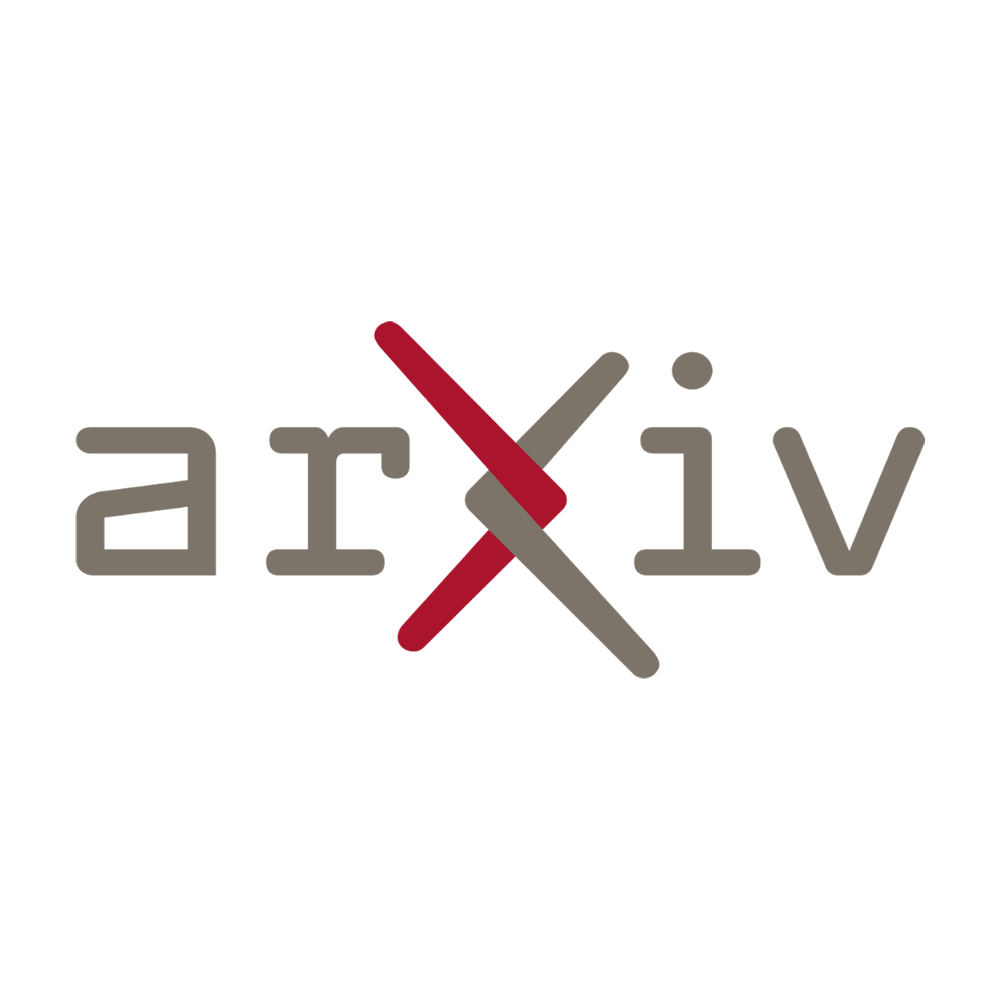
Multimodal alignment between language and vision is at the forefront of vision-language model research, and one representative method, Contrastive Captioners (CoCa), has emerged as a key player in this field. CoCa seamlessly integrates various modalities to enhance the understanding of images and their corresponding captions. By leveraging the power of both language and vision, this approach aims to bridge the gap between textual descriptions and visual content, paving the way for more accurate and comprehensive vision-language models. In this article, we will delve into the core themes of CoCa and explore how it revolutionizes the way we perceive and comprehend multimodal information.
Multimodal alignment between language and vision has emerged as a pivotal topic in vision-language model research. In this context, Contrastive Captioners (CoCa) stands out as a representative and innovative method that effectively integrates both modalities to enhance the understanding of visual content.
The Power of Multimodal Alignment
The idea behind multimodal alignment is to bridge the gap between the textual and visual representations of information. By aligning the two modalities, researchers aim to enhance the accuracy and effectiveness of vision-language models in tasks such as image captioning, visual question answering, and image recognition.
Through the alignment of language and vision, models can generate more accurate and semantically meaningful captions for images, improve image recognition capabilities, and even answer queries related to the visual content effectively.
Understanding Contrastive Captioners (CoCa)
CoCa is an emerging approach in vision-language modeling that focuses on optimizing the representation quality of both visual and textual modalities. It accomplishes this by contrasting positive image-caption pairs with negative pairs.
Positive pairs consist of an image-caption combination that accurately depicts the visual content. In contrast, negative pairs may feature a different caption for the same image or a different image altogether. By training on such contrasting pairs, CoCa models learn to better align the language and vision modalities by discriminating between correct and incorrect image-caption relationships.
Benefits and Applications
The use of CoCa models can unlock various benefits and possibilities in the intersection of language and vision. Some innovative applications include:
- Enhanced Image Captioning: CoCa can enable more accurate and contextually relevant captions for images by effectively aligning the visual and linguistic representations.
- Improved Visual Question Answering: By understanding the nuances of image-caption relationships, CoCa models can better comprehend and answer questions related to visual content, leading to enhanced performance in visual question answering tasks.
- Advanced Image Recognition: CoCa models, with their improved multimodal alignment, hold the potential to boost image recognition capabilities, aiding in various computer vision tasks and applications.
Future Directions
Building upon the concept of multimodal alignment and the innovative approach of CoCa, there are several promising directions for future research:
- Fine-Grained Image Captioning: Exploring techniques to capture and incorporate fine-grained details in image captions, such as specific object attributes or relationships, can lead to more descriptive and comprehensive image understanding.
- Multi-Modal Reasoning: Investigating methods to integrate logical and deductive reasoning within vision-language models can open doors to advanced capabilities, enabling more complex decision-making processes based on both visual and linguistic information.
- Interactive Visual Content Understanding: Exploring how CoCa models can be utilized in interactive scenarios, such as interactive image captioning or visual storytelling, can pave the way for novel applications where users can actively participate in the generation and manipulation of multimodal content.
The integration of language and vision holds immense potential for advancing various fields, including artificial intelligence, computer vision, and natural language processing. By harnessing the power of multimodal alignment and delving into innovative approaches like CoCa models, we can unlock new frontiers in visual understanding, semantic comprehension, and interactive content generation.
advanced techniques from both computer vision and natural language processing to achieve multimodal alignment between language and vision.
The main goal of multimodal alignment is to enable machines to understand and generate natural language descriptions of visual content accurately. This is a challenging task as it requires bridging the gap between two different modalities, language and vision, which have inherently different structures and representations.
Contrastive Captioners (CoCa) approach this problem by leveraging contrastive learning techniques. Contrastive learning aims to learn representations by contrasting positive pairs (similar samples) against negative pairs (dissimilar samples). In the context of CoCa, positive pairs consist of an image and its corresponding caption, while negative pairs consist of mismatched image-caption pairs.
By training on positive and negative pairs, CoCa learns to align the visual and textual representations in a shared embedding space. This alignment allows the model to associate relevant visual features with their corresponding textual descriptions accurately. Through this process, CoCa captures the semantic relationship between images and captions, enabling it to generate captions that accurately describe the content of an image.
CoCa has shown promising results in various vision-language tasks, such as image captioning, visual question answering, and image-text retrieval. By leveraging contrastive learning, CoCa can effectively exploit the rich information present in both images and captions to improve the quality of generated captions or retrieve relevant images based on textual queries.
In terms of future developments, we can expect further advancements in multimodal alignment techniques. Researchers will likely explore more advanced contrastive learning strategies, such as incorporating additional modalities like sound or touch, to enhance the alignment between different sensory inputs.
Furthermore, there is a growing interest in making vision-language models more interpretable and explainable. While CoCa and similar methods have achieved impressive performance, understanding the underlying reasoning and decision-making process of these models remains a challenge. Future research may focus on developing techniques that provide insights into how the model aligns visual and textual information, leading to more transparent and interpretable vision-language models.
Overall, multimodal alignment between language and vision, as exemplified by methods like CoCa, holds great potential for advancing the fields of computer vision and natural language processing. By effectively integrating information from both modalities, these models can facilitate a deeper understanding of visual content and enable more sophisticated interactions between humans and machines.
Read the original article