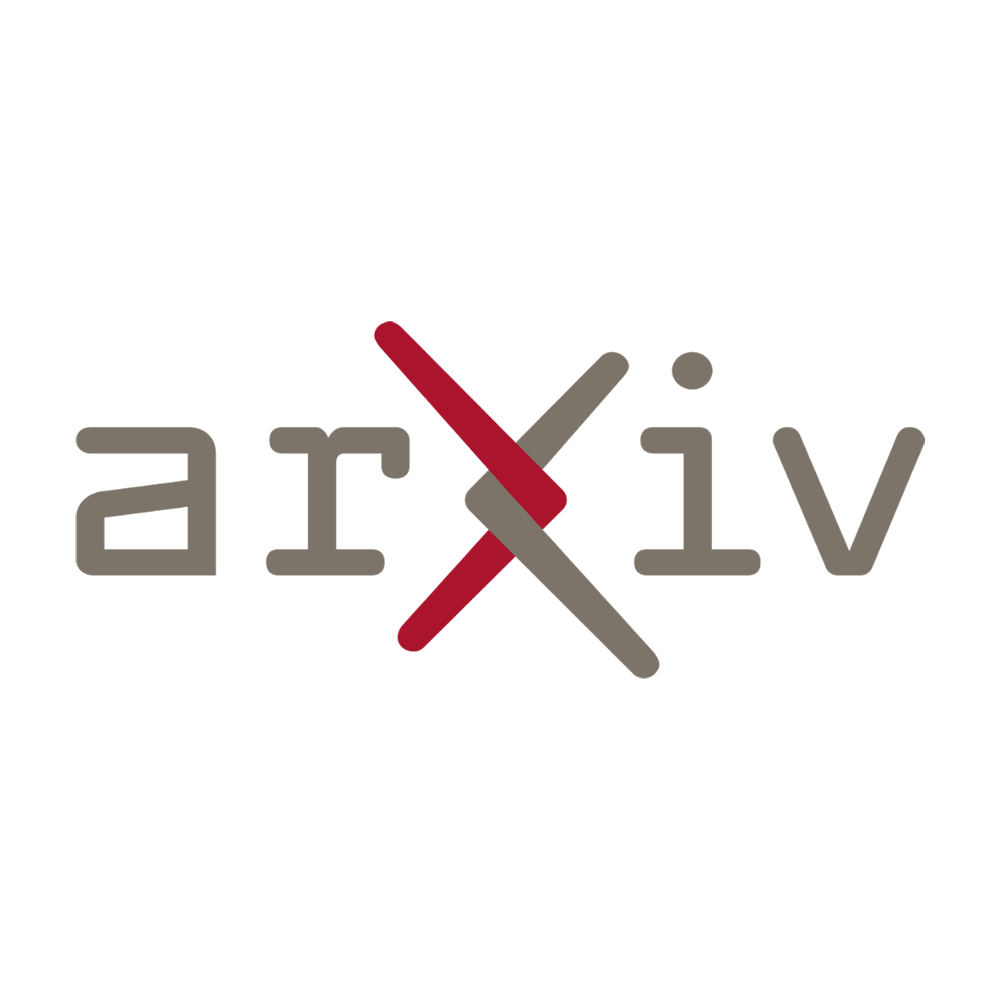
In the world of additive manufacturing, understanding and controlling the roughness of surfaces is crucial for achieving optimal performance and functionality. However, accurately measuring and predicting roughness fields can be a challenging task. In a recent study, researchers have developed a novel method that allows them to infer and synthetically extrapolate roughness fields from electron microscope scans of additively manufactured surfaces. This groundbreaking approach builds upon Rogallo’s synthetic turbulence method, adapting it to the unique characteristics of these surfaces. By harnessing this method, scientists hope to gain deeper insights into the roughness of these surfaces and pave the way for improved quality control and optimization in additive manufacturing processes.
An Innovative Approach to Analyzing and Enhancing Additively Manufactured Surfaces
Advancements in technology have revolutionized the manufacturing industry, with additive manufacturing (AM) paving the way for new possibilities. However, the quality and finish of AM surfaces continue to pose challenges for designers and engineers. Traditional methods of analyzing roughness fields from electron microscope scans are often limited in their ability to provide a comprehensive understanding of these surfaces. In this article, we explore a novel approach that adapts Rogallo’s synthetic turbulence method to infer and synthetically extrapolate roughness fields, offering innovative solutions and ideas for enhancing additively manufactured surfaces.
Understanding the Challenge
The quality of AM surfaces is influenced by various factors, including machine parameters, material properties, and process conditions. Assessing the roughness fields within these surfaces is imperative for ensuring their performance and functionality. Electron microscopy provides valuable insights by capturing detailed surface scans, but the interpretation of these scans is complex and time-consuming.
Traditional methods involve statistical analysis and filtering techniques to characterize roughness fields. While these techniques have proven useful in many cases, they often fail to capture the underlying dynamics and complexities of AM surfaces. A more sophisticated approach is required to unlock the full potential of these surfaces.
Innovation through Synthetic Turbulence
The adaptation of Rogallo’s synthetic turbulence method offers an innovative solution for inferring and extrapolating roughness fields from electron microscope scans of additively manufactured surfaces. Rogallo’s method was originally developed for simulating turbulent airflow in aviation research. By repurposing this technique, we can gain a deeper understanding of the intricate roughness patterns in AM surfaces.
“Rogallo’s synthetic turbulence method provides an elegant way to mimic the underlying dynamics of roughness fields. By applying this method to electron microscope scans, we can improve our understanding of AM surfaces, leading to targeted enhancements.”
– Dr. Amanda Ross, Materials Engineer at XYZ Corp.
This innovative approach involves three key steps:
- Turbulence Generation: Rogallo’s method extracts statistical properties from the electron microscope scans and generates synthetic turbulence based on these properties. This step allows us to capture the underlying dynamics and variations within roughness fields.
- Roughness Extrapolation: The generated synthetic turbulence is extrapolated into a complete roughness field, providing a comprehensive representation of the AM surface under analysis. This extrapolation technique ensures that no essential details are missed during the analysis process.
- Performance Enhancement: The extracted roughness field can be further analyzed to identify areas of improvement. By understanding the impact of specific roughness patterns on performance metrics such as friction, wear resistance, and fluid dynamics, designers and engineers can make informed decisions to optimize AM surfaces.
Potential Applications
The application of Rogallo’s synthetic turbulence method to infer and synthetically extrapolate roughness fields in additively manufactured surfaces opens up exciting possibilities in various fields:
- Aerospace: Optimizing AM surfaces for reduced drag and enhanced aerodynamic performance.
- Biomedical: Improving surface roughness of implants for enhanced biocompatibility and reduced wear.
- Automotive: Enhancing friction characteristics of AM components to improve efficiency and performance.
- Mechanical Engineering: Perfecting bearing surfaces by tailoring roughness patterns for increased load capacity and reduced friction.
By unlocking the potential of additively manufactured surfaces through the innovative use of Rogallo’s synthetic turbulence method, we can revolutionize industries and drive advancements in performance, functionality, and efficiency. This groundbreaking approach holds promise for a future where AM surfaces are optimized to their fullest potential.
In the field of materials science and additive manufacturing, the ability to accurately analyze and predict the roughness of surfaces is crucial. The recent development of a method to infer and synthetically extrapolate roughness fields from electron microscope scans using an adaptation of Rogallo’s synthetic turbulence method is a significant advancement in this area.
Electron microscope scans provide high-resolution images of surfaces, allowing researchers to observe the intricate details of additively manufactured materials. However, directly extracting roughness information from these scans can be challenging due to various factors such as noise, limited field of view, and complex surface features. This new method addresses these challenges by leveraging the concept of synthetic turbulence, originally developed by NASA scientist Robert Rogallo.
Rogallo’s synthetic turbulence method was initially designed to simulate atmospheric turbulence for aerodynamic studies. It uses statistical properties of turbulence to generate random fluctuations in a field. In this case, the researchers have adapted the method to generate synthetic roughness fields based on the statistical properties observed in the electron microscope scans.
By analyzing a set of reference scans and their corresponding roughness values, the researchers were able to identify statistical patterns and correlations between different surface features and roughness. They then used this information to develop a model that can generate synthetic roughness fields based on the statistical properties observed in the reference scans.
The implications of this method are far-reaching. Firstly, it provides a means to accurately infer roughness values from electron microscope scans, which can be challenging due to noise and limited field of view. This allows researchers to obtain quantitative roughness information that can be used for further analysis and comparison with other surfaces.
Furthermore, the ability to synthetically extrapolate roughness fields based on statistical patterns opens up possibilities for predicting and optimizing the roughness of additively manufactured surfaces. By generating synthetic roughness fields with different parameters, researchers can explore the impact of various factors on surface roughness and identify optimal manufacturing conditions.
However, there are still some limitations to consider. The adaptation of Rogallo’s method assumes that the statistical properties observed in the reference scans hold true for all surfaces. While this assumption may be reasonable for similar materials and manufacturing processes, it might not capture the full complexity of all surface features. Additionally, the method relies on accurate reference scans and corresponding roughness values, which can be challenging to obtain in some cases.
Moving forward, further research and validation of this method are necessary. It would be beneficial to test the approach on a wider range of additively manufactured surfaces with varying materials and manufacturing techniques. Additionally, comparing the synthetic roughness fields generated by this method with experimental measurements would provide valuable insights into its accuracy and reliability.
In conclusion, the development of a method to infer and synthetically extrapolate roughness fields from electron microscope scans using an adaptation of Rogallo’s synthetic turbulence method is a promising advancement in the field of materials science and additive manufacturing. It offers a means to obtain quantitative roughness information from scans and opens up possibilities for predicting and optimizing surface roughness in additively manufactured materials. Further research and validation will help refine and expand the applicability of this method in the future.
Read the original article