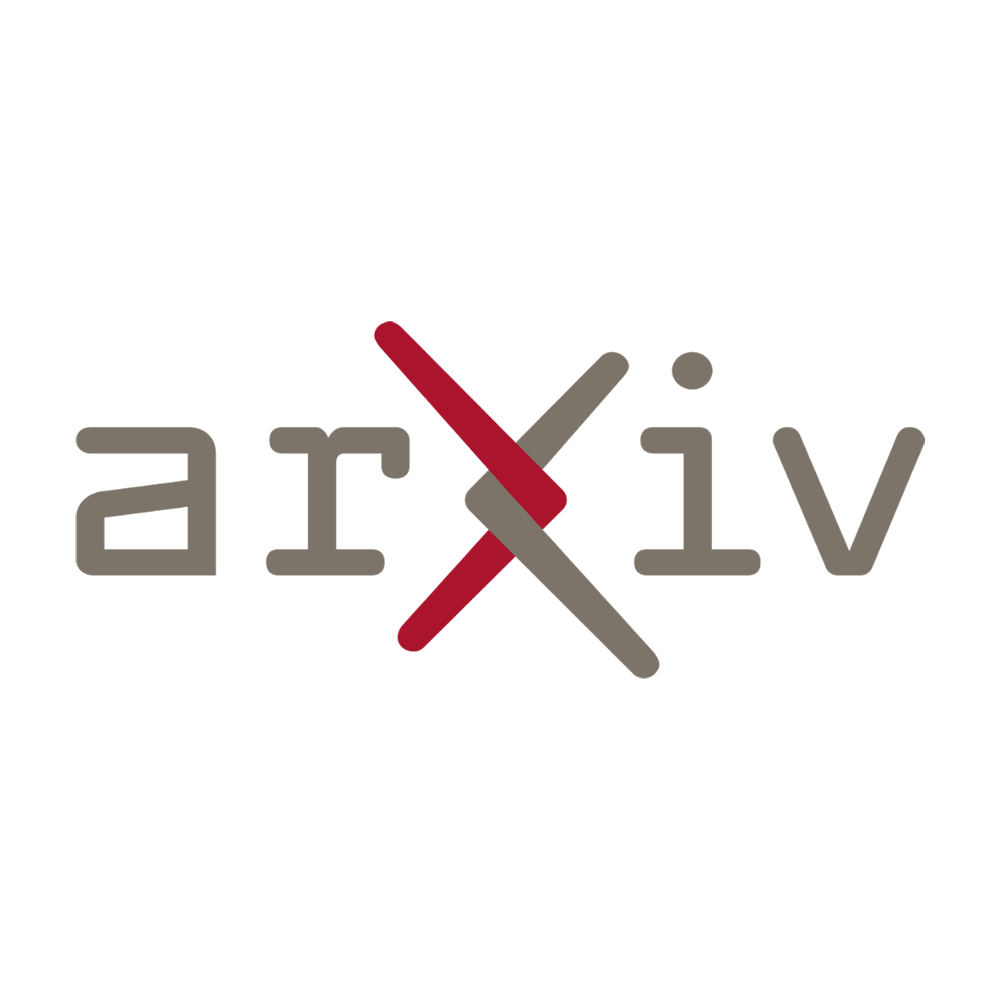
In today’s rapidly evolving field of image generation, the ability to tackle diverse tasks and generalize across unseen ones is crucial. This article introduces instruct-imagen, a groundbreaking model that addresses heterogeneous image generation tasks while also demonstrating remarkable generalization capabilities. By incorporating a novel concept called multi-modal instruction, this model pushes the boundaries of image generation by seamlessly synthesizing instructions from various sources to produce highly accurate and visually appealing images. Join us as we delve into the intricacies of instruct-imagen and explore its potential to revolutionize the world of image generation.
Reimagining the Possibilities of Heterogeneous Image Generation with Instruct-Imagen
This paper introduces a groundbreaking model called instruct-imagen, which opens new gates in the world of heterogeneous image generation. With the ability to generalize across unseen tasks, instruct-imagen brings the concept of *multi-modal instruction* to the forefront of image generation.
Unleashing the Power of Multi-Modal Instruction
Instruct-imagen takes traditional image generation to new heights by incorporating multi-modal instruction. This means that it can process not only textual instructions but also diverse forms of input like sketches, diagrams, or even audio commands. By leveraging the full spectrum of communication modalities, this model allows users to express their vision in even more explicit and intuitive ways.
The potential applications of this approach are vast. Imagine a scenario where an artist can provide a rough sketch of their desired image, along with a written description of specific details and colors. Instruct-imagen would then interpret and merge these instructions to generate a stunning digital artwork that perfectly matches the artist’s creative intent.
Generalization: The Key to Tackling Unseen Tasks
One remarkable feature of instruct-imagen is its ability to generalize across unseen tasks. This means that the model can be trained on a set of diverse image generation tasks and still perform exceptionally well on entirely new and different tasks it has never encountered before.
The implications of this generalization ability are profound. By training instruct-imagen on a wide variety of image generation tasks, such as landscape painting, object reconstruction, or portrait designing, we can create a versatile and adaptable model that can handle a multitude of creative projects. Artists and designers will no longer have to rely on specialized models for different tasks, as instruct-imagen can seamlessly transition between various creative domains.
Proposing Innovative Solutions: Democratizing Creative Tools
Instruct-imagen brings an opportunity to democratize creative tools in a revolutionary way. By making image generation accessible to a broader range of users, this model empowers individuals who may not have extensive artistic skills or technical expertise but possess unique visions and ideas. Whether they are architects, fashion designers, or hobbyists, instruct-imagen allows them to express their creativity without being limited by traditional barriers.
“Instruct-imagen has the potential to redefine the landscape of digital art and design, enabling anyone with a creative spark to materialize their imagination.”
In addition to democratizing creative tools, instruct-imagen also has immense commercial applications. From advertisement agencies seeking quick image mock-ups to interior designers envisioning virtual room transformations, this model offers an efficient and flexible solution for various industries that heavily rely on image generation and manipulation.
Conclusion
Instruct-imagen shines a new light on the landscape of heterogeneous image generation. By incorporating multi-modal instruction and showcasing impressive generalization abilities, this model revolutionizes how we generate and manipulate images. Its potential applications include empowering individuals with diverse creative visions and streamlining workflows in countless industries.
In a world where creativity knows no boundaries, instruct-imagen opens up exciting possibilities. With this model leading the way, we can look forward to a future where the realm of art and design is enriched by the fusion of human imagination and cutting-edge technology.
technique that combines textual instructions with visual prompts to generate diverse and contextually relevant images.
The concept of instruct-imagen is highly intriguing and has the potential to revolutionize the field of image generation. Traditional image generation models often struggle to generate images that align with specific instructions, particularly when dealing with complex and diverse tasks. Instruct-imagen aims to address this limitation by incorporating multi-modal instructions, which provide a more comprehensive and nuanced understanding of the desired image.
One key aspect of instruct-imagen is the integration of textual instructions with visual prompts. By combining these two modalities, the model can leverage both the semantic information conveyed through text and the visual cues provided by the prompts. This dual input approach allows for a more robust and accurate understanding of the desired image, enabling the model to generate images that better align with the given instructions.
The ability of instruct-imagen to generalize across unseen tasks is a significant advancement. Many existing image generation models are task-specific, meaning they are trained on a particular set of instructions and struggle to generate images for unseen tasks. Instruct-imagen, on the other hand, demonstrates the potential to learn a more abstract representation of instructions that can be applied to a wide range of tasks. This generalization capability is critical in real-world scenarios where new tasks constantly emerge, allowing the model to adapt and generate images for previously unseen instructions.
Furthermore, instruct-imagen’s emphasis on generating diverse images is a valuable contribution to the field. Diversity is often desirable in image generation, as it allows for a broader range of creative outputs. By incorporating techniques that encourage diversity in generated images, instruct-imagen opens up possibilities for applications such as art, design, and creative content generation.
Looking ahead, there are several avenues for further exploration and improvement. One potential direction is to investigate the interpretability of instruct-imagen. Understanding how the model processes and synthesizes textual instructions and visual prompts can provide insights into its decision-making process and potentially lead to improvements. Additionally, refining the model’s ability to handle ambiguous or contradictory instructions could enhance its robustness in real-world scenarios.
Another area of interest is exploring the scalability of instruct-imagen. While the paper demonstrates promising results, it would be valuable to investigate how the model performs on larger and more diverse datasets. Scaling up the model’s training and testing processes could reveal its true potential and uncover any limitations or challenges that may arise.
In conclusion, instruct-imagen presents a compelling approach to heterogeneous image generation tasks by incorporating multi-modal instructions. Its ability to generalize across unseen tasks, generate diverse images, and combine textual instructions with visual prompts makes it a significant contribution to the field. With further research and refinement, instruct-imagen has the potential to advance image generation capabilities and find applications in various domains.
Read the original article