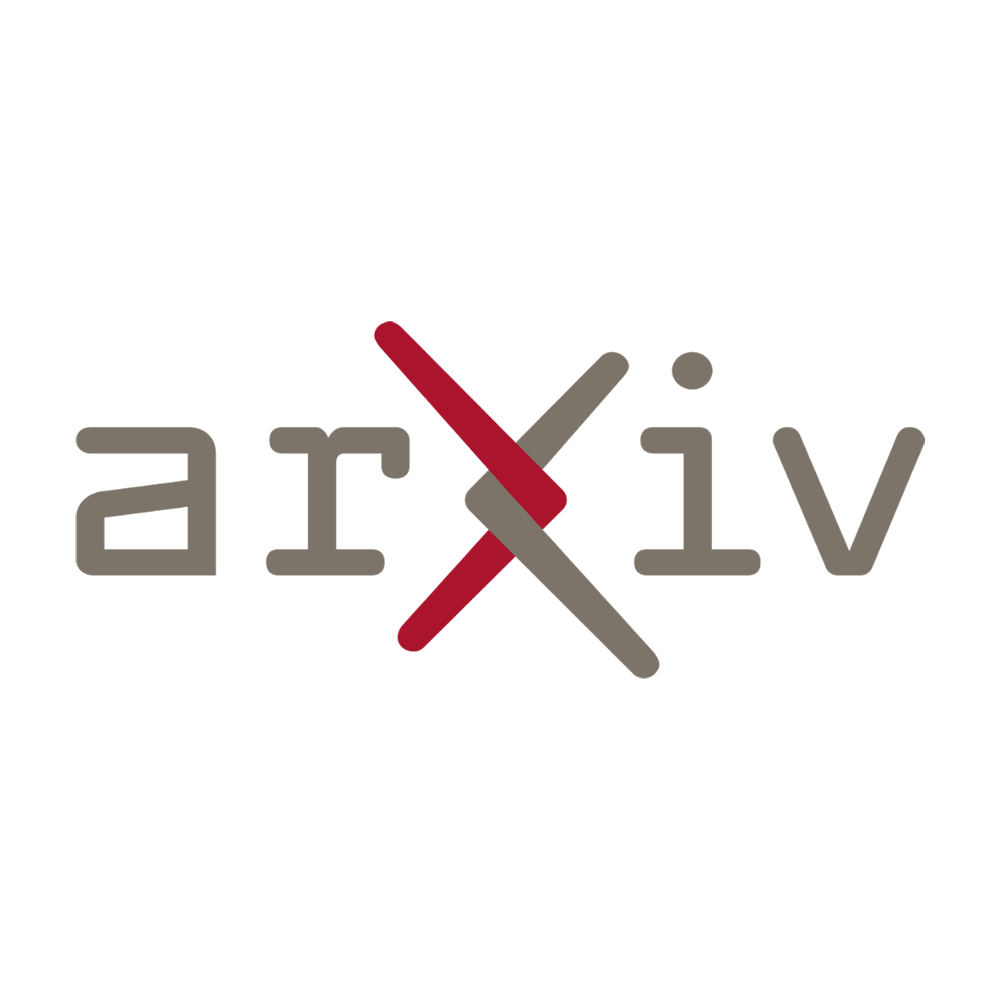
In the realm of text-to-video (T2V) generation, a major hurdle lies in achieving controllability in the resulting videos. This challenge has been commonly addressed through the implementation of low-level techniques. However, a new approach is emerging that focuses on high-level control, allowing for more precise manipulation and customization of synthesized videos. In this article, we explore the advancements in controllability within T2V generation and delve into the potential implications and benefits of this novel approach.
Within recent approaches to text-to-video (T2V) generation, achieving controllability in the synthesized video is often a challenge. Typically, this issue is addressed by providing low-level control signals, such as pose or motion sequences, to guide the video generation process. While these methods have shown promising results, they still struggle to capture high-level semantic information from the input text and incorporate it into the generated video.
One innovative solution to this problem is to leverage the power of natural language processing (NLP) techniques to extract meaningful semantic information from the input text. By breaking down the text into its constituent parts and analyzing their relationships, we can identify key themes and concepts that should be reflected in the generated video. This approach not only enhances the controllability of the synthesized video but also allows for more nuanced and contextually rich outputs.
The Importance of Semantic Understanding
In traditional T2V approaches, the focus has primarily been on matching textual descriptions with corresponding visual elements. While this can result in videos that closely resemble the given text, they lack a deeper understanding of the underlying semantics. For example, consider a text that describes a person walking through a park on a sunny day. A traditional T2V system might synthesize a video that simply shows a person walking in a park, without considering the nuances of weather or the mood associated with a sunny day.
By incorporating semantic understanding into the T2V generation process, we can go beyond direct textual matching and produce videos that capture the essence of the input text. This can be achieved by employing powerful NLP tools such as dependency parsing and semantic role labeling to analyze the relationships between words and extract key concepts. For instance, by identifying the words “sunny day” and “park,” we can infer that the video should depict a bright, cheerful atmosphere.
Controllable Video Generation Through Semantic Embeddings
Once we have extracted the relevant semantic information from the input text, the next step is to incorporate it into the video generation process. This can be done by using semantic embeddings, which encode the extracted concepts in a format that is easily understandable by the video synthesis model. By mapping these embeddings to appropriate visual elements and scenes, we can ensure that the generated video reflects the intended semantics.
To achieve this, we can develop a mapping between the semantic embeddings and specific control signals that guide the video generation process. For example, the embedding for a “sunny day” concept could be linked to a control signal that adjusts the lighting and color scheme of the synthesized video to create a bright and vibrant scene.
Enhancing Realism with Contextual Knowledge
In addition to semantic understanding, contextual knowledge plays a vital role in producing realistic and coherent videos. By analyzing the input text within its broader context, we can take into account relevant information that might not be explicitly mentioned but is crucial for generating a believable video.
For instance, consider a phrase like “a person entering a café.” While this might seem like a straightforward instruction, additional contextual knowledge is required to determine what kind of café it is. By analyzing the surrounding words or utilizing external resources such as knowledge graphs or databases, we can retrieve information about various types of cafes (e.g., coffee shops, fine dining establishments) and guide the video generation accordingly.
Conclusion
By incorporating semantic understanding and contextual knowledge into the T2V generation process, we can enhance controllability and produce videos that go beyond literal interpretations of input texts. Leveraging NLP techniques allows us to capture high-level semantic information and incorporate it into the synthesis model, resulting in more contextually rich and nuanced videos. This approach opens up exciting possibilities for applications in entertainment, education, and beyond, where video generation that captures the essence of textual input is essential.
control signals, such as specifying the style, attributes, or actions of the generated video. However, these low-level control signals may not capture the high-level intentions or semantics of the input text, resulting in videos that lack coherence or fail to convey the desired message.
To overcome this challenge, researchers have started exploring the use of higher-level control mechanisms in T2V generation. By incorporating natural language understanding and reasoning capabilities into the system, it becomes possible to generate videos that align more closely with the intended meaning of the input text.
One promising direction in achieving controllability is through the use of semantic parsing techniques. Semantic parsing involves mapping natural language sentences to structured representations that capture their underlying meaning. By leveraging this approach, T2V systems can better understand the input text and generate videos that align with its semantics.
Another important aspect of achieving controllability is incorporating user preferences and constraints into the video synthesis process. For example, allowing users to specify desired camera angles, scene compositions, or character behaviors can greatly enhance the controllability of the generated videos. This requires developing interactive interfaces that allow users to provide input and receive immediate feedback on how their instructions are being translated into the synthesized video.
Furthermore, advances in machine learning, particularly in areas such as reinforcement learning and generative adversarial networks (GANs), hold great promise for improving controllability in T2V generation. Reinforcement learning can be used to train models that learn to generate videos based on reward signals, allowing for fine-grained control over various aspects of the synthesized video. GANs, on the other hand, can enable the generation of realistic and diverse videos by learning from large amounts of data.
Looking ahead, the future of controllable T2V generation lies in combining these different approaches and leveraging multimodal techniques that integrate text, image, and video modalities. By jointly modeling these modalities and their interactions, it becomes possible to generate videos that not only align with the input text but also incorporate visual cues and context from the accompanying images or videos.
In conclusion, achieving controllability in T2V generation is a complex challenge that requires advancements in natural language understanding, semantic parsing, user interaction, and machine learning. By incorporating higher-level control mechanisms and multimodal techniques, we can expect to see significant improvements in the quality and coherence of synthesized videos, leading to more effective communication and storytelling through text-to-video systems.
Read the original article