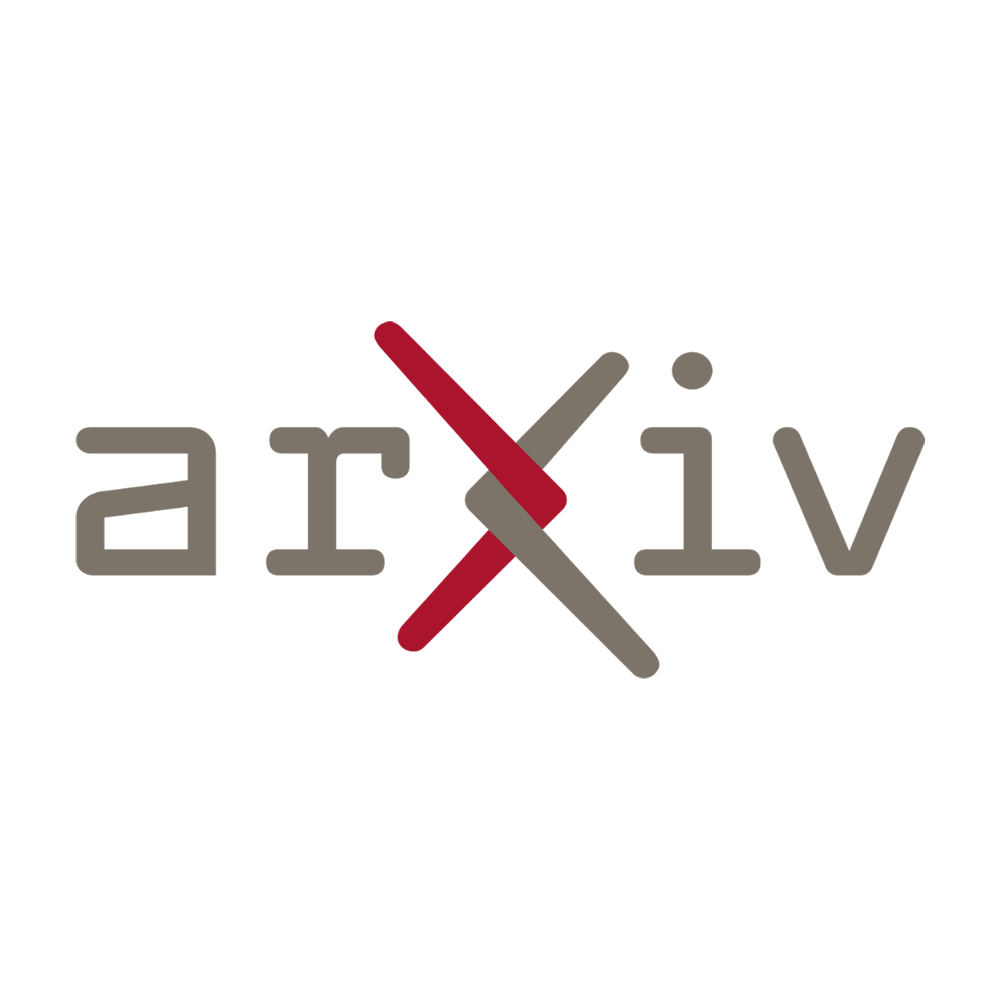
This article delves into the concept of video grounding, which involves identifying a specific section in a video that matches a given text query. It highlights a significant drawback in existing video grounding techniques and proposes a solution to overcome this limitation. By focusing on localizing spatio-temporal sections in videos based on textual input, this paper introduces a novel approach to enhance video grounding methodologies.
Title: Rethinking Video Grounding: Overcoming Limitations and Unlocking New Possibilities
Introduction:
Video grounding aims to localize a spatio-temporal section in a video corresponding to an input text query. This paper addresses a critical limitation in current video grounding methodologies by…
Video grounding, the process of associating a specific text query with a corresponding section in a video, has gained significant attention as a means to enhance video understanding and retrieval systems. However, current methodologies in this field pose certain limitations that hinder their effectiveness. In this article, we will explore these limitations and propose innovative solutions and ideas to overcome them, unlocking new possibilities for video grounding.
Understanding the Limitations:
Current video grounding methodologies face several critical limitations that impede their performance. These limitations include:
- Lack of temporal context: Existing approaches primarily focus on matching textual queries to individual frames or short snippets of videos. However, neglecting the temporal context can lead to inadequate grounding results, as crucial information may be missed.
- Dependency on pre-defined visual features: Many video grounding approaches rely on pre-defined visual features extracted from frames or objects in videos. This reliance limits the flexibility and adaptability of the system, as it fails to capture the diverse nature of visual content.
- Scalability concerns: The current state-of-the-art video grounding methods often require extensive computational resources and time-consuming procedures, making them less efficient for real-time applications or large-scale video datasets.
Proposing Innovative Solutions:
To overcome these limitations and push the boundaries of video grounding, we propose the following innovative solutions:
- Integrating Temporal Context: Rather than treating videos as a series of isolated frames, incorporating temporal context by analyzing the connection between adjacent frames enhances the understanding of the overall video content. This can involve employing motion-based features or considering long-term dependencies.
- Learning Dynamic Visual Representations: Instead of relying solely on pre-defined visual features, leveraging deep learning approaches can enable the system to learn dynamic visual representations from raw video data. By doing so, the grounding system becomes more adaptable to different types of videos and captures fine-grained details.
- Efficient Parallel Processing: Utilizing parallel processing architectures, such as GPUs or distributed computing frameworks, can significantly enhance the efficiency and scalability of video grounding methods. Parallelization allows for simultaneous processing of multiple videos or sections, enabling real-time applications and handling large-scale datasets.
Unlocking New Possibilities:
By addressing the limitations of current video grounding methodologies and adopting these innovative solutions, we can unlock new possibilities for video understanding and retrieval systems:
- Enhanced Video Search: Improved video grounding techniques would empower users to search and retrieve specific sections within videos, revolutionizing how we navigate and explore video content.
- Interactive Multimedia Applications: With more accurate and efficient video grounding mechanisms, interactive multimedia applications can be developed, allowing users to conveniently extract relevant information from videos or create personalized video summaries.
- Augmented Reality (AR) Integration: Video grounding advancements offer the potential for seamless integration with AR technologies. By accurately localizing spatio-temporal sections, AR experiences can be enriched with contextual information overlaid onto real-world videos.
Conclusion:
Redefining video grounding through the integration of temporal context, dynamic visual representations, and efficient parallel processing opens up a realm of possibilities for video understanding and retrieval systems. Overcoming limitations and embracing innovation will enable us to unleash the true potential of video grounding and greatly enhance our interaction with visual content.
introducing a novel approach to improve the accuracy and efficiency of video grounding through the integration of multimodal information and advanced deep learning techniques.
Video grounding, also known as video localization or video object grounding, refers to the task of identifying and localizing specific objects or events in a video based on a given textual description. This technology has numerous applications, including video summarization, content-based video retrieval, and video understanding.
The paper highlights a critical limitation in existing video grounding methodologies, which often struggle with accurately localizing spatio-temporal sections in videos corresponding to input text queries. This limitation arises due to the inherent complexity of video data, which contains both visual and temporal information that needs to be effectively processed and understood.
To address this challenge, the authors propose a new approach that leverages multimodal information fusion and deep learning techniques. By combining textual and visual features, the model aims to establish a stronger connection between the input text query and the corresponding video segment.
One key aspect of this approach is the use of advanced deep learning architectures, such as convolutional neural networks (CNNs) and recurrent neural networks (RNNs), to extract high-level representations from both the textual and visual modalities. These representations are then fused together using fusion mechanisms like attention mechanisms or multimodal embeddings.
The integration of multimodal information allows the model to capture both semantic and visual cues, enabling more accurate and robust video grounding. For example, by jointly considering the textual description and visual content, the model can better understand and interpret complex queries involving spatial relationships, object interactions, or temporal dependencies.
Moreover, the authors address the efficiency aspect of video grounding by proposing optimization techniques to speed up the inference process. This is crucial for real-time applications or scenarios where large-scale video datasets need to be processed.
Looking ahead, this paper opens up several possibilities for future research in video grounding. Firstly, the proposed approach could be further enhanced by incorporating other modalities, such as audio or motion information, to provide a more comprehensive understanding of video content. Additionally, investigating the potential of transfer learning or pre-training on large-scale video datasets could improve the generalization capabilities of the model.
Furthermore, exploring the application of this video grounding methodology in more complex scenarios, such as long videos or real-world surveillance footage, would be valuable. These scenarios often involve challenging conditions, occlusions, and multiple objects/events occurring simultaneously, which require more sophisticated algorithms for accurate localization.
In conclusion, this paper introduces a promising approach to address the limitations in current video grounding methodologies. By leveraging multimodal information and deep learning techniques, the proposed model shows potential for significantly improving the accuracy and efficiency of video grounding. Further research in this direction is expected to advance the field and unlock new possibilities for video understanding and analysis.
Read the original article