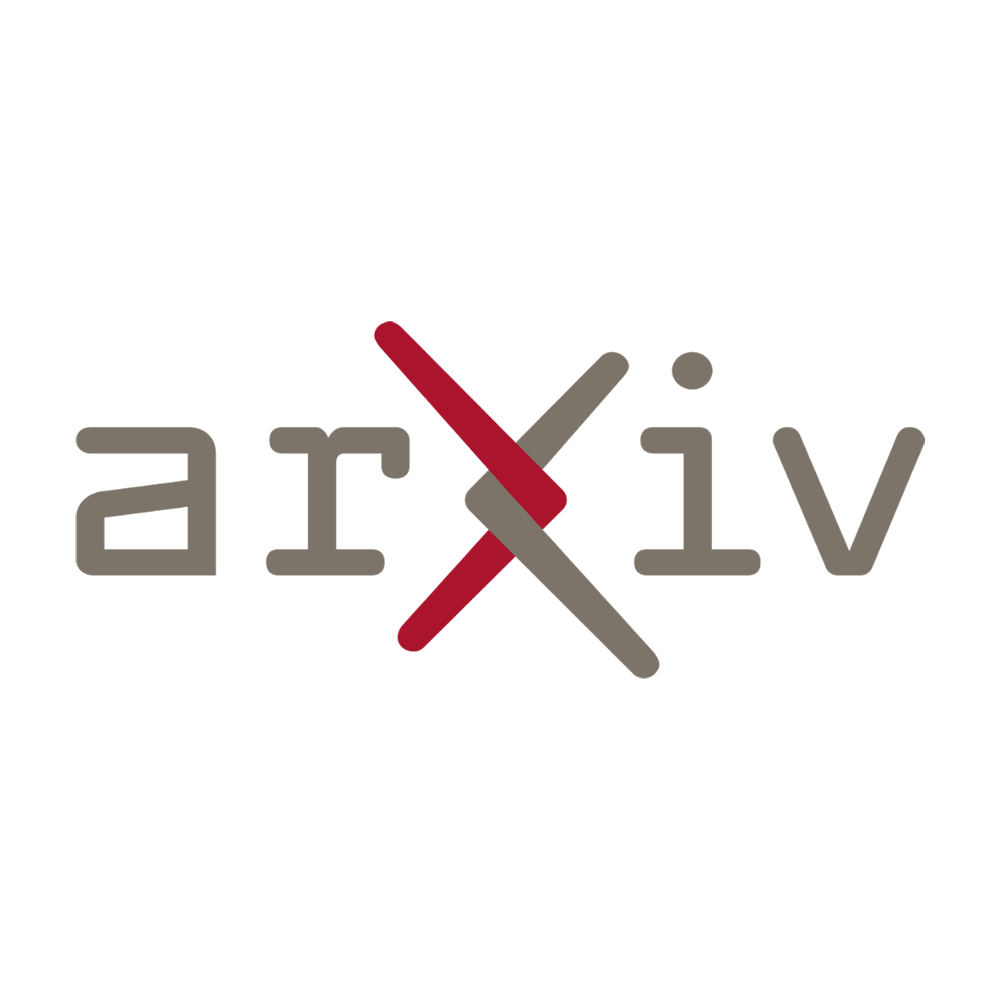
that visual anomaly detection algorithms are becoming highly accurate and reliable. These advancements have been achieved through the use of deep learning techniques, which have revolutionized the field of computer vision. By training neural networks on large-scale datasets, researchers have been able to develop models that can accurately detect anomalies in images and videos with unprecedented precision. This article explores the recent progress in visual anomaly detection research, highlighting the improvements in AUROC and AUPRO scores on benchmark datasets. It also discusses the implications of these advancements, including their potential applications in various industries such as manufacturing, surveillance, and healthcare.
Recent advances in visual anomaly detection research have seen AUROC and AUPRO scores on public benchmark datasets such as MVTec and VisA converge towards perfect recall, giving the impression that we are nearing the end of the road in terms of improving anomaly detection performance. However, the underlying themes and concepts behind these advancements raise important questions about the limitations of current approaches and the need for innovative solutions.
The Problem of Labeling Anomalies
One of the fundamental challenges in visual anomaly detection lies in accurately labeling anomalies. In most benchmark datasets, anomalies are pre-defined and annotated. This approach assumes a static definition of anomalies, which may not hold in real-world scenarios where anomalies can be dynamic and ever-evolving. Additionally, relying on human-labeled annotations introduces subjectivity and potential inconsistencies.
To address this problem, researchers should focus on developing algorithms that can learn from unlabeled data. Unsupervised anomaly detection approaches can provide a more flexible and robust solution by allowing the model to identify anomalies without relying on predefined labels. This could involve leveraging techniques such as self-supervised learning or generative models to learn representations that capture the underlying structure of normal data, enabling the detection of deviations.
The Challenge of Real-World Anomalies
While current benchmark datasets provide a valuable testing ground for anomaly detection algorithms, they often fail to capture the true complexity of real-world anomalies. These datasets typically focus on specific object categories or controlled scenarios, which may not adequately reflect the wide range of anomalies encountered in practice.
To overcome this limitation, it is crucial to collect and annotate diverse real-world datasets that cover a broad spectrum of anomalies. Leveraging crowdsourcing platforms or collaboration with industry partners can help gather data from various domains, ensuring that anomaly detection algorithms are robust and applicable across different contexts. Additionally, incorporating natural variations and noise into these datasets can further challenge algorithms and foster the development of more robust models.
Contextual Anomaly Detection
Anomaly detection approaches often assume that anomalies can be detected solely based on visual information. However, anomalies are inherently context-dependent, and their detection may require considering additional information beyond pixels. Contextual anomalies refer to situations where an object or event may not be anomalous in isolation but becomes anomalous in a specific context.
To address contextual anomalies, researchers should explore multi-modal approaches that integrate visual data with contextual information such as textual data, temporal data, or environmental factors. By incorporating these additional dimensions, algorithms can gain a deeper understanding of the scene and make more informed anomaly predictions. For example, a car parked in the middle of a highway may not seem anomalous in a rural area but would be considered an anomaly in an urban setting.
Continual Learning for Anomaly Detection
As anomalies continue to evolve and adapt, anomaly detection models should be able to continuously update their knowledge to remain effective over time. Traditional approaches often assume static datasets and fail to adapt to new anomalies or changing environments.
To enable continual learning in anomaly detection, researchers should explore techniques such as online learning, transfer learning, and active learning. Online learning allows models to update their parameters using new data streams, ensuring that they stay up-to-date with emerging anomalies. Transfer learning can leverage knowledge gained from previously seen anomalies to detect new ones. Active learning involves incorporating human feedback to guide the model’s learning process, enabling it to focus on uncertain or challenging instances.
Conclusion: While recent advances in visual anomaly detection have showcased impressive performance on benchmark datasets, it is important to critically examine the underlying themes and concepts driving these advancements. By addressing the challenges of labeling anomalies, accounting for real-world complexities, considering contextual information, and enabling continual learning, researchers can push the boundaries of anomaly detection and provide more robust and adaptable solutions. This will ultimately enhance the practicality and reliability of anomaly detection systems, opening up opportunities for numerous applications in areas such as security, manufacturing, and healthcare.
that visual anomaly detection algorithms are becoming highly accurate in detecting anomalies. This is a significant development in the field, as accurate anomaly detection plays a crucial role in many real-world applications, such as surveillance, quality control, and medical diagnosis.
The AUROC (Area Under the Receiver Operating Characteristic curve) and AUPRO (Area Under the Precision-Recall curve) are commonly used evaluation metrics in anomaly detection research. These scores provide insights into the performance of algorithms by measuring their ability to discriminate between normal and anomalous samples. As these scores approach perfect recall, it suggests that the algorithms are becoming more effective at correctly identifying anomalies while minimizing false negatives.
One of the reasons for this progress is the availability of large-scale benchmark datasets like MVTec and VisA. These datasets provide researchers with a standardized evaluation platform, allowing for fair comparisons between different algorithms. The convergence towards perfect recall on these datasets reflects the collective efforts of researchers to develop more sophisticated and robust anomaly detection models.
However, it is important to note that achieving perfect recall does not necessarily mean that visual anomaly detection algorithms are flawless. While they may have high sensitivity in detecting anomalies, there could still be room for improvement in terms of specificity and generalization to real-world scenarios. Algorithms need to strike a balance between minimizing false negatives (anomalies missed) and false positives (normal samples falsely classified as anomalies).
To further advance the field, future research could focus on addressing some of the challenges that still exist in visual anomaly detection. One such challenge is the presence of complex and rare anomalies that may not be well-represented in existing benchmark datasets. Developing techniques to handle such cases and improve generalization capabilities would be valuable.
Additionally, incorporating domain knowledge and contextual information into anomaly detection algorithms could enhance their performance. By considering factors such as the environment, temporal dynamics, and interdependencies between different data sources, algorithms could become more robust and accurate in detecting anomalies in real-world settings.
Furthermore, the interpretability of anomaly detection models is an area that deserves attention. While achieving high accuracy is essential, understanding the reasoning behind the model’s predictions is crucial for building trust and facilitating decision-making. Developing techniques to explain the detection process and provide interpretable insights could greatly enhance the usability and acceptance of visual anomaly detection systems.
In conclusion, recent advances in visual anomaly detection research have shown promising results, with AUROC and AUPRO scores converging towards perfect recall on benchmark datasets. This progress signifies the growing accuracy of anomaly detection algorithms, but there is still room for improvement in terms of specificity, generalization, and interpretability. Future research should focus on addressing these challenges to make visual anomaly detection algorithms more robust, reliable, and applicable in various real-world scenarios.
Read the original article