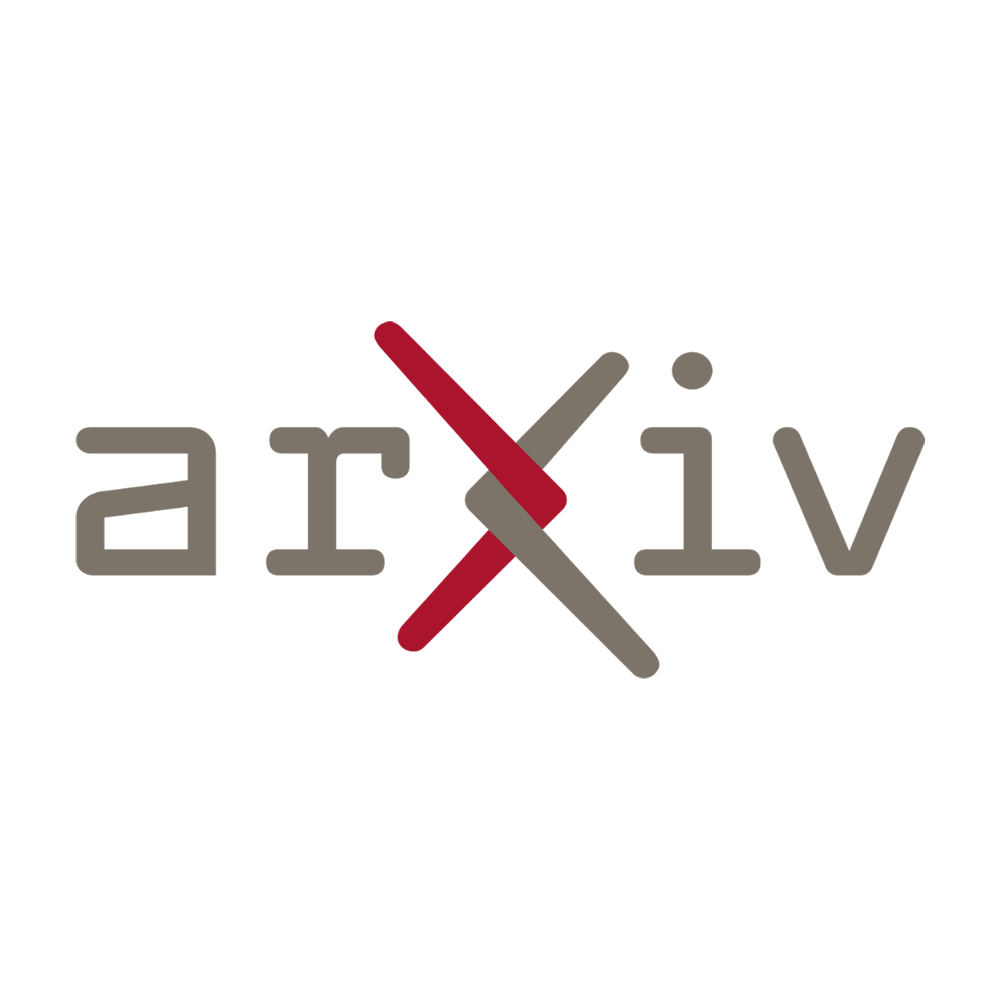
have revolutionized various fields such as natural language processing, computer vision, and speech recognition. Foundation models, also known as pre-trained models, have proven to be highly effective in solving complex problems by leveraging their ability to learn from extensive amounts of data. This article explores the significance of foundation models in deep learning and delves into their applications, benefits, and challenges. From their ability to understand and generate human-like text to their potential in democratizing AI, foundation models have emerged as a game-changer in the world of artificial intelligence.
In recent years, foundation models (FMs) have solidified their role as cornerstone advancements in the deep learning domain. By extracting intricate patterns from vast datasets, these models have revolutionized the way we approach complex problems and enhance machine learning capabilities. However, just like any technological breakthrough, FMs bring along their own set of challenges and limitations.
The Challenge of Interpretability
One of the key challenges posed by FMs is the lack of interpretability. While these models are excellent at identifying patterns and making predictions, understanding how they arrived at those conclusions is often a black box. This lack of transparency raises ethical concerns in critical domains like healthcare and finance, where decision-making based on opaque algorithms may result in biased outcomes or limited accountability.
An innovative solution to this problem lies in the concept of Explainable Artificial Intelligence (XAI). By integrating XAI techniques into FMs, we can unlock the underlying reasoning and decision-making processes. Techniques such as rule extraction, feature importance analysis, and attention mechanisms can shed light on the inner workings of these models, enabling users to trust and interpret their outputs.
Data Privacy and Security
Another pressing concern with FMs revolves around data privacy and security. These models require vast amounts of data to achieve optimal performance, which often includes sensitive information. As we increasingly rely on FMs to handle personal, business, and societal data, it becomes crucial to ensure robust privacy-preserving measures.
One innovative solution lies in federated learning, where the model is trained collaboratively across multiple decentralized devices or servers. By keeping the data at its source and only sharing updates rather than raw information, federated learning mitigates concerns regarding data exposure and centralized vulnerabilities. This approach not only protects privacy but also allows for a more distributed and resilient learning network.
Addressing Bias and Fairness
Bias and fairness have been persistent issues in machine learning, and FMs are not exempt from this challenge. Training these models on biased or unrepresentative data can lead to unjust outcomes and perpetuate existing inequalities. To ensure fairness and reduce bias in FM applications, it is crucial to adopt innovative solutions that address these concerns head-on.
One approach is to proactively curate diverse and representative datasets during the model development phase. Collaborating with domain experts and stakeholders can help identify potential biases and develop robust strategies to mitigate them. Additionally, ongoing monitoring and evaluation of FM performance can uncover bias patterns and prompt necessary adjustments to improve fairness.
Empowering Human Oversight
A final consideration in the advancement of FMs is the need for robust human oversight. While FMs have proven their ability to derive insights at an unprecedented scale, human expertise and intuition remain invaluable, particularly in high-stakes decision-making scenarios.
One innovative solution involves designing hybrid models that combine the strengths of FMs with a human-in-the-loop approach. These hybrid models enable human experts to interact with the system, provide feedback, and make informed decisions based on both model predictions and their own judgment. This symbiotic relationship between AI and human intelligence fosters a more accountable, explainable, and reliable decision-making process.
In conclusion, while foundation models have undoubtedly transformed the landscape of deep learning, they come with their own unique challenges. By embracing innovative solutions such as Explainable AI, federated learning, bias mitigation techniques, and human-in-the-loop approaches, we can address these challenges head-on and pave the way for a future where AI is transparent, privacy-conscious, fair, and human-centric.
have revolutionized various fields such as natural language processing, computer vision, and speech recognition. FMs, such as BERT, GPT-3, and Vision Transformers, have achieved remarkable success in tasks like language understanding, text generation, image classification, and object detection.
The power of FMs lies in their ability to learn intricate patterns and representations directly from the data, without the need for explicit feature engineering. They employ deep neural networks with millions or even billions of parameters, enabling them to capture complex relationships and dependencies within the input data. This has led to significant breakthroughs in tasks that were previously considered challenging or unsolved.
One of the key advantages of FMs is their capability to transfer knowledge across domains or tasks. Pretraining a FM on a large corpus of unlabeled data allows it to learn general features and linguistic structures. This pretrained model can then be fine-tuned on specific downstream tasks with smaller labeled datasets. This transfer learning approach has proven to be highly effective, reducing the need for large amounts of task-specific annotated data.
Looking ahead, we can expect further advancements in foundation models. One area of focus will be improving their efficiency and scalability. Current FMs are computationally expensive and require substantial computing resources. Researchers are actively exploring techniques such as model distillation, sparse attention mechanisms, and neural architecture search to make FMs more lightweight and accessible.
Another direction for improvement is enhancing the interpretability of FMs. Despite their remarkable performance, FMs often lack transparency in their decision-making process. Understanding how these models arrive at their predictions is crucial for building trust and ensuring fairness. Researchers are investigating techniques such as attention visualization, saliency maps, and explainable AI methods to shed light on the inner workings of FMs.
Furthermore, there is a growing interest in multimodal foundation models that can handle diverse types of data, such as text, images, speech, and videos. Integrating different modalities into a single model could enable more comprehensive understanding and generation of content. This opens up exciting possibilities for applications in areas like multimodal translation, video summarization, and content generation.
In conclusion, foundation models have had a profound impact on deep learning and have become indispensable tools for various tasks. As research progresses, we can anticipate advancements in efficiency, interpretability, and multimodal capabilities. These developments will further expand the range of applications for FMs and continue to push the boundaries of what is possible in the field of deep learning.
Read the original article