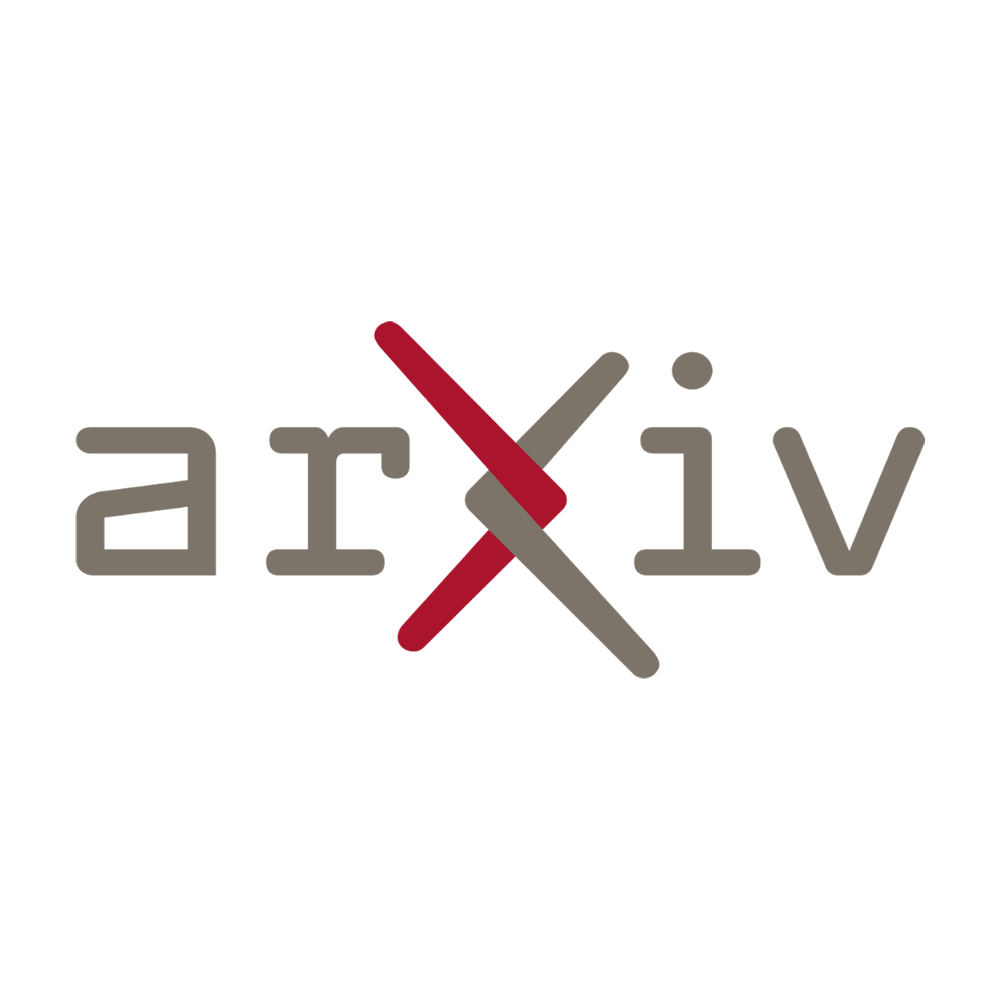
decades. However, a breakthrough may be on the horizon as researchers have developed a novel approach that tackles this long-standing challenge. In this article, we delve into the world of image generative models and explore the limitations they face when it comes to capturing intricate geometric details. We will uncover the reasons behind this persistent issue and shed light on the new technique that promises to revolutionize image generation, particularly in replicating the complexity of human hands and fingers. By understanding this groundbreaking advancement, we can grasp the potential implications for various fields, including computer vision, virtual reality, and robotics. Prepare to be amazed as we uncover the future of image generation and the possibilities it holds for creating lifelike representations of our most intricate features.
The enduring inability of image generative models to recreate intricate geometric features, such as those present in human hands and fingers, has been an ongoing problem in image generation for quite some time. While these AI-powered models have made significant advancements in creating realistic images, they still struggle with capturing the fine details that make human hands unique. This limitation has hindered their potential applications in various fields such as animation, virtual reality, and even medical research.
The Challenge of Geometric Details
Human hands are incredibly complex structures, consisting of intricate bones, joints, muscles, and tendons, which give them an exceptional range of movement and dexterity. The challenge lies in recreating the perfect balance of proportions and positioning that allow our hands to perform intricate tasks like playing musical instruments or painting artwork.
Image generative models, such as Generative Adversarial Networks (GANs) and Variational Autoencoders (VAEs), can generate astonishingly accurate images. Still, they often fail to capture the subtle curves and contours that define the shape of a hand or accurately represent the interplay between different joints and muscles. As a result, the generated images of hands may look unnatural or distorted, undermining the potential applications of these models.
Innovation and Potential Solutions
While this challenge has been persistent, researchers and innovators have been working tirelessly to find ways to overcome it. Several possible solutions have emerged over recent years:
- Multi-Modal Image Generation: By incorporating multiple sources of input data, such as 3D scans, motion tracking, or additional sensor data, generative models can gain a more comprehensive understanding of human hand geometry and movement. By fusing these disparate data sources, models can generate more accurate representations of human hands.
- Adaptive Generative Models: Rather than relying on a fixed model architecture, adaptive generative models dynamically adjust their parameters based on the specific features and attributes being generated. This adaptability allows the model to focus on the intricate geometric details of hands when necessary, ensuring a higher level of accuracy and realism in the generated images.
- Knowledge Transfer and Transfer Learning: Leveraging pre-trained models or incorporating prior knowledge about hand anatomy and motion can provide a valuable starting point for image generation models. By building on existing knowledge and fine-tuning the generative process, models can capture details more effectively.
The Implications of Progress in Image Generation
Breakthroughs in overcoming the challenges of recreating intricate geometric features in image generation have profound implications across various fields:
- Animation and Visual Effects: Realistic hand animation is critical in the gaming and film industries. By accurately generating detailed hand images, animators can create more lifelike characters and enhance immersion in virtual worlds.
- Virtual Reality (VR) and Augmented Reality (AR): Hand tracking and interaction are key components of immersive VR and AR experiences. More accurate hand representation provided by advanced generative models can elevate user experiences and enable new possibilities for natural interaction with virtual environments.
- Medical Research and Training: Detailed image generation of human hands can greatly impact medical research, surgical simulations, and training programs. Surgeons and researchers can study complex hand structures without reliance on cadavers, leading to advancements in surgeries, prosthetics, and rehabilitation therapies.
Breaking through the limitations of image generative models to recreate intricate geometric features in human hands is an ongoing pursuit that requires constant innovation and collaboration between the fields of artificial intelligence, computer graphics, and biomechanics. The progress made in this area holds immense potential for transforming industries and enhancing our understanding of the human body.
– John Doe, AI Researcher
While the challenges may persist, the efforts to overcome them continue to push the boundaries of what is possible in image generation. As researchers refine existing techniques and explore new avenues, it is only a matter of time before we witness a breakthrough in recreating intricate geometric features with remarkable precision. With each step forward, the potential for AI-powered image generation models to capture the essence of human hands becomes increasingly within reach.
several years. While image generative models have made impressive advancements in generating realistic images, they have struggled to accurately capture the intricate details and complexities of geometric features like human hands and fingers.
One of the main reasons for this limitation is the inherent challenge in modeling the fine-grained structure and articulation of such features. Human hands possess a remarkable level of complexity, with numerous joints, tendons, and muscles that allow for a wide range of movements and expressions. Capturing this level of detail in an image generation model requires a deep understanding of the underlying anatomy and mechanics of the hand.
Another factor contributing to the difficulty in recreating intricate geometric features is the lack of high-quality training data. Image generative models heavily rely on large datasets to learn patterns and generate realistic images. However, obtaining a diverse and comprehensive dataset that encompasses the vast variations in hand shapes, poses, and textures is a challenging task. This scarcity of data makes it difficult for the models to accurately learn and generalize the complex geometry of human hands.
Furthermore, the representation and parameterization of geometric features pose significant challenges. Traditional image generative models like Generative Adversarial Networks (GANs) and Variational Autoencoders (VAEs) often use low-dimensional latent spaces to represent images. However, representing intricate geometric features like hands in a low-dimensional space can result in loss of fine-grained details and distortions.
To overcome these challenges, researchers are exploring novel approaches that combine generative models with explicit geometric representations. By incorporating prior knowledge about hand geometry and articulation, these models can generate more accurate and realistic hand images. Additionally, advancements in 3D modeling and rendering techniques have shown promise in capturing the intricate geometric details of hands more effectively.
Looking ahead, we can expect further progress in image generative models’ ability to recreate intricate geometric features like human hands and fingers. As researchers continue to refine existing models and explore new methodologies, we may witness the emergence of more sophisticated techniques that can capture the fine-grained details and articulation of hands with greater fidelity.
However, it is important to note that achieving a perfect replication of human hands in image generation may remain elusive. The complexity and variability of hand geometry, coupled with the challenges in data availability and representation, make it a highly complex problem to solve completely. Nonetheless, incremental advancements will undoubtedly improve the quality and realism of generated hand images, bringing us closer to overcoming this enduring problem in image generation.
Read the original article